Development of Predictive Models in Patients with Epiphora Using Lacrimal Scintigraphy and Machine Learning
Nuclear Medicine and Molecular Imaging(2019)
摘要
Purpose We developed predictive models using different programming languages and different computing platforms for machine learning (ML) and deep learning (DL) that classify clinical diagnoses in patients with epiphora. We evaluated the diagnostic performance of these models. Methods Between January 2016 and September 2017, 250 patients with epiphora who underwent dacryocystography (DCG) and lacrimal scintigraphy (LS) were included in the study. We developed five different predictive models using ML tools, Python-based TensorFlow, R, and Microsoft Azure Machine Learning Studio (MAMLS). A total of 27 clinical characteristics and parameters including variables related to epiphora (VE) and variables related to dacryocystography (VDCG) were used as input data. Apart from this, we developed two predictive convolutional neural network (CNN) models for diagnosing LS images. We conducted this study using supervised learning. Results Among 500 eyes of 250 patients, 59 eyes had anatomical obstruction, 338 eyes had functional obstruction, and the remaining 103 eyes were normal. For the data set that excluded VE and VDCG, the test accuracies in Python-based TensorFlow, R, multiclass logistic regression in MAMLS, multiclass neural network in MAMLS, and nuclear medicine physician were 81.70%, 80.60%, 81.70%, 73.10%, and 80.60%, respectively. The test accuracies of CNN models in three-class classification diagnosis and binary classification diagnosis were 72.00% and 77.42%, respectively. Conclusions ML-based predictive models using different programming languages and different computing platforms were useful for classifying clinical diagnoses in patients with epiphora and were similar to a clinician’s diagnostic ability.
更多查看译文
关键词
Epiphora,Dacryocystography,Lacrimal scintigraphy,Machine learning,Deep learning,Convolutional neural network
AI 理解论文
溯源树
样例
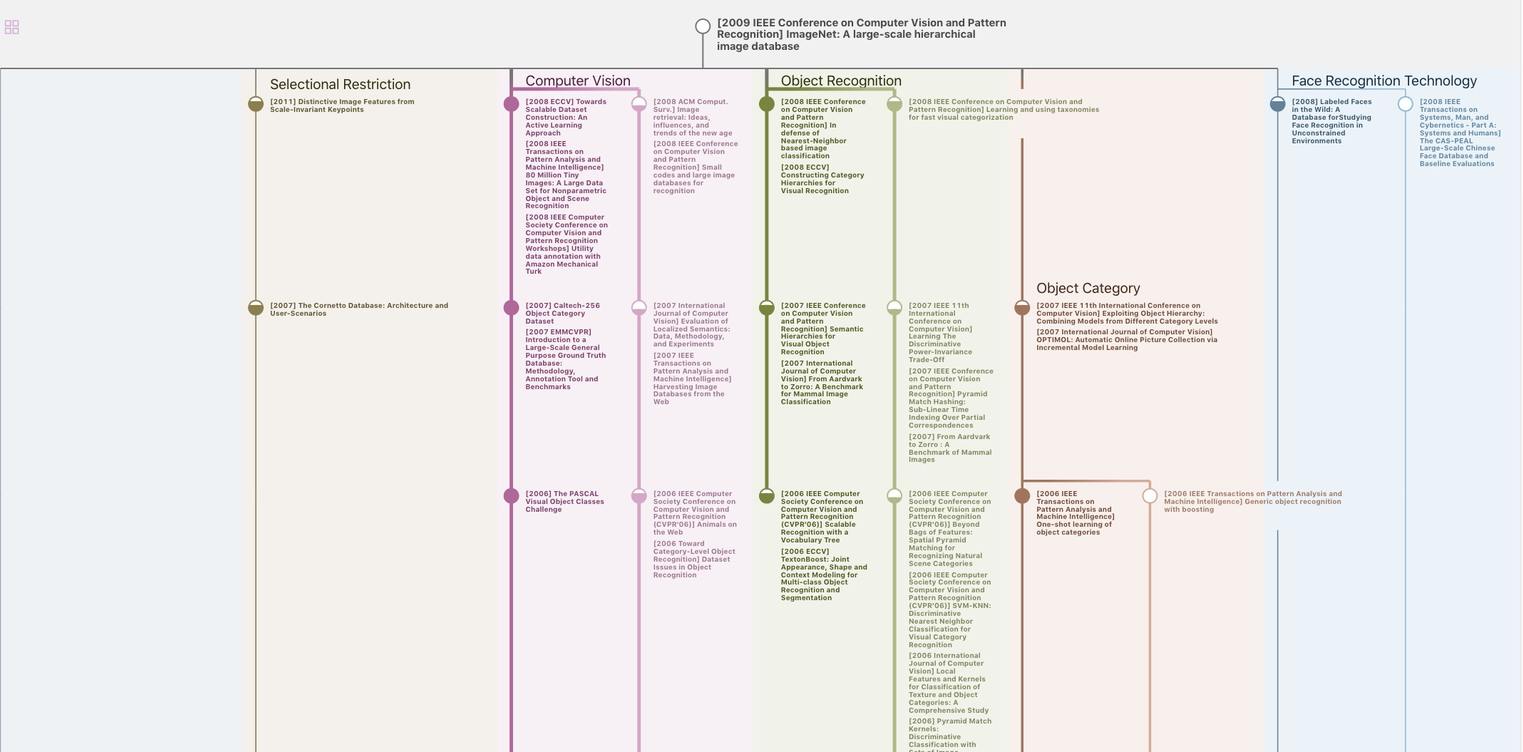
生成溯源树,研究论文发展脉络
Chat Paper
正在生成论文摘要