Learning Binary Codes With Neural Collaborative Filtering For Efficient Recommendation Systems
KNOWLEDGE-BASED SYSTEMS(2019)
摘要
The fast-growing e-commerce scenario brings new challenges to traditional collaborative filtering because the huge amount of users and items requires large storage and efficient recommendation systems. Hence, hashing for collaborative filtering has attracted increasing attention as binary codes can significantly reduce the storage requirement and make similarity calculations efficient. In this paper, we investigate the novel problem of deep collaborative hashing codes on user-item ratings. We propose a new deep learning framework for it, which adopts neural networks to better learn both user and item representations and make these close to binary codes such that the quantization loss is minimized. In addition, we extend the proposed framework for out-of-sample cases, i.e., dealing with new users, new items, and new ratings. Extensive experiments on real-world datasets demonstrate the effectiveness of the proposed framework. (C) 2019 Elsevier B.V. All rights reserved.
更多查看译文
关键词
Recommendation systems,Binary code learning,Neural networks,Neural collaborative hashing
AI 理解论文
溯源树
样例
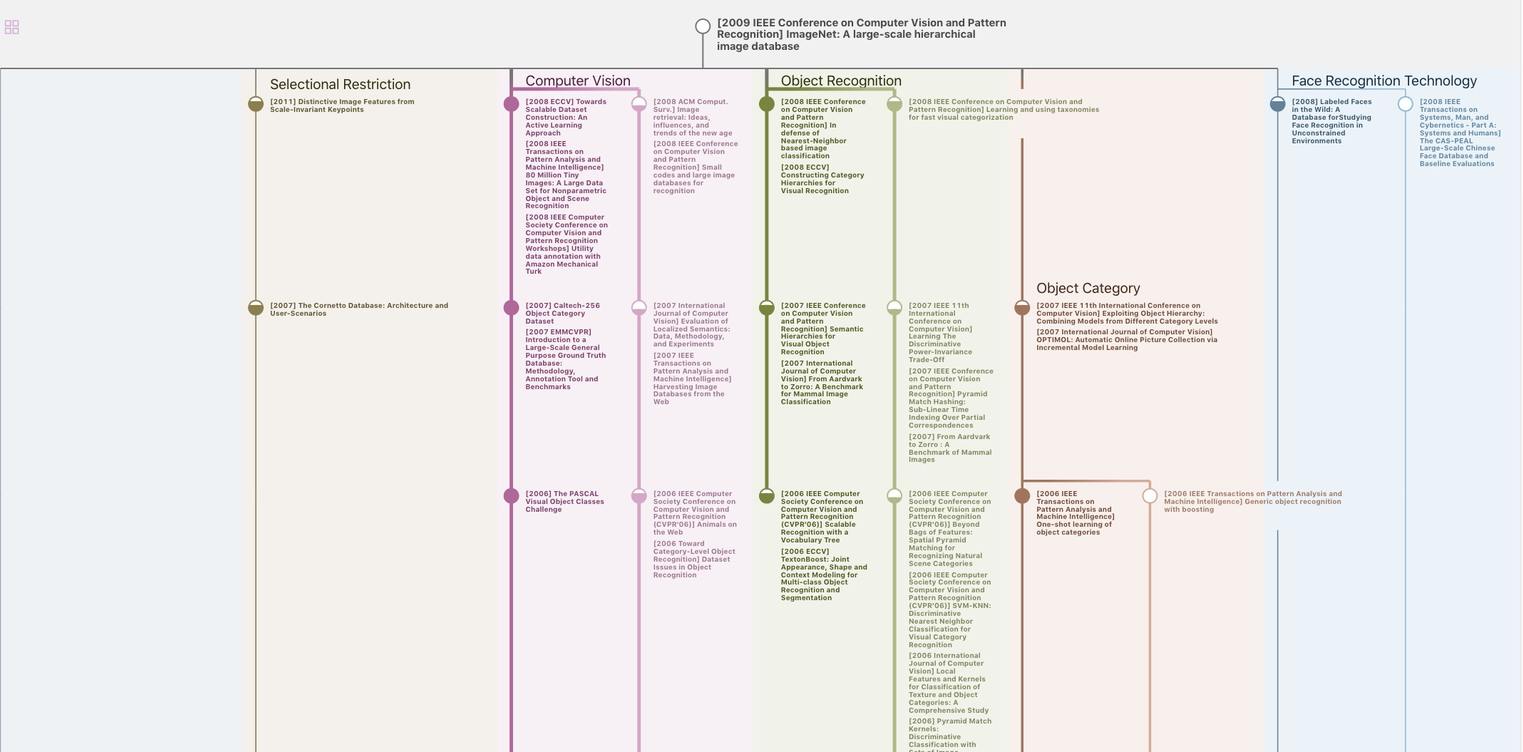
生成溯源树,研究论文发展脉络
Chat Paper
正在生成论文摘要