Nonconvex Optimization for 3-Dimensional Point Source Localization Using a Rotating Point Spread Function.
SIAM JOURNAL ON IMAGING SCIENCES(2019)
摘要
We consider the high-resolution imaging problem of 3-dimensional (3D) point source image recovery from 2-dimensional data using a method based on point spread function (PSF) engineering. The method involves a new technique, recently proposed by Prasad, based on the use of a rotating PSF with a single lobe to obtain depth from defocus. The amount of rotation of the PSF encodes the depth position of the point source. Applications include high-resolution single molecule localization microscopy as well as the problem addressed in this paper on localization of space debris using a space-based telescope. The localization problem is discretized on a cubical lattice where the coordinates of nonzero entries represent the 3D locations and the values of these entries the fluxes of the point sources. Finding the locations and fluxes of the point sources is a large-scale sparse 3D inverse problem. A new non-convex regularization method with a data-fitting term based on Kullback-Leibler (KL) divergence is proposed for 3D localization for the Poisson noise model. In addition, we propose a new scheme of estimation of the source fluxes from the KL data-fitting term. Numerical experiments illustrate the efficiency and stability of the algorithms that are trained on a random subset of image data before being applied to other images. Our 3D localization algorithms can readily be applied to other kinds of depth-encoding PSFs as well.
更多查看译文
关键词
nonconvex optimization algorithms,3D localization,space debris,point spread function,image rotation,image processing
AI 理解论文
溯源树
样例
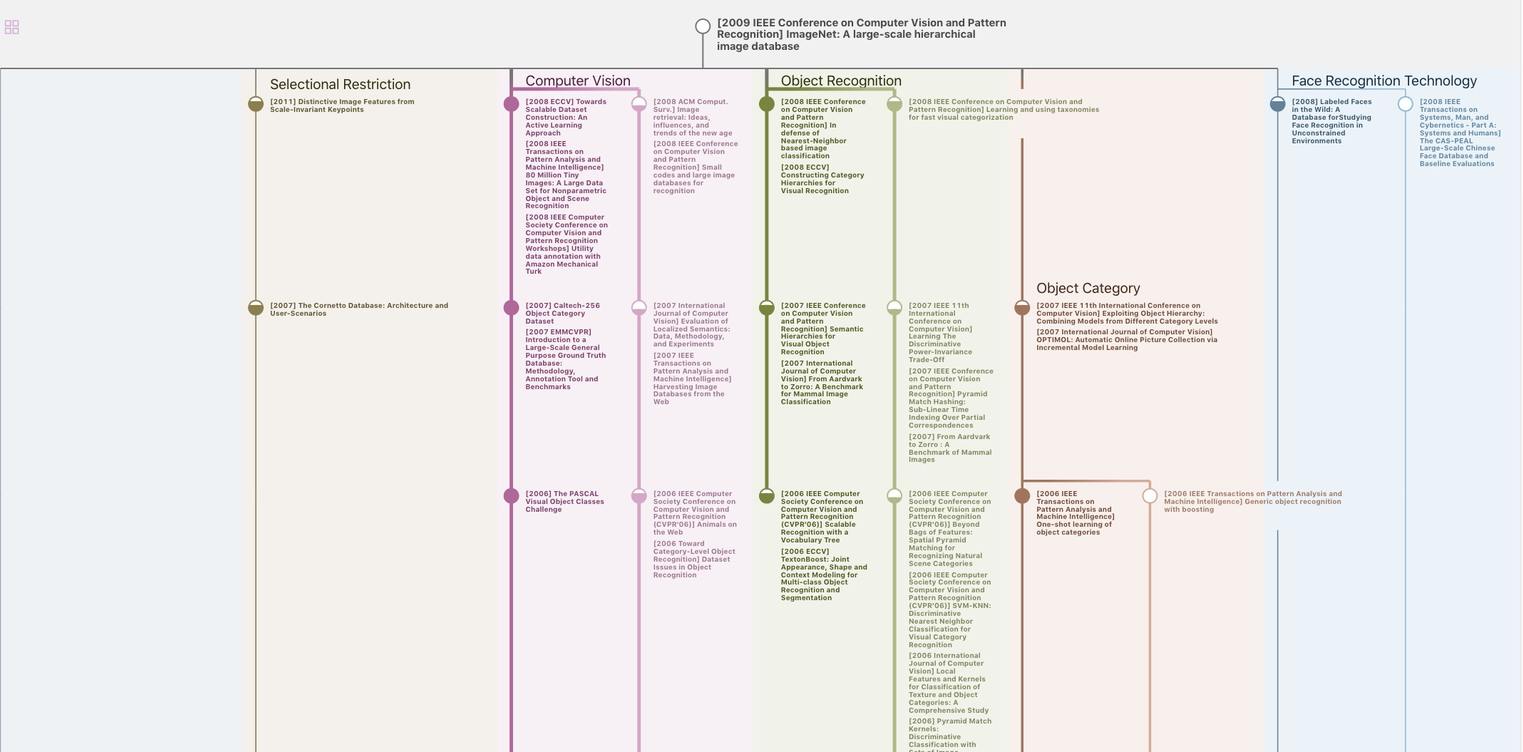
生成溯源树,研究论文发展脉络
Chat Paper
正在生成论文摘要