Stacked Fully Convolutional Networks for Pulmonary Vessel Segmentation
2018 IEEE Visual Communications and Image Processing (VCIP)(2018)
摘要
Accurate pulmonary vessel segmentation in non-contrast pulmonary computed tomography (CT) images is significant for vessel reconstruction and disease diagnosis. Recently, there is an increased interest in applying Convolutional Neural Networks (CNNs) in biomedical images analysis. However, most of the existing approaches suffer from discontinuity problem in pulmonary vessel segmentation due to blurry boundary and complicated pulmonary elements. To address this problem, we propose Stacked Fully Convolutional Networks for Pulmonary Vessel Segmentation (SFCNPVS) which consists of a stacked Fully Convolutional Networks (FCNS) and an orientation-based region growing method. The first fully convolutional network is presented to extract lung and alleviate distraction from mediastinum. The second fully con-volutional network takes result from previous network as input and generates the vessel probability map. To further dispose the non-vascular components, we introduce a novel orientation-based region growing approach that encourages smoothness of vessels in 3D space. We conduct extensive experiments on realistic non-contrast pulmonary CT datasets, and show that the proposed approach achieves the best performance on pulmonary vessel segmentation task.
更多查看译文
关键词
Pulmonary vessel segmentation,region growing,fully convolutional network
AI 理解论文
溯源树
样例
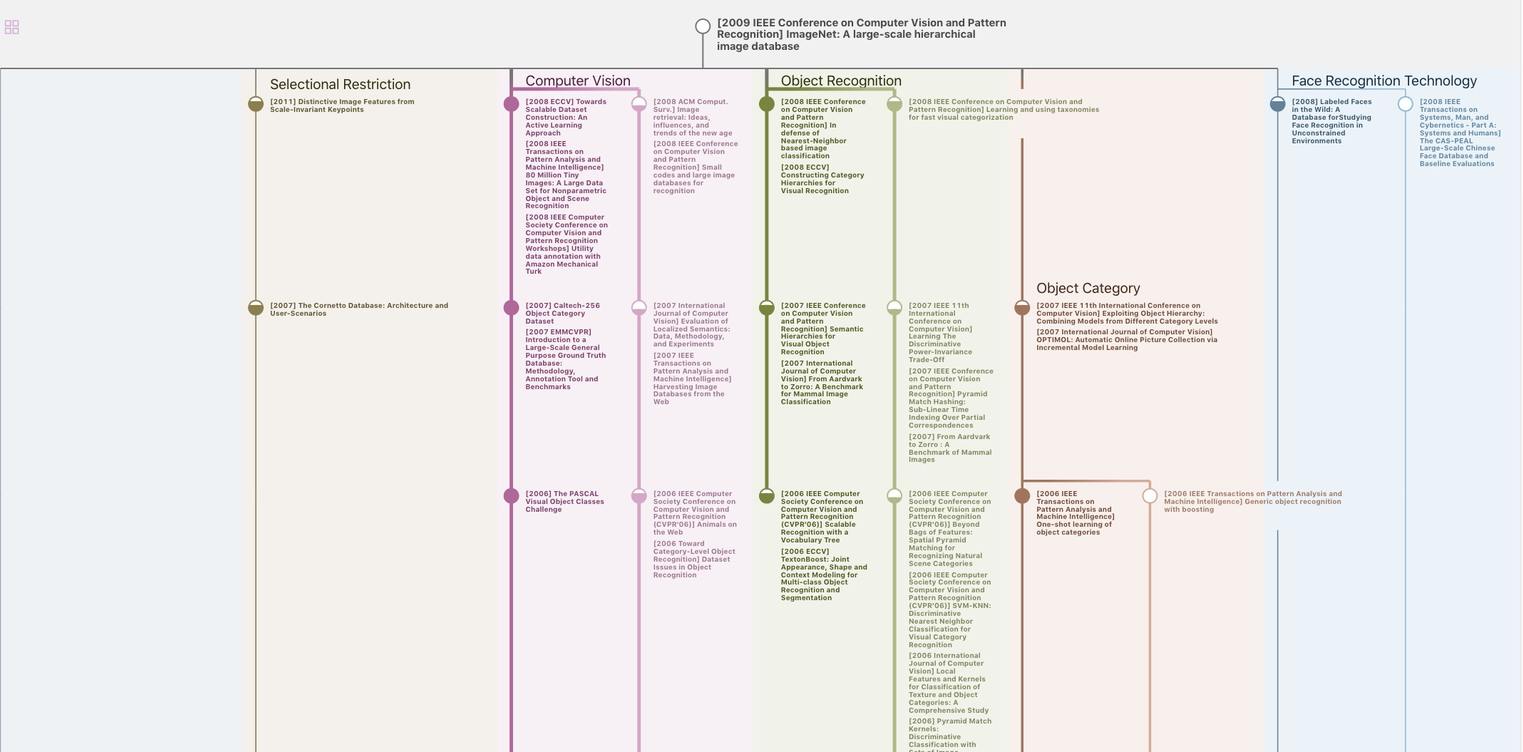
生成溯源树,研究论文发展脉络
Chat Paper
正在生成论文摘要