Implicit Pairs for Boosting Unpaired Image-to-Image Translation.
Visual Informatics(2019)
摘要
In image-to-image translation the goal is to learn a mapping from one image domain to another. In the case of supervised approaches the mapping is learned from paired samples. However, collecting large sets of image pairs is often either prohibitively expensive or not possible. As a result, in recent years more attention has been given to techniques that learn the mapping from unpaired sets.In our work, we show that injecting implicit pairs into unpaired sets strengthens the mapping between the two domains, improves the compatibility of their distributions, and leads to performance boosting of unsupervised techniques by up to 12% across several measurements.The competence of the implicit pairs is further displayed with the use of pseudo-pairs, i.e., paired samples which only approximate a real pair. We demonstrate the effect of the approximated implicit samples on image-to-image translation problems, where such pseudo-pairs may be synthesized in one direction, but not in the other. We further show that pseudo-pairs are significantly more effective as implicit pairs in an unpaired setting, than directly using them explicitly in a paired setting. (C) 2020 The Author(s). Published by Elsevier B.V. on behalf of Zhejiang University and Zhejiang University Press Co. Ltd.
更多查看译文
关键词
Generative adversarial networks, Image-to-image translation, Data augmentation, Synthetic samples
AI 理解论文
溯源树
样例
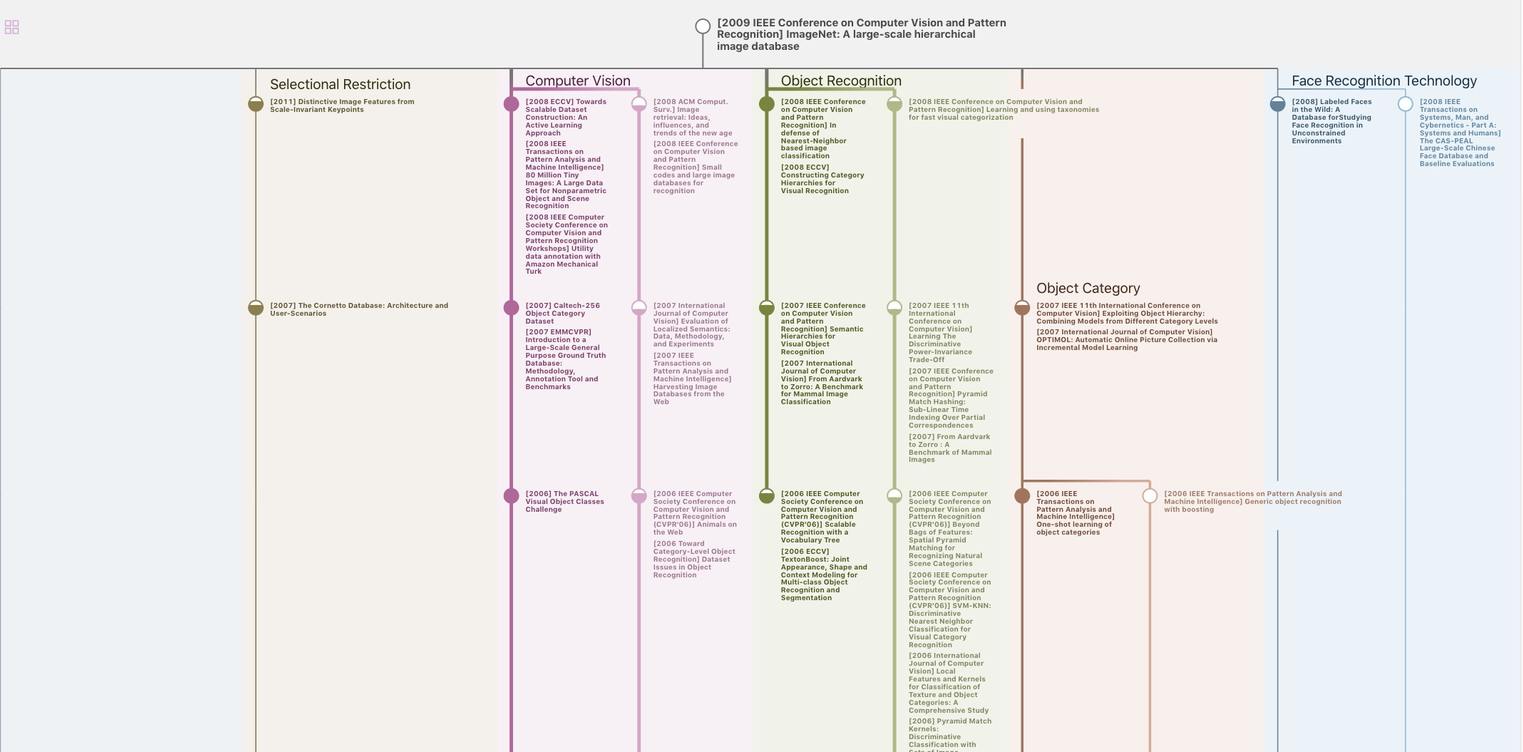
生成溯源树,研究论文发展脉络
Chat Paper
正在生成论文摘要