Time to Scale - Generalizable Affect Detection for Tens of Thousands of Students across An Entire School Year.
CHI(2019)
摘要
We developed generalizable affect detectors using 133,966 instances of 18 affective states collected from 69,174 students who interacted with an online math learning platform called Algebra Nation over the entire school year. To enable scalability and generalizability, we used generic interaction features (e.g., viewing a video, taking a quiz), which do not require specialized sensors and are domain- and (to a certain extent) system-independent. We experimented with standard classifiers, recurrent neural networks, and genetically evolved neural networks for affect modeling. Prediction accuracies, quantified with Spearman's rho, were modest and ranged from .08 (for surprise) to .34 (for happiness) with a mean of .25. Our model trained on Algebra students generalized to a different set of Geometry students (n = 28,458) on the same platform. We discuss implications for scaling up affect detection for affect-sensitive online learning environments which aim to improve engagement and learning by detecting and responding to student affect.
更多查看译文
关键词
affect detection, machine learning, online education, sensor-free
AI 理解论文
溯源树
样例
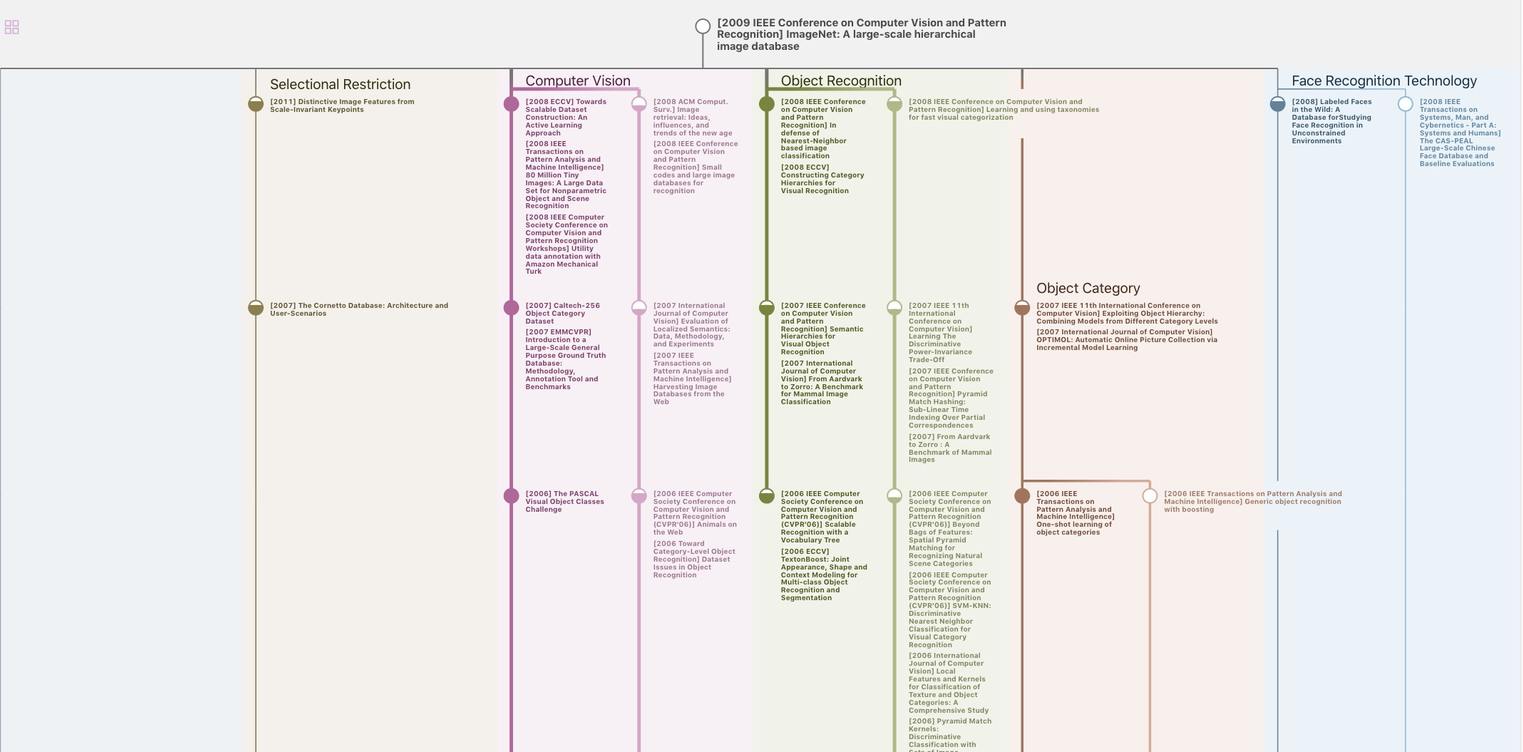
生成溯源树,研究论文发展脉络
Chat Paper
正在生成论文摘要