Methodological Gaps in Predicting Mental Health States from Social Media - Triangulating Diagnostic Signals.
CHI(2019)
摘要
A growing body of research is combining social media data with machine learning to predict mental health states of individuals. An implication of this research lies in informing evidence-based diagnosis and treatment. However, obtaining clinically valid diagnostic information from sensitive patient populations is challenging. Consequently, researchers have operationalized characteristic online behaviors as "proxy diagnostic signals" for building these models. This paper posits a challenge in using these diagnostic signals, purported to support clinical decision-making. Focusing on three commonly used proxy diagnostic signals derived from social media, we find that predictive models built on these data, although offer strong internal validity, suffer from poor external validity when tested on mental health patients. A deeper dive reveals issues of population and sampling bias, as well as of uncertainty in construct validity inherent in these proxies. We discuss the methodological and clinical implications of these gaps and provide remedial guidelines for future research.
更多查看译文
关键词
construct validity, machine learning, mental health, population bias, sampling bias, social media, validity theory
AI 理解论文
溯源树
样例
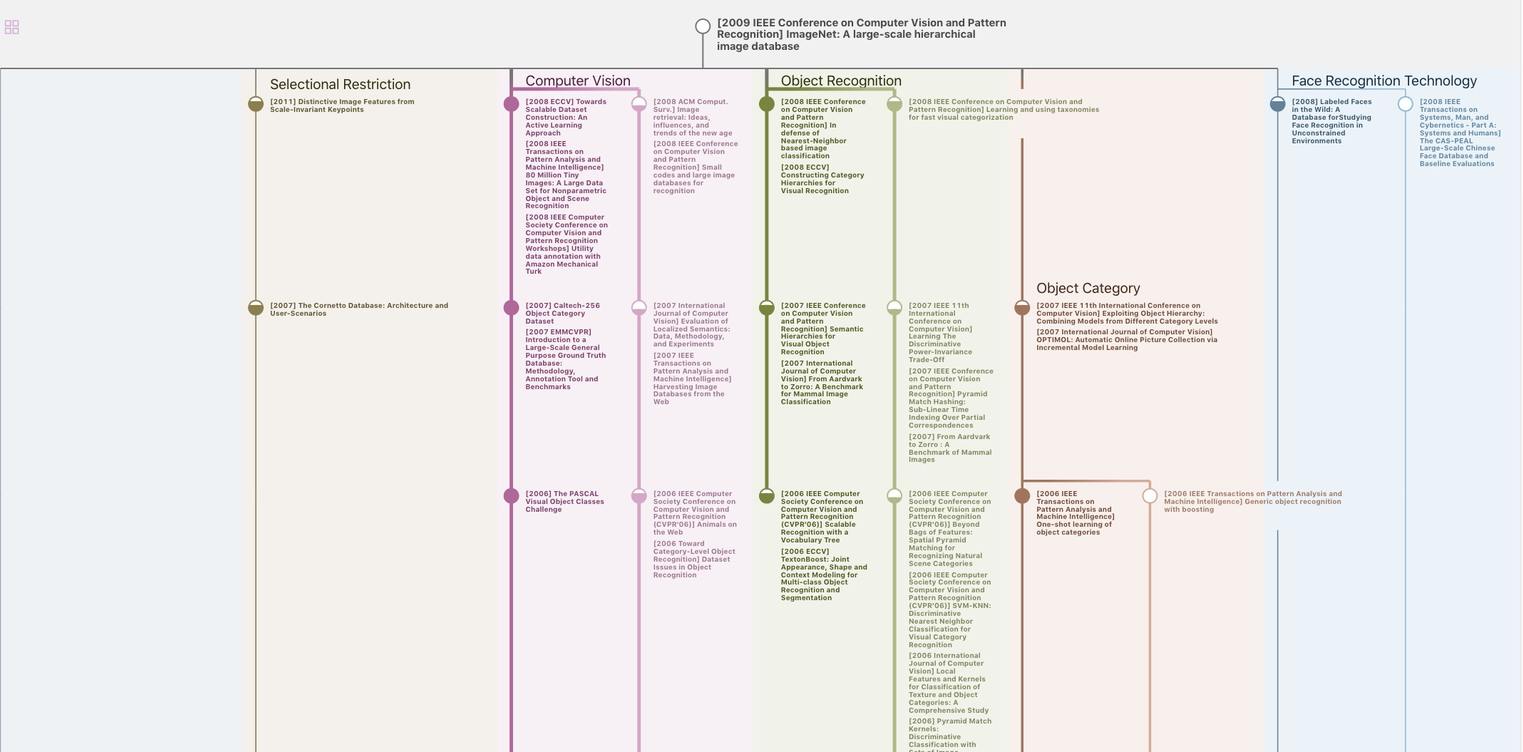
生成溯源树,研究论文发展脉络
Chat Paper
正在生成论文摘要