Using model uncertainty for robust optimization in approximate inference control
Artificial Life and Robotics(2017)
摘要
Recently, the optimization-by-inference approach has been proposed as a new means for solving high-dimensional optimization problems quickly. Approximate Inference COntrol (AICO) is one of the most successful and promising methods that implement the optimization-by-inference approach. AICO is able to solve stochastic optimal control problems and has already been successfully used in many applications. However, it is known that the iterative inference of AICO sometimes fails to converge to the optimal solution. To make the optimization more robust, in this paper, we propose to take model uncertainty into account. In AICO, the cost function to be minimized is accurate around a particular state of a given stochastic system, but the accuracy is uncertain in regions far from that state. Because using such an uncertain function is harmful to the convergence, we modify AICO, so that it does not use the function in uncertain regions. Our method is easy to implement and does not add much computational time to the original AICO. Experiments using two different scenarios show that our method substantially improves AICO in terms of the rate at which the algorithm produces convergent results.
更多查看译文
关键词
Approximate inference control,Stochastic optimal control,Optimization-by-inference approach,Model uncertainty
AI 理解论文
溯源树
样例
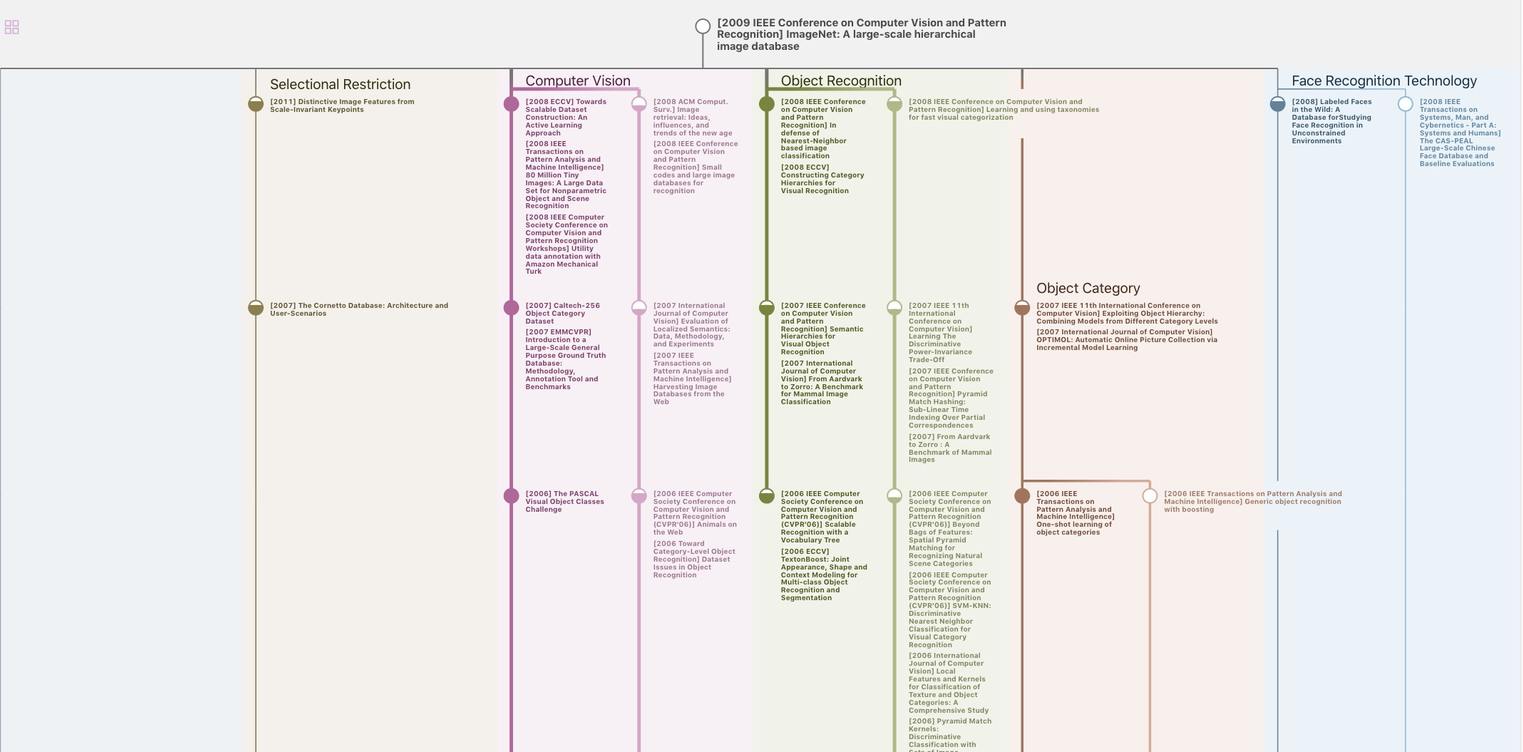
生成溯源树,研究论文发展脉络
Chat Paper
正在生成论文摘要