Multi-Label Deep Sparse Hashing
2018 IEEE Visual Communications and Image Processing (VCIP)(2018)
摘要
In this paper, we propose a multi-label deep sparse hashing (MDSH) to learn compact binary codes for efficient image retrieval. Unlike most existing supervised hashing methods which only exploit pairwise or triplet-wise similarity to learn binary codes, we perform deep network training such that optimal binary codes are obtained from a sparsity-based discriminative criterion. Specifically, we learn our hashing network by solving a multi-label classification problem with a sparse cross-entropy loss which ensures that sparse probabilities can be obtained while also learning the binary codes. By doing so, our network is able to scale well with ground truth labels which are generally sparse. Experimental results on two widely used multi-label image hashing datasets are presented to show the effectiveness of our proposed approach.
更多查看译文
关键词
hashing,image retrieval,deep learning
AI 理解论文
溯源树
样例
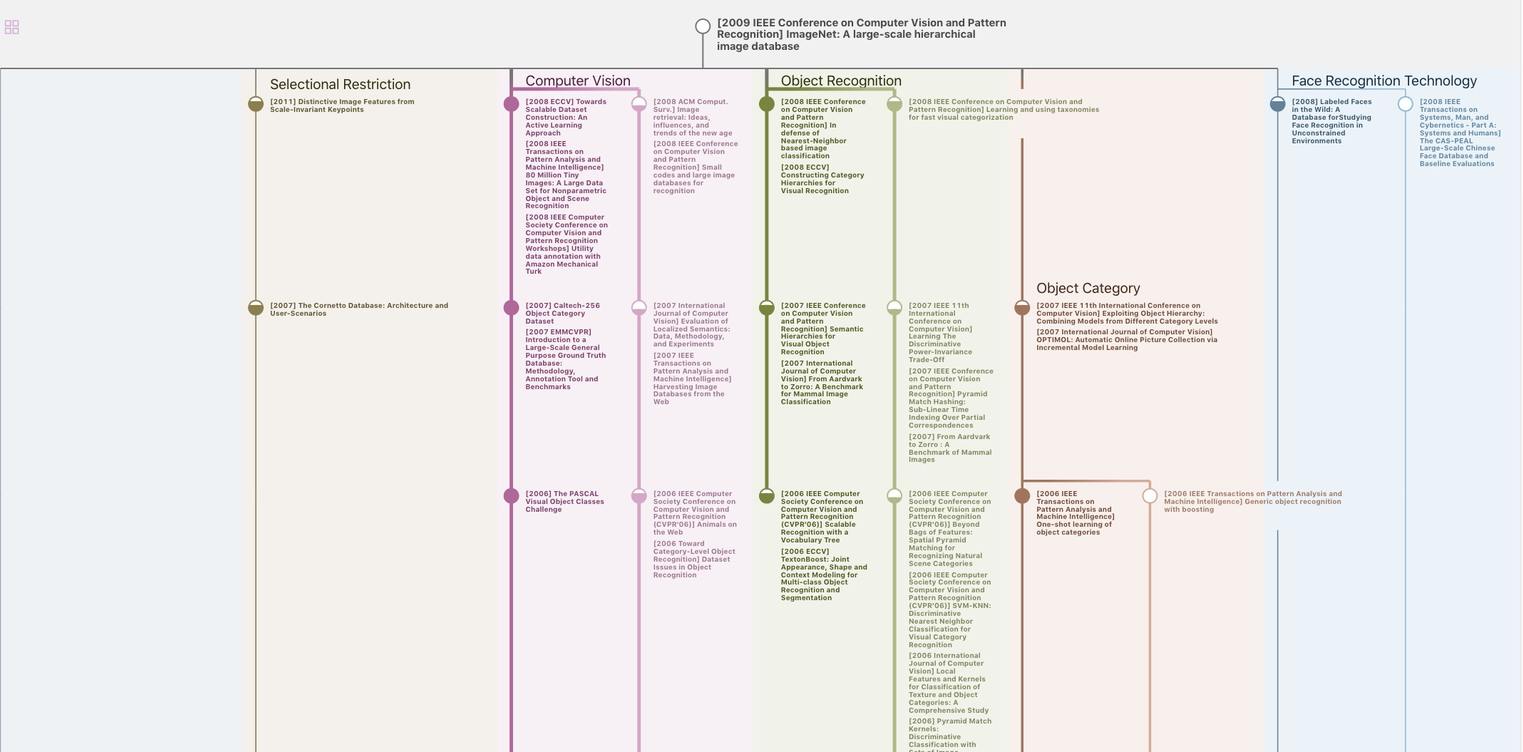
生成溯源树,研究论文发展脉络
Chat Paper
正在生成论文摘要