Hierarchical Multi-Agent Deep Reinforcement Learning To Develop Long-Term Coordination
SAC '19: PROCEEDINGS OF THE 34TH ACM/SIGAPP SYMPOSIUM ON APPLIED COMPUTING(2019)
摘要
Multi-agent systems need to communicate to coordinate a shared task. We show that a recurrent neural network (RNN) can learn a communication protocol for coordination, even if the actions to coordinate lie outside of the communication range. We also show that a single RNN is unable to do this if there is an independent action sequence necessary before the coordinated action can be executed. We propose a hierarchical deep reinforcement learning model for multi-agent systems that separates the communication and coordination task from the action picking through a hierarchical policy. As a testbed, we propose the Dungeon Lever Game and we extend the Differentiable Inter-Agent Learning (DIAL) framework [ 3]. First we prove that the agents need a hierarchical policy to learn communication and actions and, second, we present results from our successful model of the Dungeon Lever Game.
更多查看译文
关键词
Hierarchical Learning,Multi-Agent Systems,Agent Communication,Deep Reinforcement Learning
AI 理解论文
溯源树
样例
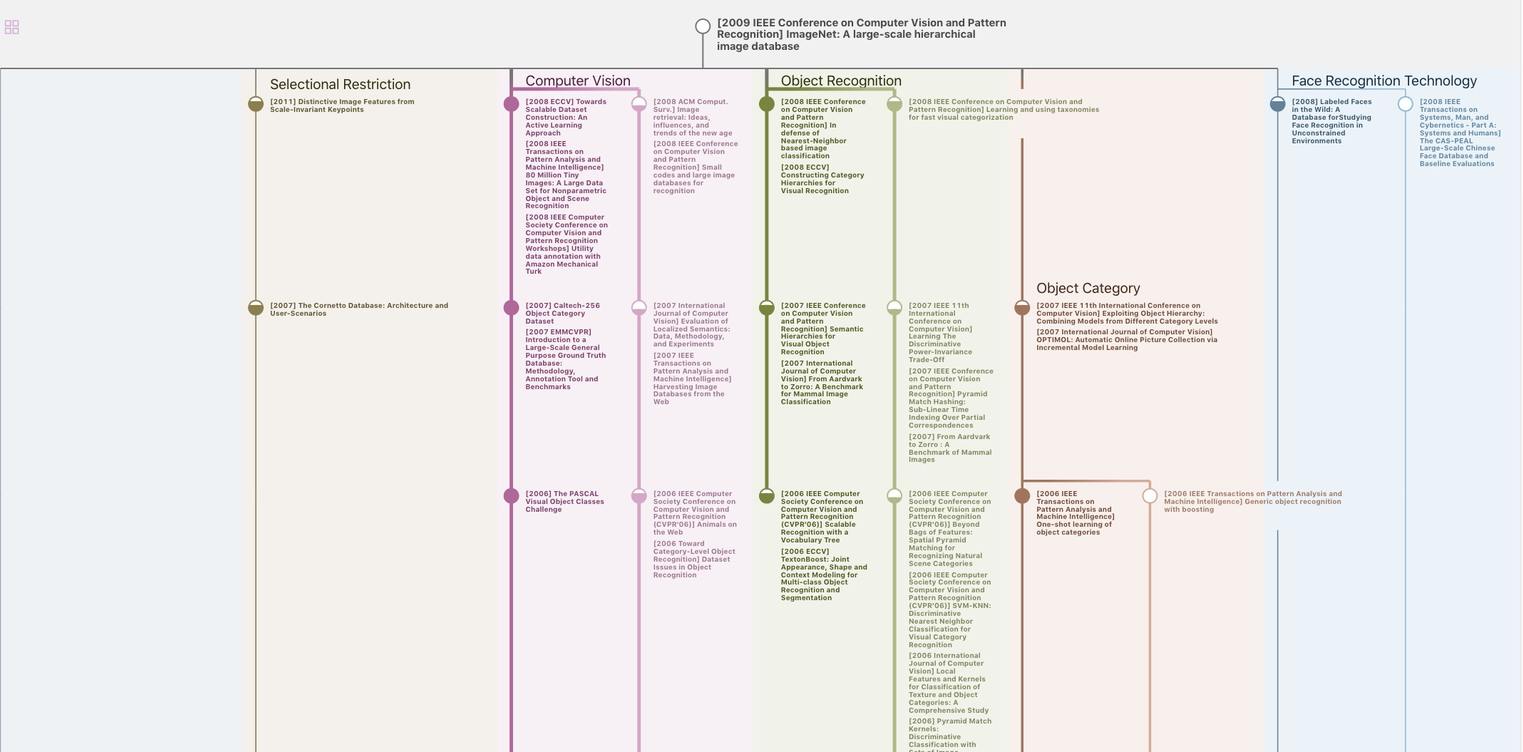
生成溯源树,研究论文发展脉络
Chat Paper
正在生成论文摘要