Analysis and Comparison of Vector Space and Metric Space Representations in QSAR Modeling.
MOLECULES(2019)
摘要
The performance of quantitative structure-activity relationship (QSAR) models largely depends on the relevance of the selected molecular representation used as input data matrices. This work presents a thorough comparative analysis of two main categories of molecular representations (vector space and metric space) for fitting robust machine learning models in QSAR problems. For the assessment of these methods, seven different molecular representations that included RDKit descriptors, five different fingerprints types (MACCS, PubChem, FP2-based, Atom Pair, and ECFP4), and a graph matching approach (non-contiguous atom matching structure similarity; NAMS) in both vector space and metric space, were subjected to state-of-art machine learning methods that included different dimensionality reduction methods (feature selection and linear dimensionality reduction). Five distinct QSAR data sets were used for direct assessment and analysis. Results show that, in general, metric-space and vector-space representations are able to produce equivalent models, but there are significant differences between individual approaches. The NAMS-based similarity approach consistently outperformed most fingerprint representations in model quality, closely followed by Atom Pair fingerprints. To further verify these findings, the metric space-based models were fitted to the same data sets with the closest neighbors removed. These latter results further strengthened the above conclusions. The metric space graph-based approach appeared significantly superior to the other representations, albeit at a significant computational cost.
更多查看译文
关键词
QSAR modeling,non-contiguous atom matching structure similarityNAMS,metric space,vector space,PCA,feature selection,random forest,support vector machines
AI 理解论文
溯源树
样例
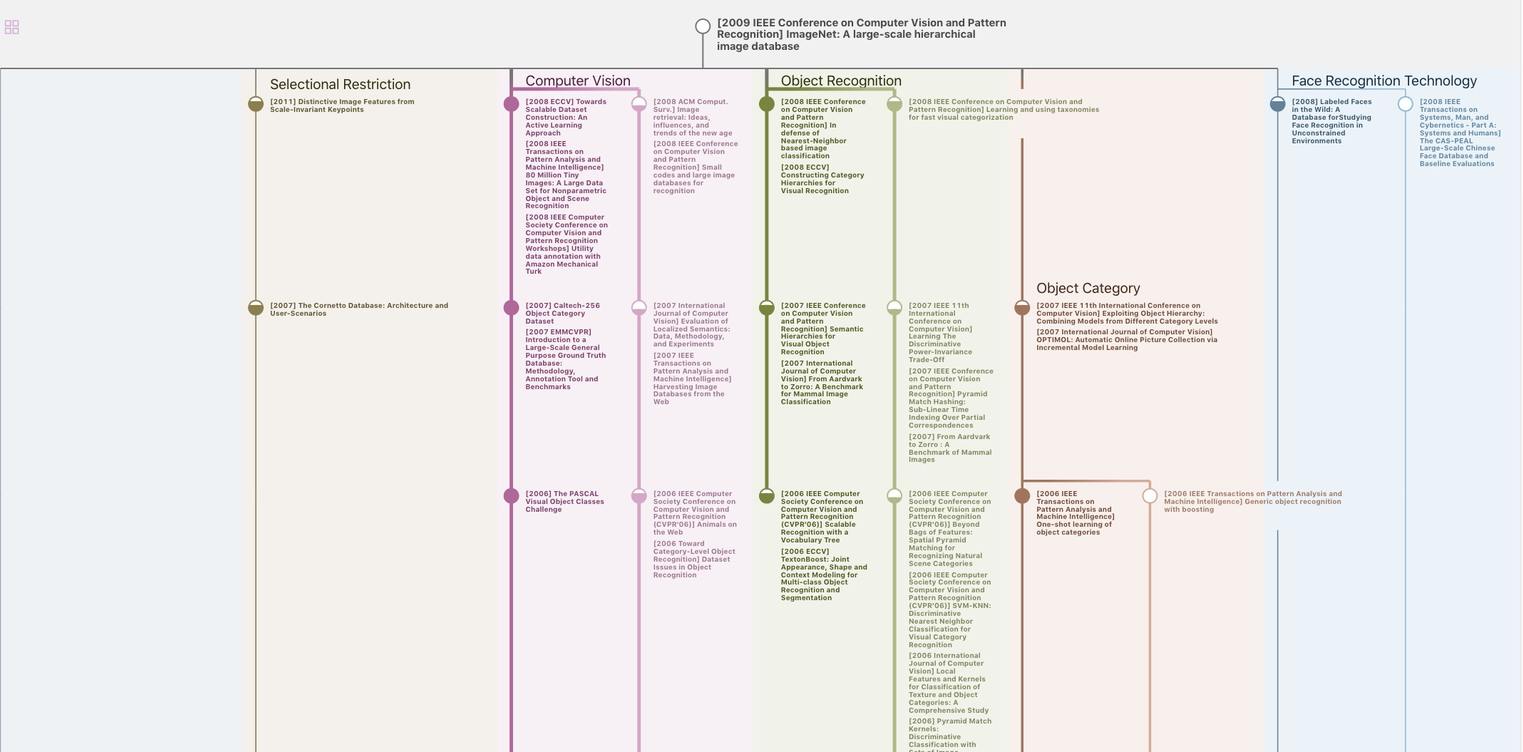
生成溯源树,研究论文发展脉络
Chat Paper
正在生成论文摘要