An Auc-Based Active Learning Algorithm Via Logitboost For Binary Classification
2018 7TH INTERNATIONAL CONGRESS ON ADVANCED APPLIED INFORMATICS (IIAI-AAI 2018)(2018)
摘要
When labeling subjects is quite expensive, to incorporate both labeled and unlabeled data in training a classifier becomes a common strategy. Semi-supervised learning is widely applied for this situation and typically uses a small amount of labeled data with a large amount of unlabeled data to train classifiers. Active learning, one kind of semi-supervised learning, is able to interactively query some information to get new subjects' labels/classes and is able to reduce cost because only the selected subjects need to be exanimated labels. Additionally, boosting algorithm is an ensemble learning algorithm for reducing bias and variations in supervised learning. Area under ROC curve is a widely used criterion for evaluating the predictive performance of a classifier. Hence, in this paper, we proposed a boosting-base active learning algorithm with optimizing AUC for binary classification. The simulation results show that the proposed method can achieve good predictive performance with using fewer samples.
更多查看译文
关键词
active learning, area under ROC curve (AUC), binary classification, boosting
AI 理解论文
溯源树
样例
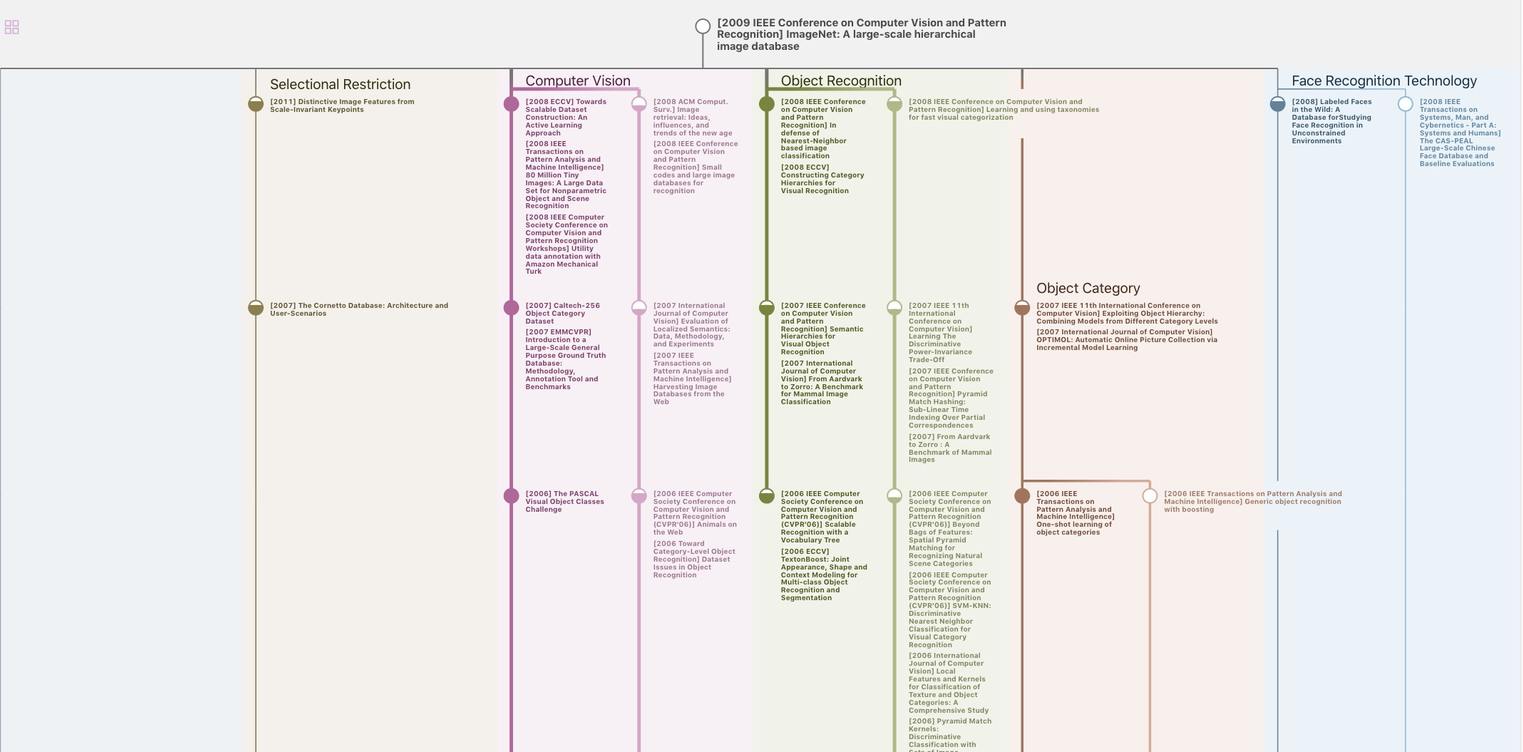
生成溯源树,研究论文发展脉络
Chat Paper
正在生成论文摘要