Beyond feature integration: a coarse-to-fine framework for cascade correlation tracking
Mach. Vis. Appl.(2019)
摘要
Discriminative correlation filters (DCF) have achieved enormous popularity in the tracking community. Recently, the performance advancement in DCF-based trackers is predominantly driven by the use of convolutional features. In pursuit of extreme tracking performance, state-of-the-art trackers (e.g., cascade correlation tracking [ 1 ] and HCF [ 2 ]) equip DCF with hierarchical convolutional features to capture both semantics and spatial details of the target appearance. While such methods have been shown to work well, multiple feature integration results in high model complexity which significantly increases the over-fitting risk and computational burden. In this paper, we present a coarse-to-fine framework for cascade correlation tracking (CCT). Instead of integrating hierarchical features, this framework decomposes a complicated tracker into two low-complexity modules, a coarse tracker 𝒞 and a refined tracker ℛ , working in a coarse-to-fine manner. The coarse tracker 𝒞 employs low-resolution semantic convolutional features extracted from a large search area to cope with large target displacement and appearance change between adjacent frames. By contrast, the refined tracker ℛ employs high-resolution handcraft features extracted from a small search area to further refine the coarse location of 𝒞 . Our CCT tracker enjoys the strong discriminative power of 𝒞 and the high efficiency of ℛ . Experiments on the OTB2013 and TC128 benchmarks show that CCT performs favorably against state-of-the-art trackers.
更多查看译文
关键词
Correlation filter,Coarse to fine,Visual tracking
AI 理解论文
溯源树
样例
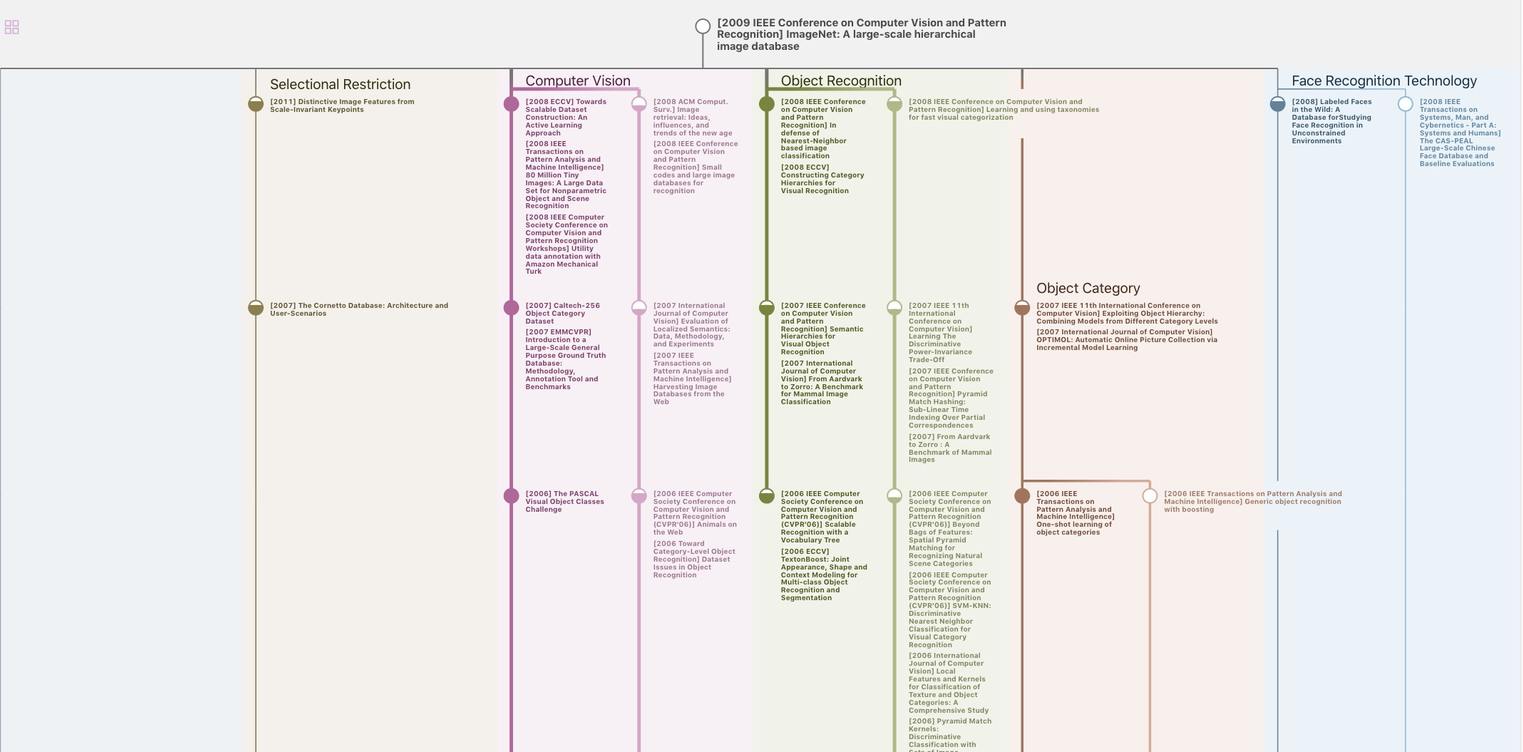
生成溯源树,研究论文发展脉络
Chat Paper
正在生成论文摘要