Improving Few-Shot User-Specific Gaze Adaptation Via Gaze Redirection Synthesis
2019 IEEE/CVF CONFERENCE ON COMPUTER VISION AND PATTERN RECOGNITION (CVPR 2019)(2019)
摘要
As an indicator of human attention gaze is a subtle behavioral cue which can be exploited in many applications. However, inferring 3D gaze direction is challenging even for deep neural networks given the lack of large amount of data (groundtruthing gaze is expensive and existing datasets use different setups) and the inherent presence of gaze biases due to person-specific difference. In this work, we address the problem ofperson-specific gaze model adaptation from only a few reference training samples. The main and novel idea is to improve gaze adaptation by generating additional training samples through the synthesis of gaze-redirected eye images from existing reference samples. In doing so, our contributions are threefold: (i) we design our gaze redirection framework from synthetic data, allowing us to benefit from aligned training sample pairs to predict accurate inverse mapping fields; (ii) we proposed a self-supervised approach for domain adaptation; (iii) we exploit the gaze redirection to improve the performance of person-specific gaze estimation. Extensive experiments on two public datasets demonstrate the validity of our gaze re-targeting and gaze estimation framework.
更多查看译文
关键词
Face,Gesture,and Body Pose,Image and Video Synthesis, Vision Applications and Systems
AI 理解论文
溯源树
样例
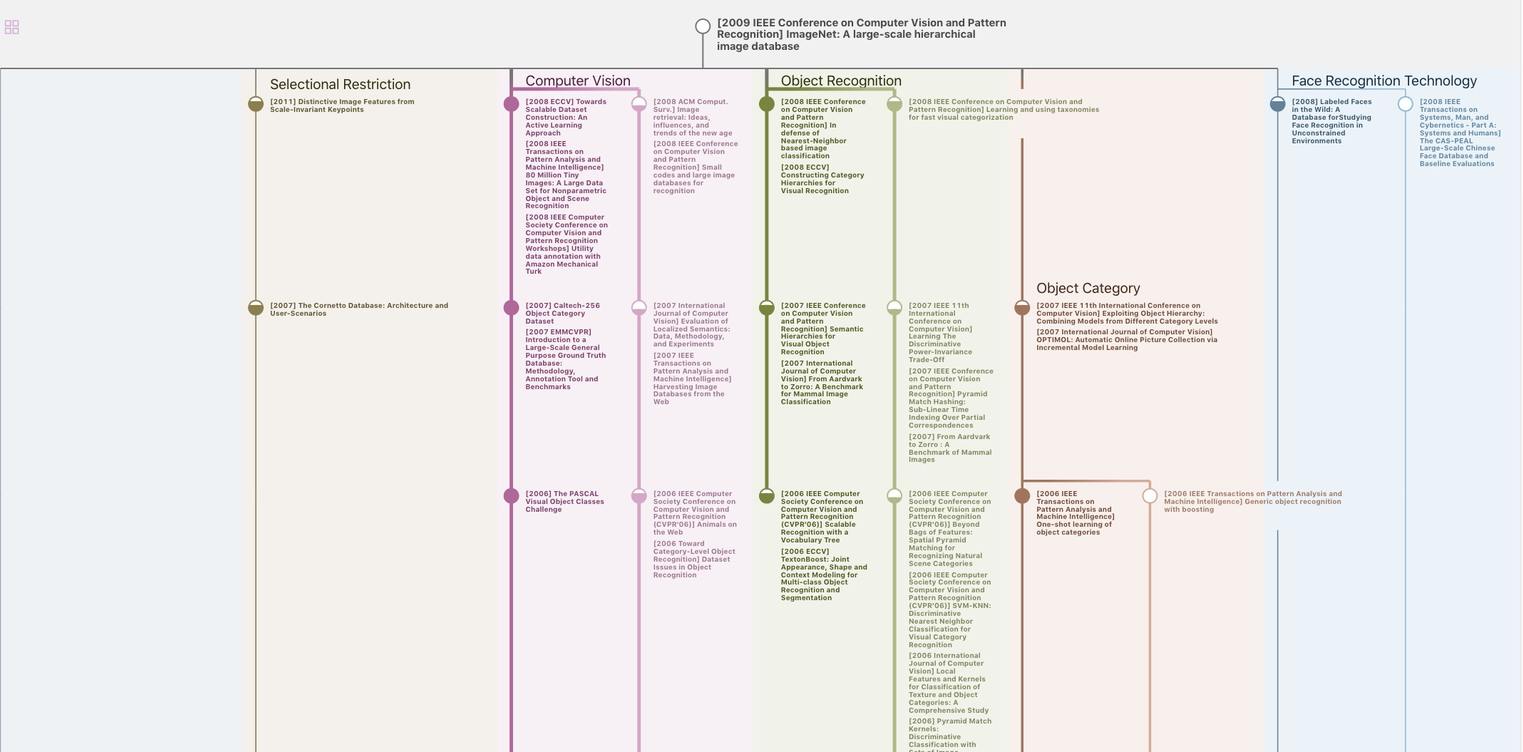
生成溯源树,研究论文发展脉络
Chat Paper
正在生成论文摘要