Bidirectional LSTM with Extended Input Context
2018 11th International Symposium on Chinese Spoken Language Processing (ISCSLP)(2018)
摘要
Long short-term memory (LSTM) unit has been widely used in speech recognition tasks, both for acoustic model and language model. For offline speech recognition task, bidirectional LSTM (BLSTM) is the state-of-the-art acoustic model. In this paper, we propose the BLSTM with extended input context (BLSTM-E), which achieves higher speech recognition accuracy than the standard BLSTM. Time delay neural network (TDNN) or element-wise scale block-sum network (ESBN) is used to extend the input context of forward and backward LSTM. Our experiments show that the proposed ESBN-BLSTM-E can achieve 0.9% absolute reduction in word error rate (WER) trained on one 1000 hours Chinese conversational telephone speech (CTS) compared with the standard BLSTM. Meanwhile, compared with the standard BLSTM, ESBN-BLSTM-E reduces relative 22.1% model parameter size.
更多查看译文
关键词
Standards,Speech recognition,Acoustics,Logic gates,Context modeling,Neural networks,Computer architecture
AI 理解论文
溯源树
样例
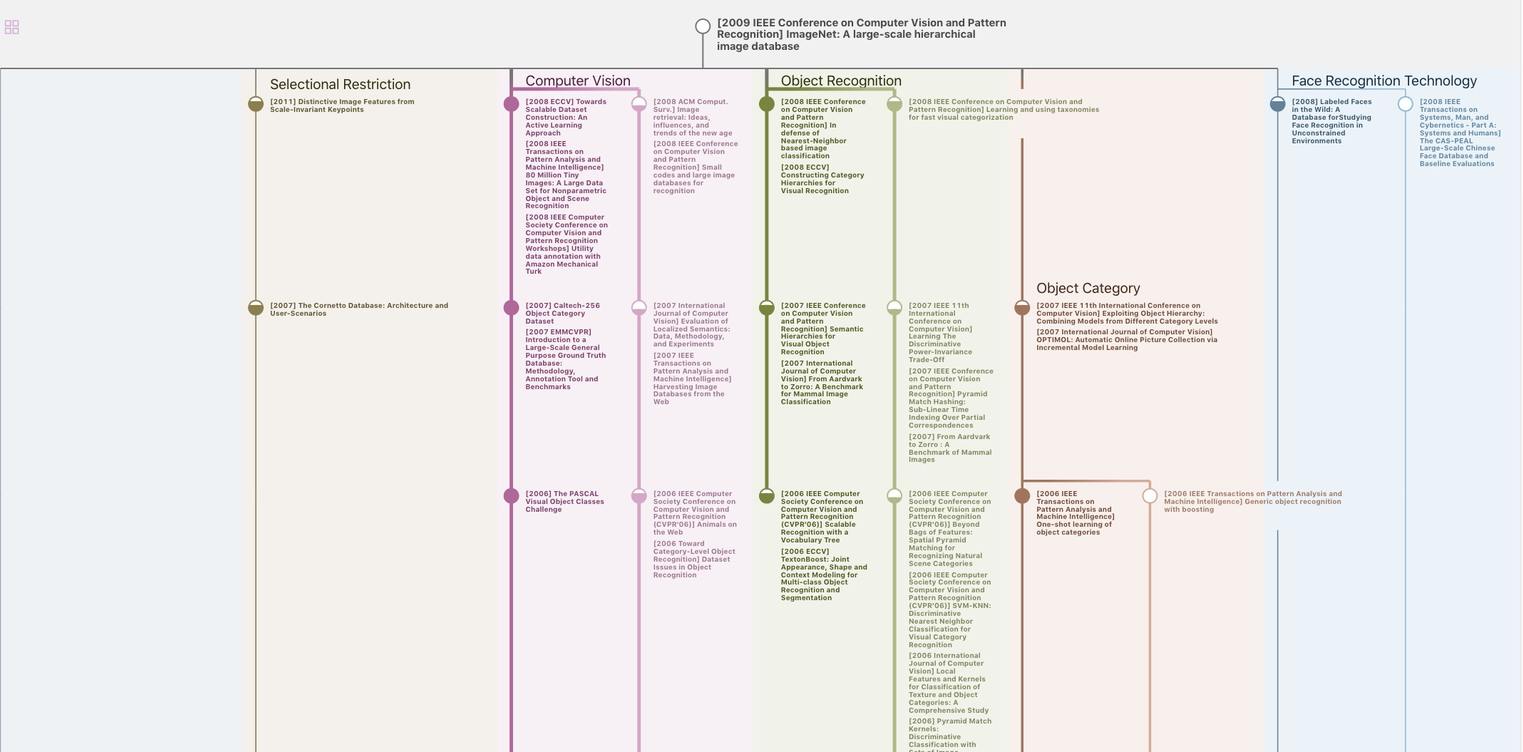
生成溯源树,研究论文发展脉络
Chat Paper
正在生成论文摘要