DMDP: A Dynamic Multi-source Default Probability Prediction Framework
Data Science and Engineering(2019)
摘要
In this paper, we propose a dynamic forecasting framework, named DMDP (dynamic multi-source default probability prediction), to predict the default probability of a company. The default probability is a very important factor to assess the credit risk of listed companies on a stock market. Aiming at aiding financial institutions in decision making, our DMDP framework not only analyzes financial data to capture the historical performance of a company, but also utilizes long short-term memory model to dynamically incorporate daily news from social media to take the perceptions of market participants and public opinions into consideration. The study of this paper makes two key contributions. First, we make use of unstructured news crawled from social media to alleviate the impact of financial fraud issue made on default probability prediction. Second, we propose a neural network method to integrate both structured financial factors and unstructured social media data with appropriate time alignment for default probability prediction. Extensive experimental results demonstrate the effectiveness of DMDP in predicting default probability for the listed companies in mainland China, compared with various baselines.
更多查看译文
关键词
Default probability prediction, Time series, Multi-source data
AI 理解论文
溯源树
样例
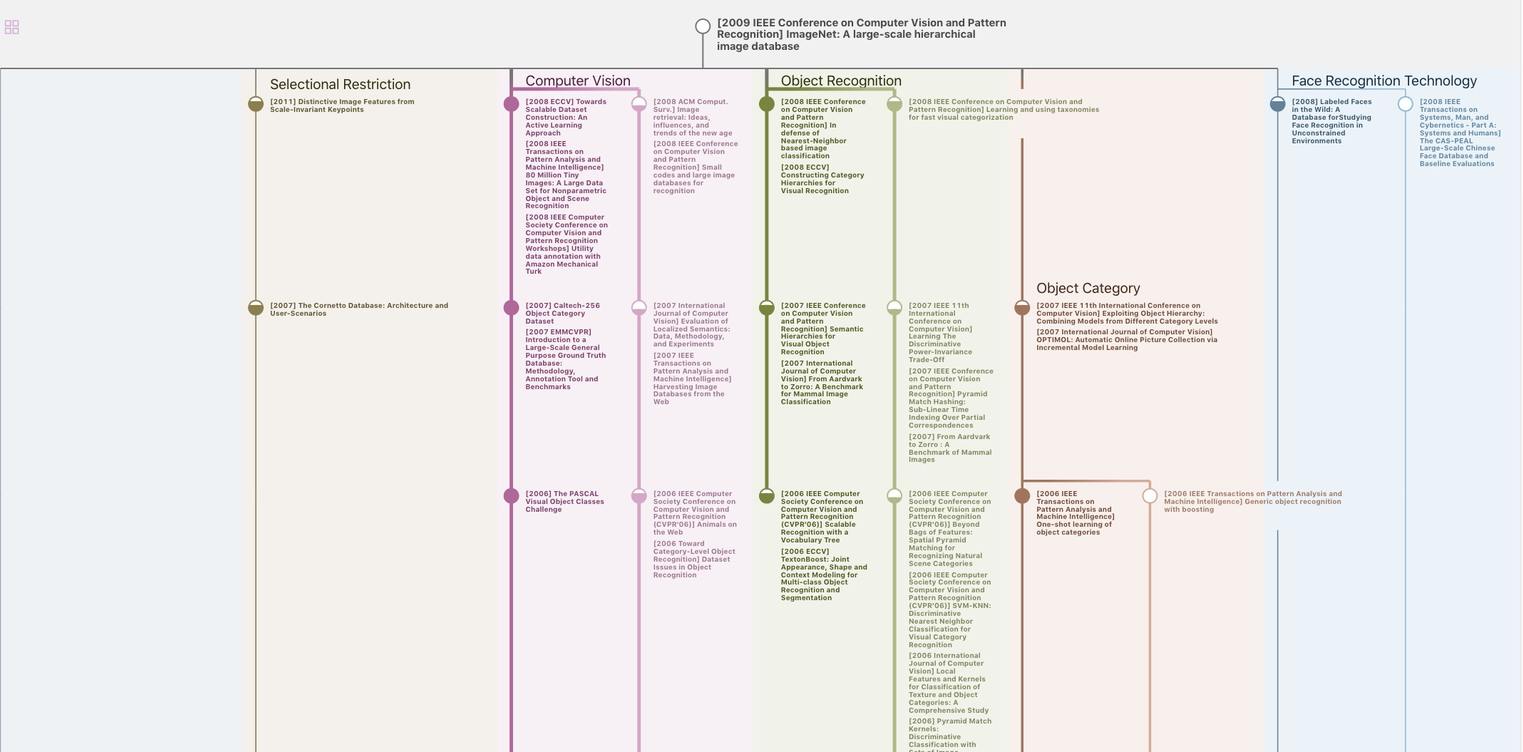
生成溯源树,研究论文发展脉络
Chat Paper
正在生成论文摘要