Enhanced global optimization methods applied to complex fisheries stock assessment models.
Applied Soft Computing(2019)
摘要
Statistical fisheries models are frequently used by researchers and agencies to understand the behavior of marine ecosystems or to estimate the maximum acceptable catch of different species of commercial interest. The parameters of these models are usually adjusted through the use of optimization algorithms. Unfortunately, the choice of the best optimization method is far from trivial. This work proposes the use of population-based algorithms to improve the optimization process of the Globally applicable Area Disaggregated General Ecosystem Toolbox (Gadget), a flexible framework that allows the development of complex statistical marine ecosystem models. Specifically, parallel versions of the Differential Evolution (DE) and the Particle Swarm Optimization (PSO) methods are proposed. The proposals include an automatic selection of the internal parameters to reduce the complexity of their usage, and a restart mechanism to avoid local minima. The resulting optimization algorithms were called PMA (Parallel Multirestart Adaptive) DE and PMA PSO respectively. Experimental results prove that the new algorithms are faster and produce more accurate solutions than the other parallel optimization methods already included in Gadget. Although the new proposals have been evaluated on fisheries models, there is nothing specific to the tested models in them, and thus they can be also applied to other optimization problems. Moreover, the PMA scheme proposed can be seen as a template that can be easily applied to other population-based heuristics.
更多查看译文
关键词
Global optimization,Parallel programming,Marine ecosystem models,Particle Swarm Optimization,Differential evolution
AI 理解论文
溯源树
样例
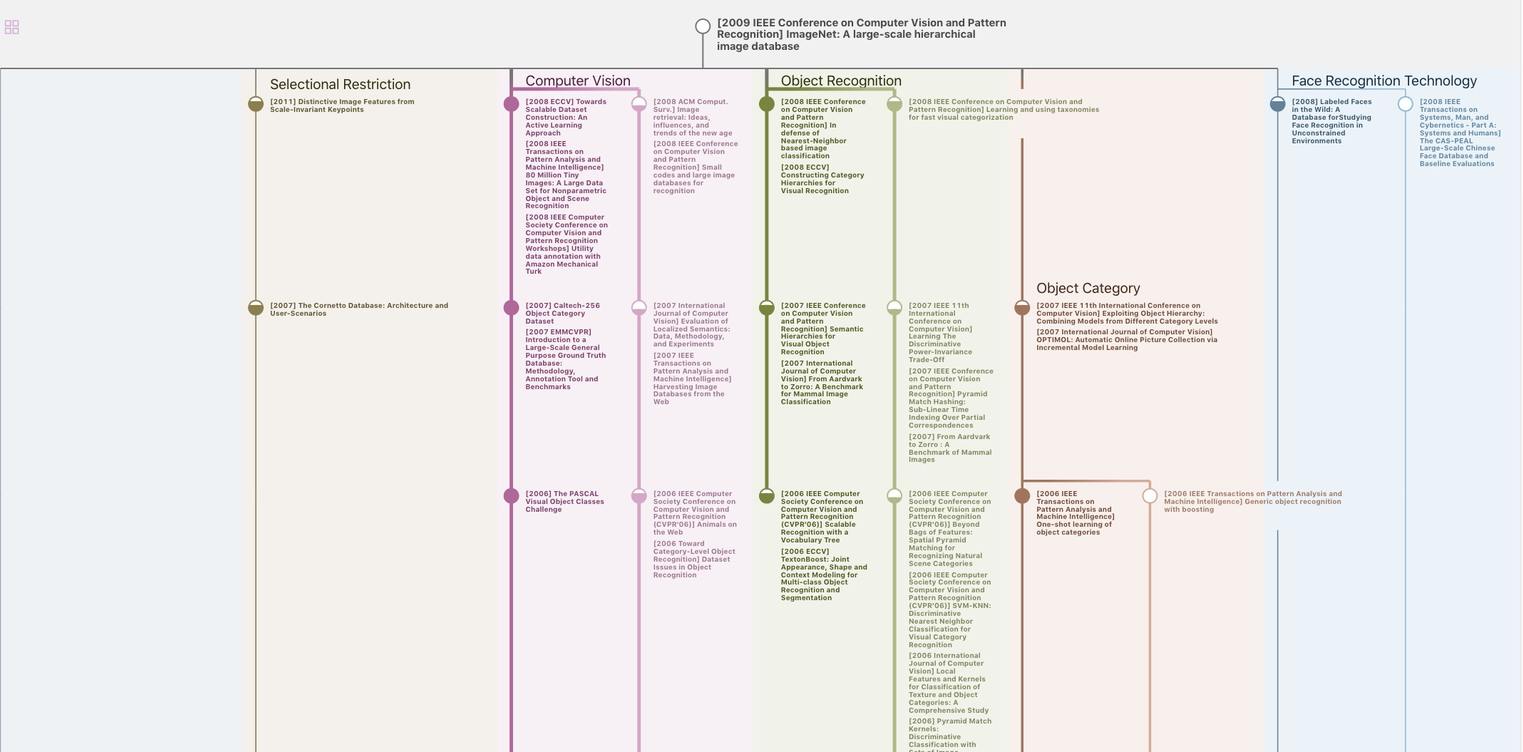
生成溯源树,研究论文发展脉络
Chat Paper
正在生成论文摘要