Neighborhood attribute reduction approach to partially labeled data
Granular Computing(2018)
摘要
Presently, from the viewpoint of rough set, most of the attribute reductions are only suitable for analyzing samples with complete labels. However, in many real-world applications, it is difficult to acquire the detailed labels of all samples, it follows that many attribute reductions may be ineffective for data with both labeled and unlabeled samples, i.e., partially labeled data. To fill such a gap, the attribute reduction is explored by neighborhood rough set over partially labeled data. First, two different measurements are combined for evaluating the importance of attribute, which comes from the labeled and unlabeled samples, respectively. Second, a heuristic algorithm is re-designed using such combined importance for computing reduct. Finally, by considering several different ratios of missing labels over UCI datasets, the experimental results demonstrate that the reducts derived by our approach not only reduce the degree of uncertainty, but also offer us better classification performance. Therefore, the main contribution of this paper is to construct an effective attribute reduction strategy for partially labeled data. Moreover, this research also suggests new applications for considering attribute reduction problems in complex data.
更多查看译文
关键词
Attribute reduction, Missing labels, Neighborhood rough set, Partially labeled data
AI 理解论文
溯源树
样例
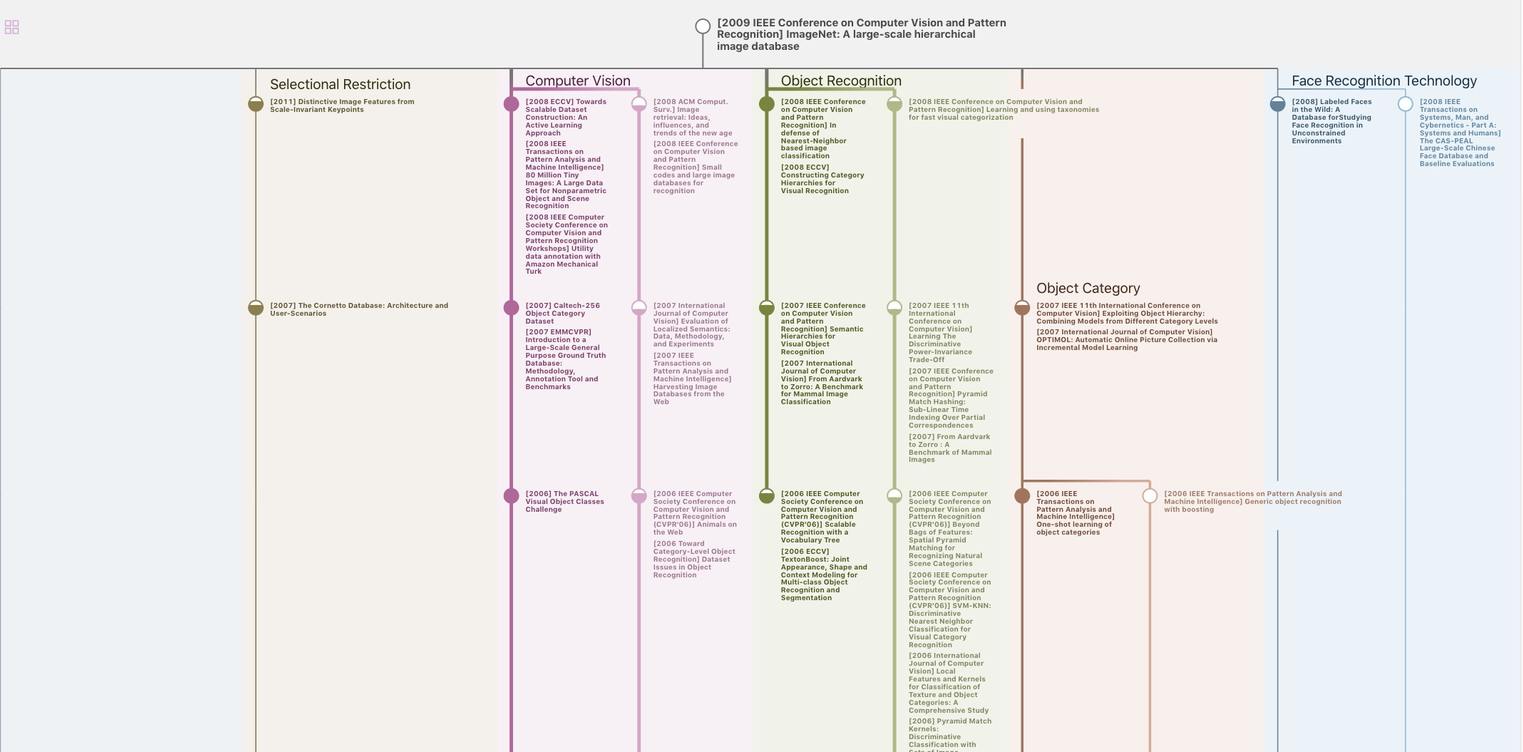
生成溯源树,研究论文发展脉络
Chat Paper
正在生成论文摘要