Risk-Averse Selfish Routing.
MATHEMATICS OF OPERATIONS RESEARCH(2019)
摘要
We consider a nonatomic selfish routing model with independent stochastic travel times for each edge, represented by mean and variance latency functions that depend on edge flows. This model can apply to traffic in the Internet or in a road network. Variability negatively impacts packets or drivers by introducing jitter in transmission delays, which lowers quality of streaming audio or video, or by making it more difficult to predict the arrival time at destination. At equilibrium, agents may select paths that do not minimize the expected latency so as to obtain lower variability. A social planner, who is likely to be more risk neutral than agents because it operates at a longer time scale, quantifies social cost with the total expected delay along routes. From that perspective, agents may make suboptimal decisions that degrade long-term quality. We define the price of risk aversion (PRA) as the worst-case ratio of the social cost at a risk-averse Wardrop equilibrium to that where agents are risk neutral. This inefficiency metric captures the degradation of system performance caused by variability and risk aversion. For networks with general delay functions and a single source-sink pair, we first show upper bounds for the PRA that depend linearly on the agents' risk tolerance and on the degree of variability present in the network. We call these bounds structural, as they depend on the structure of the network. To get this result, we rely on a combinatorial proof that employs alternating paths that are reminiscent of those used in max-flow algorithms. For series-parallel graphs, the PRA becomes independent of the network topology and its size. Next, we provide tight and asymptotically tight lower bounds on the PRA by showing a family of structural lower bounds, which grow linearly with the number of nodes in the graph and players' risk aversion. These are tight for graph sizes that are powers of 2. After that, by focusing on restricting the set of allowable mean latency and variance functions, we derive functional bounds on the PRA that are asymptotically tight and depend on the allowed latency functions but not on the topology. The functional bounds match the price-of-anarchy bounds for congestion games multiplied by an extra factor that accounts for risk aversion. Finally, we turn to the mean-standard deviation user objective-a much more complex model of risk aversion because the cost of a path is nonadditive over edge costs-and provide tight bounds for instances that admit alternating paths with one or two forward subpaths.
更多查看译文
关键词
stochastic Wardrop game,risk aversion,price of anarchy
AI 理解论文
溯源树
样例
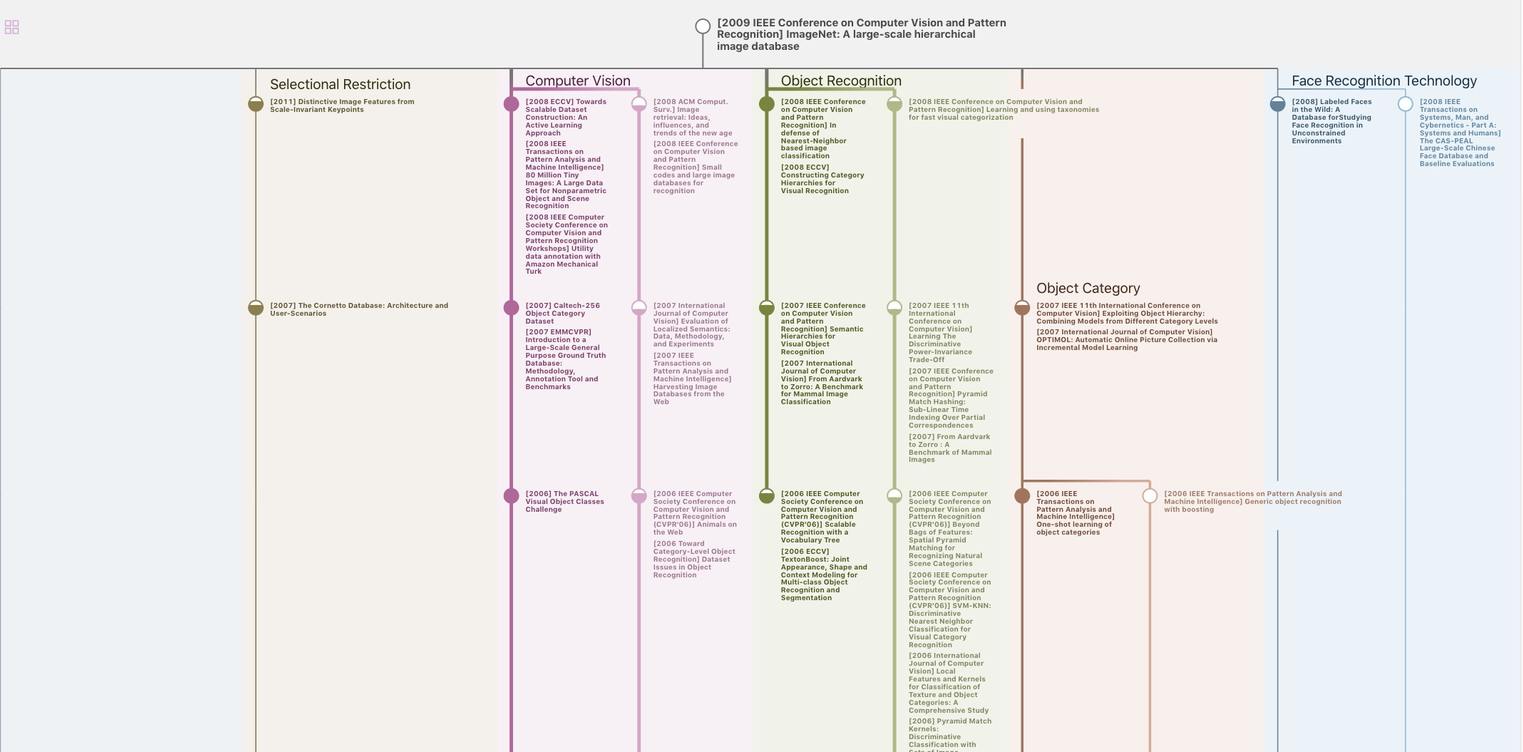
生成溯源树,研究论文发展脉络
Chat Paper
正在生成论文摘要