2d3d-Matchnet: Learning To Match Keypoints Across 2d Image And 3d Point Cloud
2019 INTERNATIONAL CONFERENCE ON ROBOTICS AND AUTOMATION (ICRA)(2019)
摘要
Large-scale point cloud generated from 3D sensors is more accurate than its image-based counterpart. However, it is seldom used in visual pose estimation due to the difficulty in obtaining 2D-3D image to point cloud correspondences. In this paper, we propose the 2D3D-MatchNet - an end-to-end deep network architecture to jointly learn the descriptors for 2D and 3D keypoint from image and point cloud, respectively. As a result, we are able to directly match and establish 2D-3D correspondences from the query image and 3D point cloud reference map for visual pose estimation. We create our Oxford 2D-3D Patches dataset from the Oxford Robotcar dataset with the ground truth camera poses and 2D-3D image to point cloud correspondences for training and testing the deep network. Experimental results verify the feasibility of our approach.
更多查看译文
关键词
image-based counterpart,visual pose estimation,2D-3D image,cloud correspondences,end-to-end deep network architecture,query image,3D point cloud reference map,Oxford 2D-3D Patches dataset,Oxford Robotcar dataset,ground truth camera pose
AI 理解论文
溯源树
样例
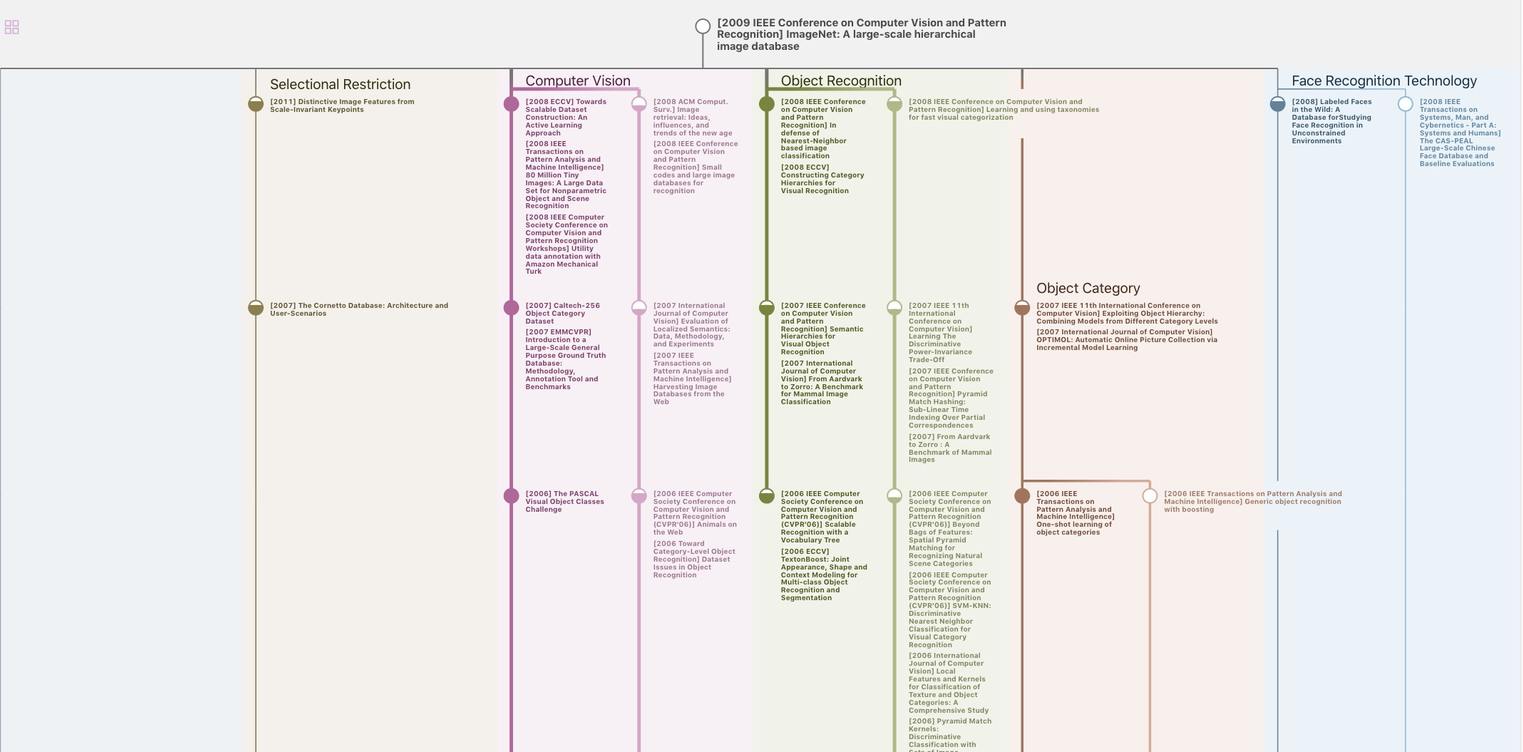
生成溯源树,研究论文发展脉络
Chat Paper
正在生成论文摘要