Embedding Cardinality Constraints in Neural Link Predictors
SAC '19: PROCEEDINGS OF THE 34TH ACM/SIGAPP SYMPOSIUM ON APPLIED COMPUTING(2018)
摘要
Neural link predictors learn distributed representations of entities and relations in a knowledge graph. They are remarkably powerful in the link prediction and knowledge base completion tasks, mainly due to the learned representations that capture important statistical dependencies in the data. Recent works in the area have focused on either designing new scoring functions or incorporating extra information into the learning process to improve the representations. Yet the representations are mostly learned from the observed links between entities, ignoring commonsense or schema knowledge associated with the relations in the graph. A fundamental aspect of the topology of relational data is the cardinality information, which bounds the number of predictions given for a relation between a minimum and maximum frequency. In this paper, we propose a new regularisation approach to incorporate relation cardinality constraints to any existing neural link predictor without affecting their efficiency or scalability. Our regularisation term aims to impose boundaries on the number of predictions with high probability, thus, structuring the embeddings space to respect commonsense cardinality assumptions resulting in better representations. Experimental results on Freebase, WordNet and YAGO show that, given suitable prior knowledge, the proposed method positively impacts the predictive accuracy of downstream link prediction tasks.
更多查看译文
关键词
Knowledge graphs,cardinality constraints,commonsense knowledge,regularisation
AI 理解论文
溯源树
样例
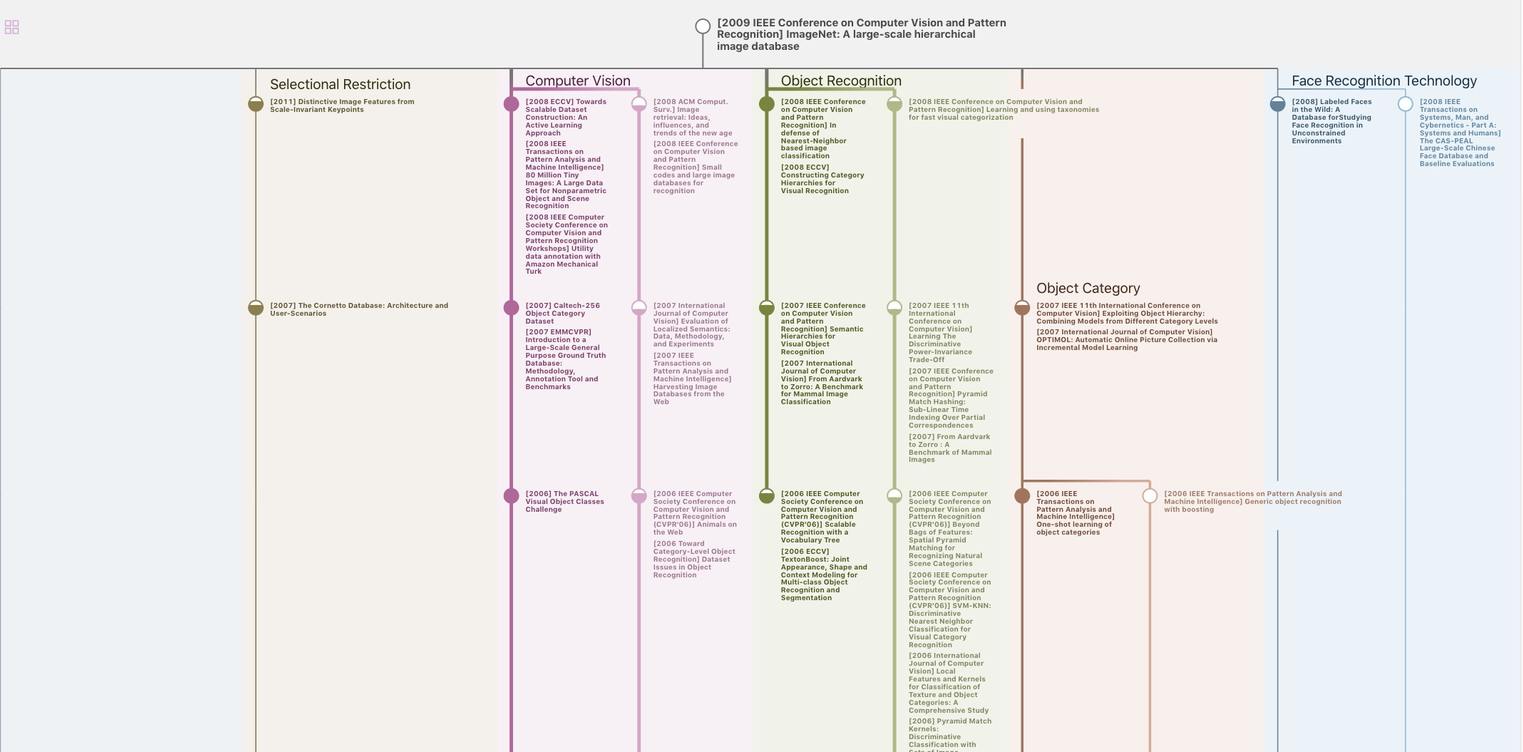
生成溯源树,研究论文发展脉络
Chat Paper
正在生成论文摘要