Linear Convergence Of Accelerated Conditional Gradient Algorithms In Spaces Of Measures
ESAIM-CONTROL OPTIMISATION AND CALCULUS OF VARIATIONS(2021)
摘要
A class of generalized conditional gradient algorithms for the solution of optimization problem in spaces of Radon measures is presented. The method iteratively inserts additional Dirac-delta functions and optimizes the corresponding coefficients. Under general assumptions, a sub-linear O(1/k) rate in the objective functional is obtained, which is sharp in most cases. To improve efficiency, one can fully resolve the finite-dimensional subproblems occurring in each iteration of the method. We provide an analysis for the resulting procedure: under a structural assumption on the optimal solution, a linear O(zeta(k)) convergence rate is obtained locally.
更多查看译文
关键词
Vector-valued finite Radon measures, generalized conditional gradient, sparsity, nonsmooth optimization
AI 理解论文
溯源树
样例
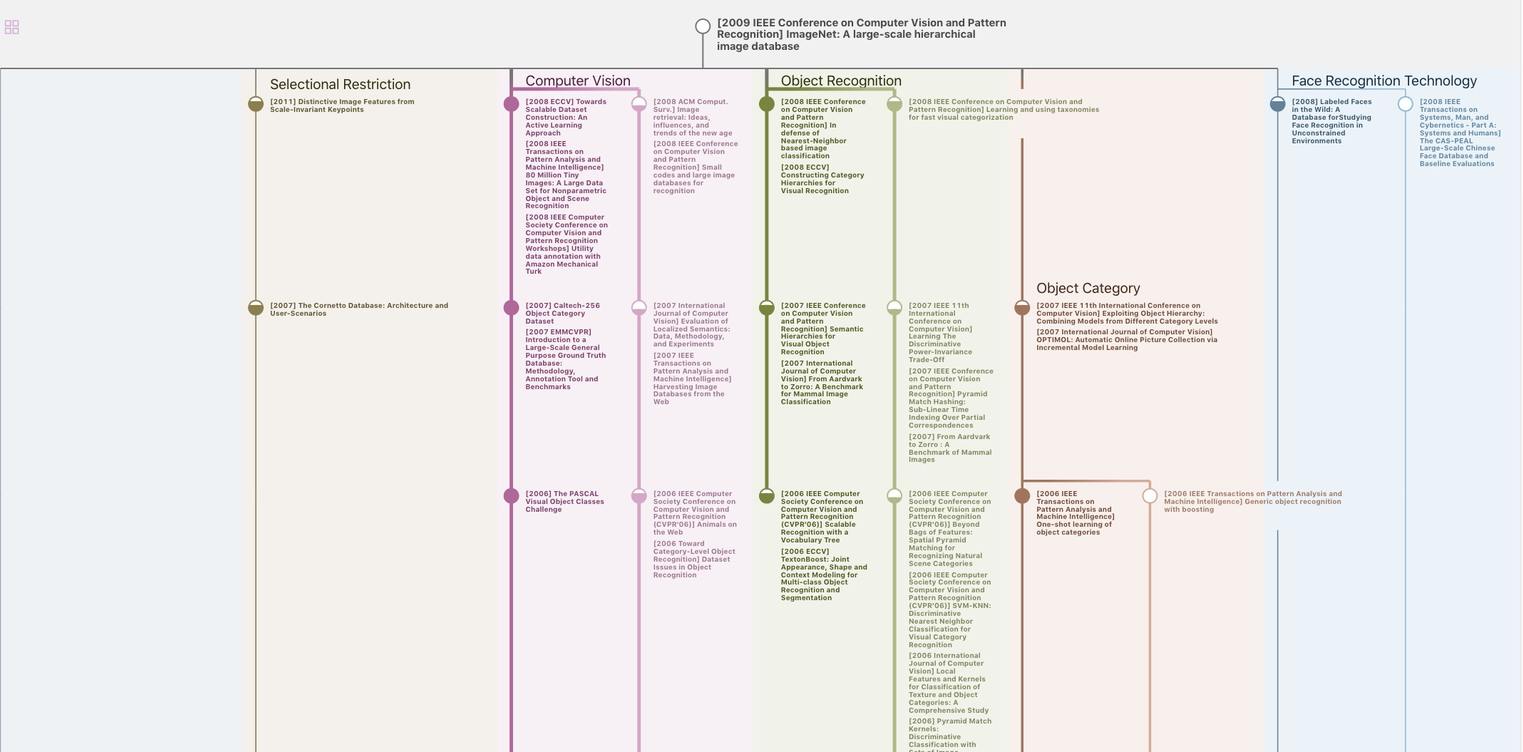
生成溯源树,研究论文发展脉络
Chat Paper
正在生成论文摘要