Multimodal classification of drug-naïve first-episode schizophrenia combining anatomical, diffusion and resting state functional resonance imaging.
Neuroscience letters(2019)
摘要
The accurate diagnosis in the early stage of schizophrenia (SZ) is of great importance yet remains challenging. The classification between SZ and control groups based on magnetic resonance imaging (MRI) data using machine learning method could be helpful for SZ diagnosis. Increasing evidence showed that the combination of multimodal MRI data might further improve the classification performance However, medication effect has a profound influence on patients' anatomical and functional features and may reduce the classification efficiency. In this paper, we proposed a multimodal classification method to discriminate drug-naïve first-episode schizophrenia patients from healthy controls (HCs) by a combined structural MRI, diffusion tensor imaging (DTI) and resting state-functional MRI data. To reduce the feature dimension of multimodal data, we applied sparse coding (SC) for feature selection and multi-kernel support vector machine (SVM) for feature combination and classification. The best classification performance with the classification accuracy of 84.29% and area under the receiver operating characteristic (ROC) curve (AUC) of 81.64% was achieved when all modality data were combined. Interestingly, the identified functional markers were mainly found in default mode network (DMN) and cerebellar connections, while the structural markers were within limbic system and prefrontal-thalamo-hippocampal circuit.
更多查看译文
AI 理解论文
溯源树
样例
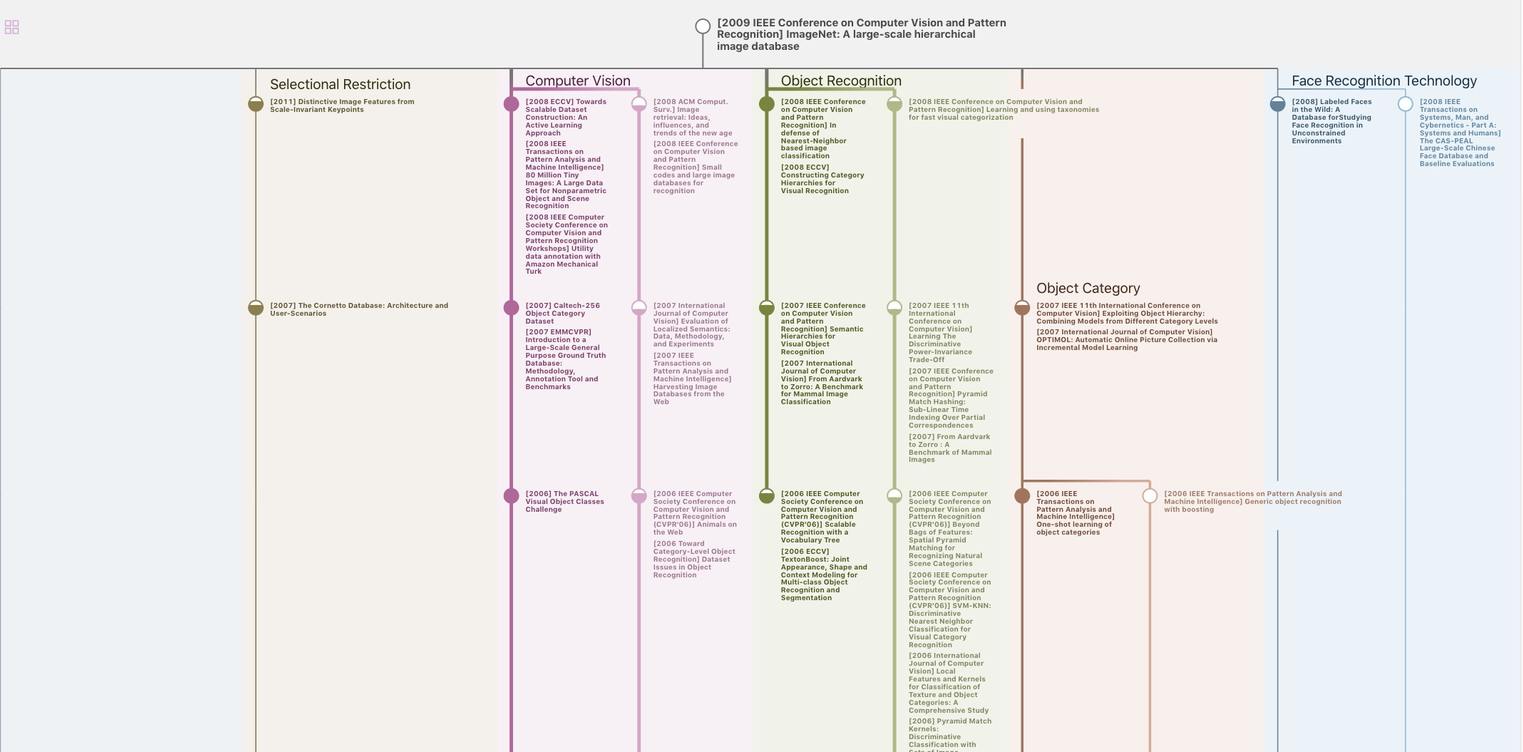
生成溯源树,研究论文发展脉络
Chat Paper
正在生成论文摘要