Bladder cancer staging in CT urography - estimation and validation of decision thresholds for a radiomics-based decision support system.
Proceedings of SPIE(2019)
摘要
Stage T2 is the clinical threshold for deciding whether to treat bladder cancer with neoadjuvant chemotherapy. In this study we refined a radiomics-based decision support system (CDSS-S) to aid clinicians in staging of bladder cancer in CT urography (CTU). To train the CDSS-S, we used a data set of 84 bladder cancers from 76 CTU clinically staged cases, 43 cancers were below stage T2, and 41 were stage T2 or above. An independent test set comprising of 82 bladder cancers from 80 CTU clinically staged cases that were staged as T2 or above were also collected. Our Auto-Initialized Cascaded Level Sets ( AI-CALS) segmentation pipeline was utilized to segment the lesions from which radiomics features were extracted. The training set was split on 2 balanced partitions. Four classifiers were studied: linear discriminant analysis (LDA), support vector machines (SVM), back-propagation neural networks (BPNN), and random forest (RAF) classifiers. Based on the likelihood scores for a training set, the decision threshold providing the highest classification accuracy for each classifier was determined. The classifier with the fixed decision threshold was then applied to the test set and the performance evaluated. The test classification accuracy for the LDA, SVM, BPNN, and RAF trained on Partition 1 was 0.95, 0.98, 0.88, and 0.89, respectively, and was 0.88, 0.94, 0.88, and 0.93, respectively, when trained on Partition 2. The test classification accuracy for the LDA, SVM, BPNN, and RAF trained on the entire training set was 0.94, 0.94, 0.94, and 0.89, respectively. The results show the potential of CDSS-S in bladder cancer stage assessment.
更多查看译文
关键词
Bladder Cancer Staging,Radiomics,Classification,Segmentation
AI 理解论文
溯源树
样例
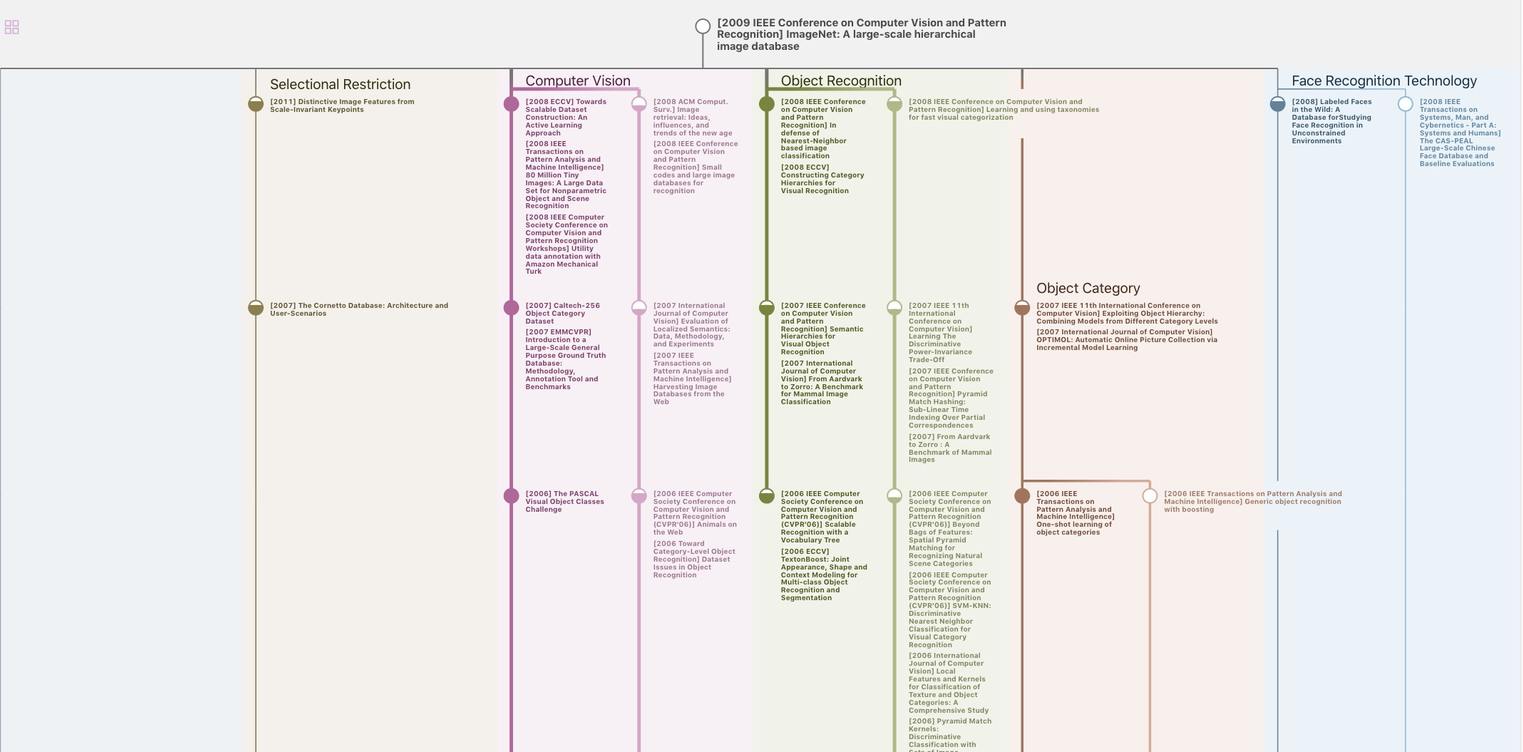
生成溯源树,研究论文发展脉络
Chat Paper
正在生成论文摘要