Unsupervised Human Activity Analysis for Intelligent Mobile Robot
KI(2018)
摘要
The success of intelligent mobile robots operating and collaborating with humans in daily living environments depends on their ability to generalise and learn human movements, and obtain a shared understanding of an observed scene. In this thesis we aim to understand human activities being performed in real-world environments from long-term observation from an autonomous mobile robot. A number of qualitative spatial–temporal representations are used to capture different aspects of the relations between human subjects and their environment. Analogous to information retrieval on text corpora, a generative probabilistic technique is used to recover latent, semantically-meaningful concepts in the encoded observations in an unsupervised manner. The small number of concepts discovered are considered as human activity classes, granting the robot a low-dimensional understanding of visually observed complex scenes. Finally, variational inference is used to facilitate incremental updating of such concepts allowing for efficient learning and updating of human activity models over time, resulting in efficient life-long learning.
更多查看译文
关键词
Human activity analysis,Mobile robotics,Qualitative spatio-temporal representation,Probabilistic machine learning,Latent Dirichlet allocation
AI 理解论文
溯源树
样例
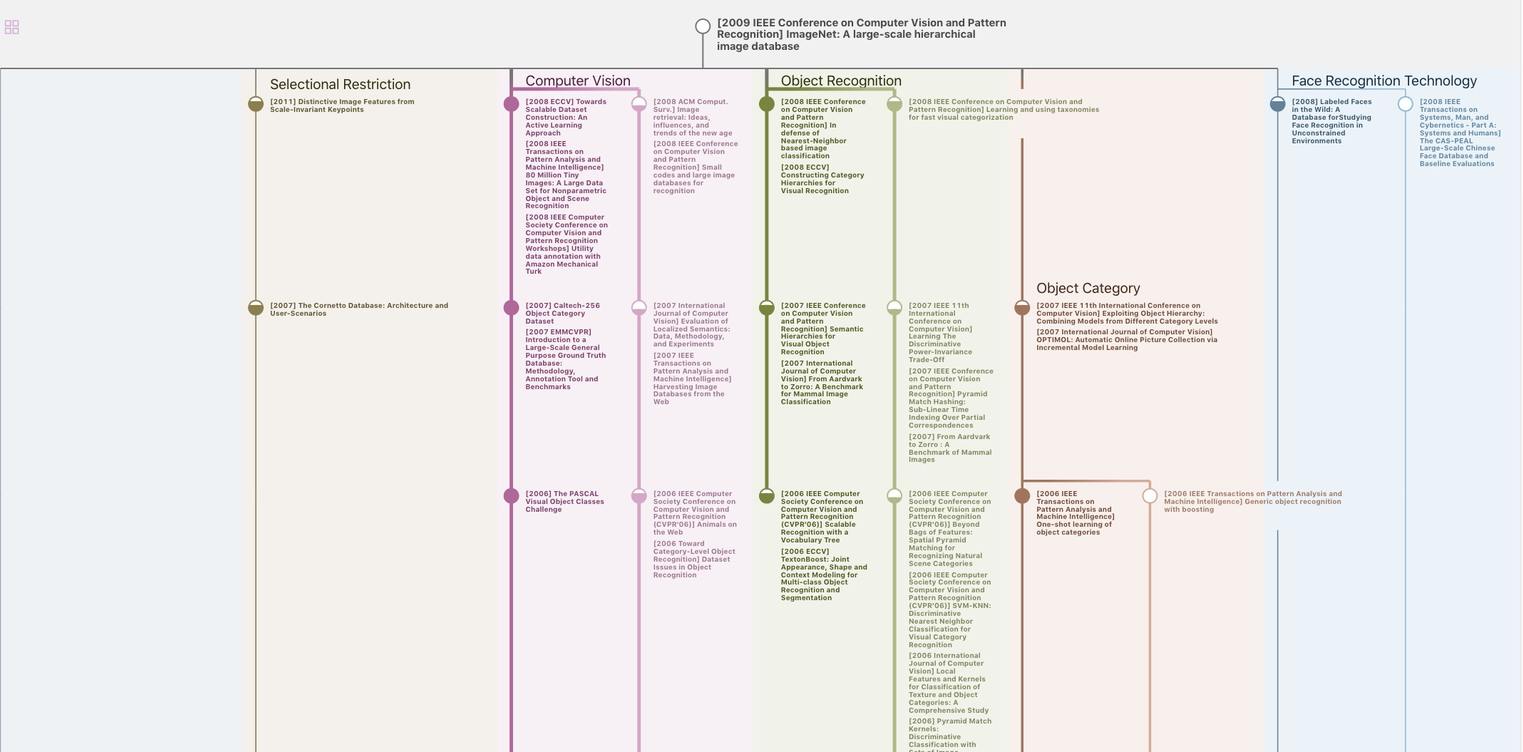
生成溯源树,研究论文发展脉络
Chat Paper
正在生成论文摘要