Artificial intelligence for point of care radiograph quality assessment.
Proceedings of SPIE(2019)
摘要
Chest X-rays are among the most common modalities in medical imaging. Technical flaws of these images, such as over- or under-exposure or wrong positioning of the patients can result in suboptimal images. We propose an automatic method to detect such technical deficiencies. Images with these deficiencies could still be used in the context of a specific diagnostic process. We use a deep neural network trained on a dataset of 3487 images labeled by two experienced radiologists to detect images with technical defects. The DenseNet121 architecture is used for this classification task. The trained network has an area under the receiver operator curve (AUC) of 0.93. By removing the X-rays with technical quality issues, this technology could potentially provide significant cost savings for hospitals.
更多查看译文
关键词
Deep learning,Quality Improvement,Radiograph Reject/Repeat,Radiograph Assessment
AI 理解论文
溯源树
样例
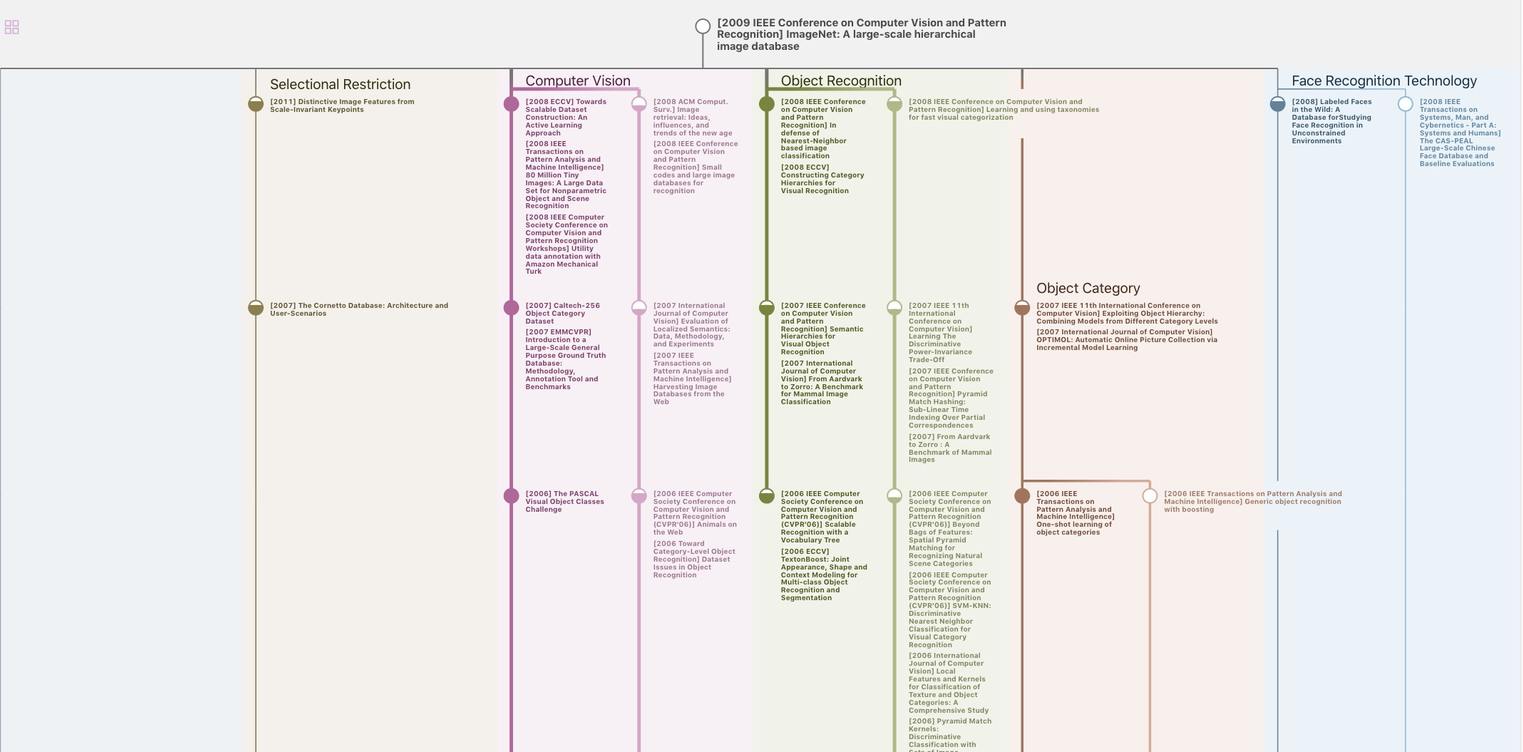
生成溯源树,研究论文发展脉络
Chat Paper
正在生成论文摘要