Designing Neural Networks Using Novel Water Wave Optimization Based Memetic Algorithm
2018 14th International Conference on Natural Computation, Fuzzy Systems and Knowledge Discovery (ICNC-FSKD)(2018)
摘要
Searching neural networks' (NN) optimal parameters can be seen as a multi-modal optimization problem. This paper proposes a memetic water wave optimization algorithm (WWOMA) to determine the optimal weights of NN. In the proposed WWO-based MA (WWOMA), we employ WWO to perform global search by both individual improvement and population co-evolution, and then employ several local search components to enhance its local refinement ability. Furthermore, a Meta-Lamarckian learning strategy is utilized to choose a proper local refinement component to concentrate computational efforts on more promising solutions. We carry out numerical experiments on six well-known NN designing benchmark problems, the empirical results demonstrate both the feasibility and effectiveness by applying WWOMA to design NN.
更多查看译文
关键词
Meta-Lamarckian learning strategy,WWOMA,multimodal optimization problem,memetic water wave optimization algorithm,optimal weights,WWO-based MA,global search,population co-evolution,local search components,local refinement ability,neural network designing,water wave optimization based memetic algorithm,local refinement component
AI 理解论文
溯源树
样例
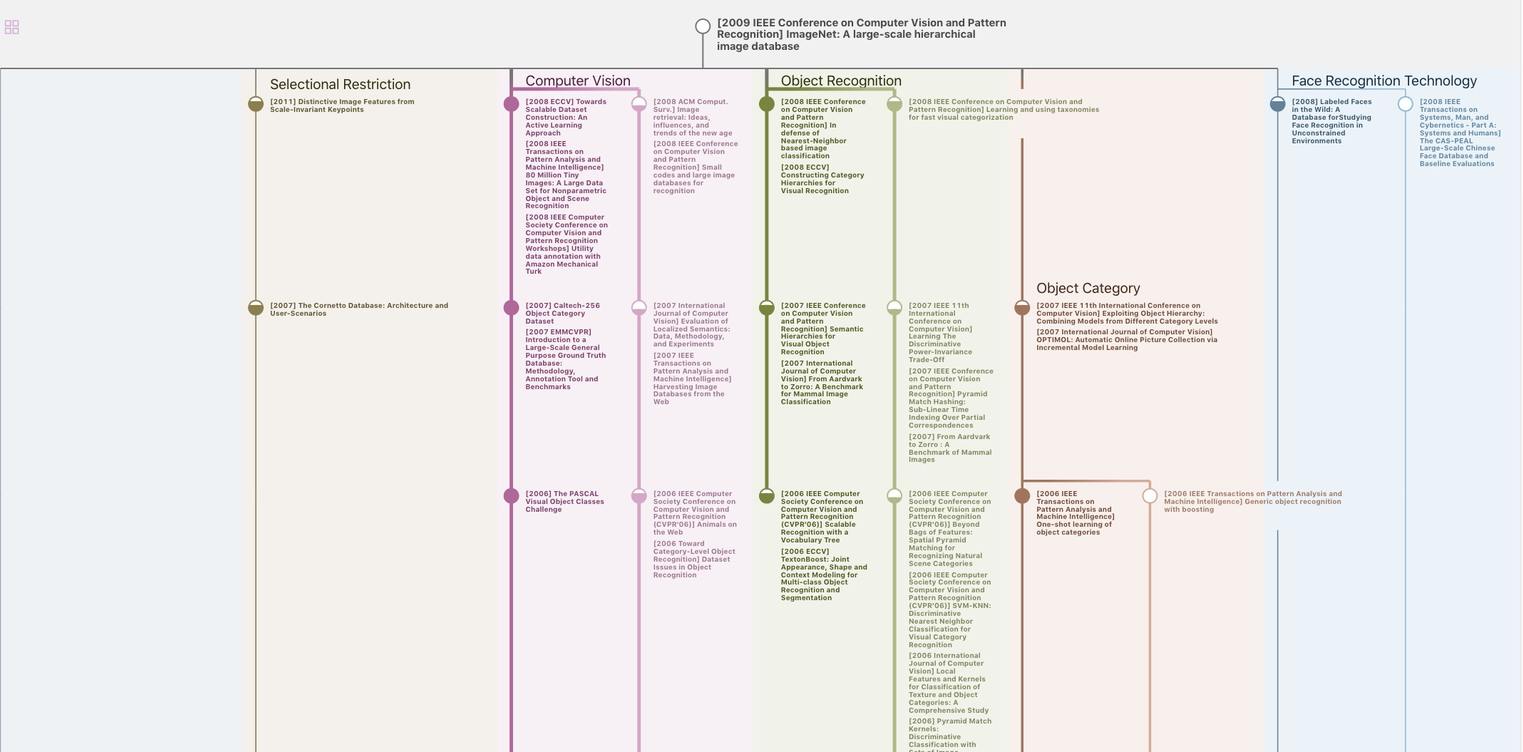
生成溯源树,研究论文发展脉络
Chat Paper
正在生成论文摘要