Combining gene expression data and prior knowledge for inferring gene regulatory networks via Bayesian networks using structural restrictions.
STATISTICAL APPLICATIONS IN GENETICS AND MOLECULAR BIOLOGY(2019)
摘要
Gene Regulatory Networks (GRNs) are known as the most adequate instrument to provide a clear insight and understanding of the cellular systems. One of the most successful techniques to reconstruct GRNs using gene expression data is Bayesian networks (BN) which have proven to be an ideal approach for heterogeneous data integration in the learning process. Nevertheless, the incorporation of prior knowledge has been achieved by using prior beliefs or by using networks as a starting point in the search process. In this work, the utilization of different kinds of structural restrictions within algorithms for learning BNs from gene expression data is considered. These restrictions will codify prior knowledge, in such a way that a BN should satisfy them. Therefore, one aim of this work is to make a detailed review on the use of prior knowledge and gene expression data to inferring GRNs from BNs, but the major purpose in this paper is to research whether the structural learning algorithms for BNs from expression data can achieve better outcomes exploiting this prior knowledge with the use of structural restrictions. In the experimental study, it is shown that this new way to incorporate prior knowledge leads us to achieve better reverse-engineered networks.
更多查看译文
关键词
Bayesian networks,genetic regulatory networks,microarray data,prior knowledge,structural restrictions
AI 理解论文
溯源树
样例
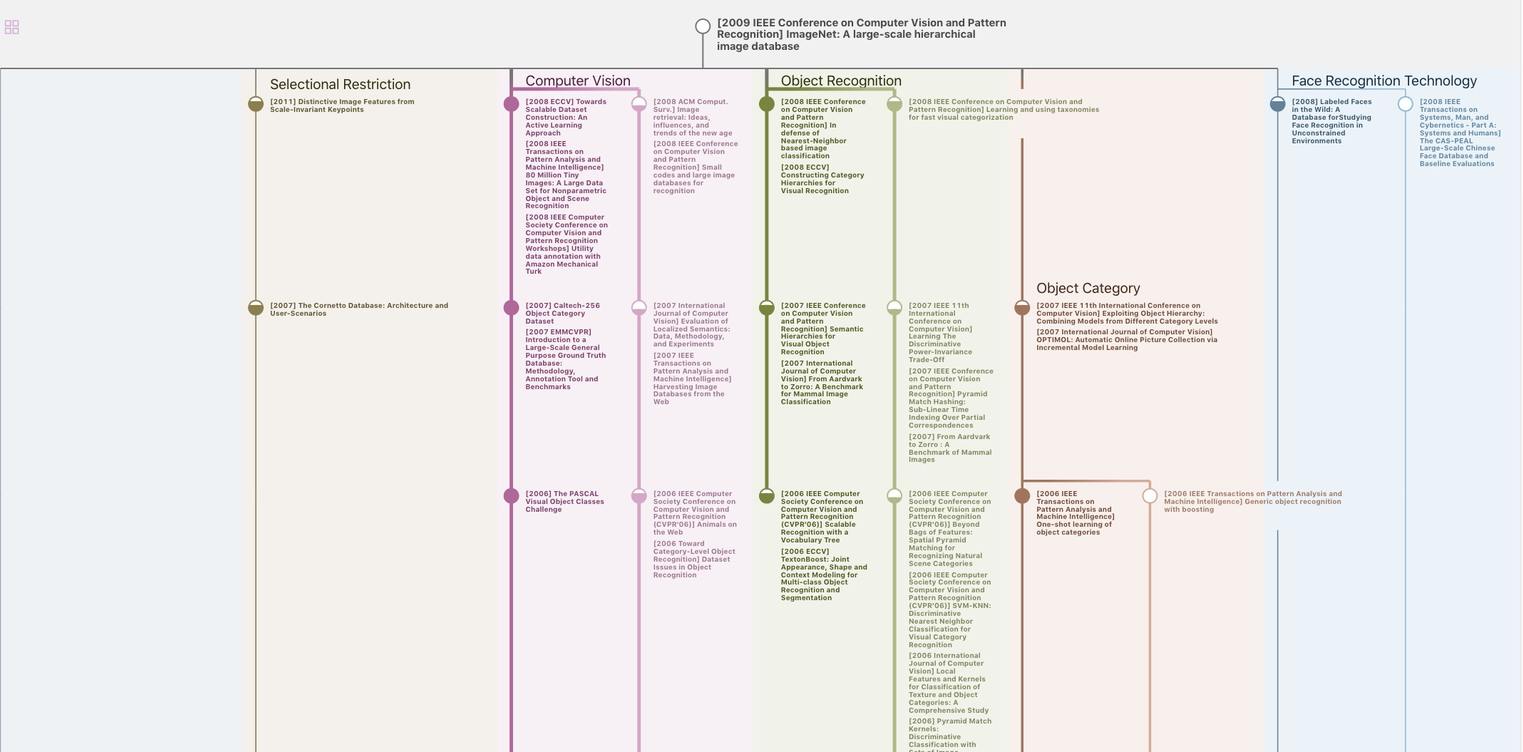
生成溯源树,研究论文发展脉络
Chat Paper
正在生成论文摘要