Intelligent Wide-band Spectrum Classifier
arXiv: Signal Processing(2019)
摘要
We introduce a new technique for narrow-band (NB) signal classification in sparsely populated wide-band (WB) spectrum using supervised learning approach. For WB spectrum acquisition, Nyquist rate sampling is required at the receiveru0027s analog-to-digital converter (ADC), hence we use compressed sensing (CS) theory to alleviate such high rate sampling requirement at the receiver ADC. From the estimated WB spectrum, we then extract various spectral features of each of the NB signal. These features are then used to train and classify each NB signal into its respective modulation using the random forest classifier. In the end, we evaluate the performance of the proposed algorithm under different empirical setups and verify its superior performance in comparison to a recently proposed signal classification algorithm.
更多查看译文
AI 理解论文
溯源树
样例
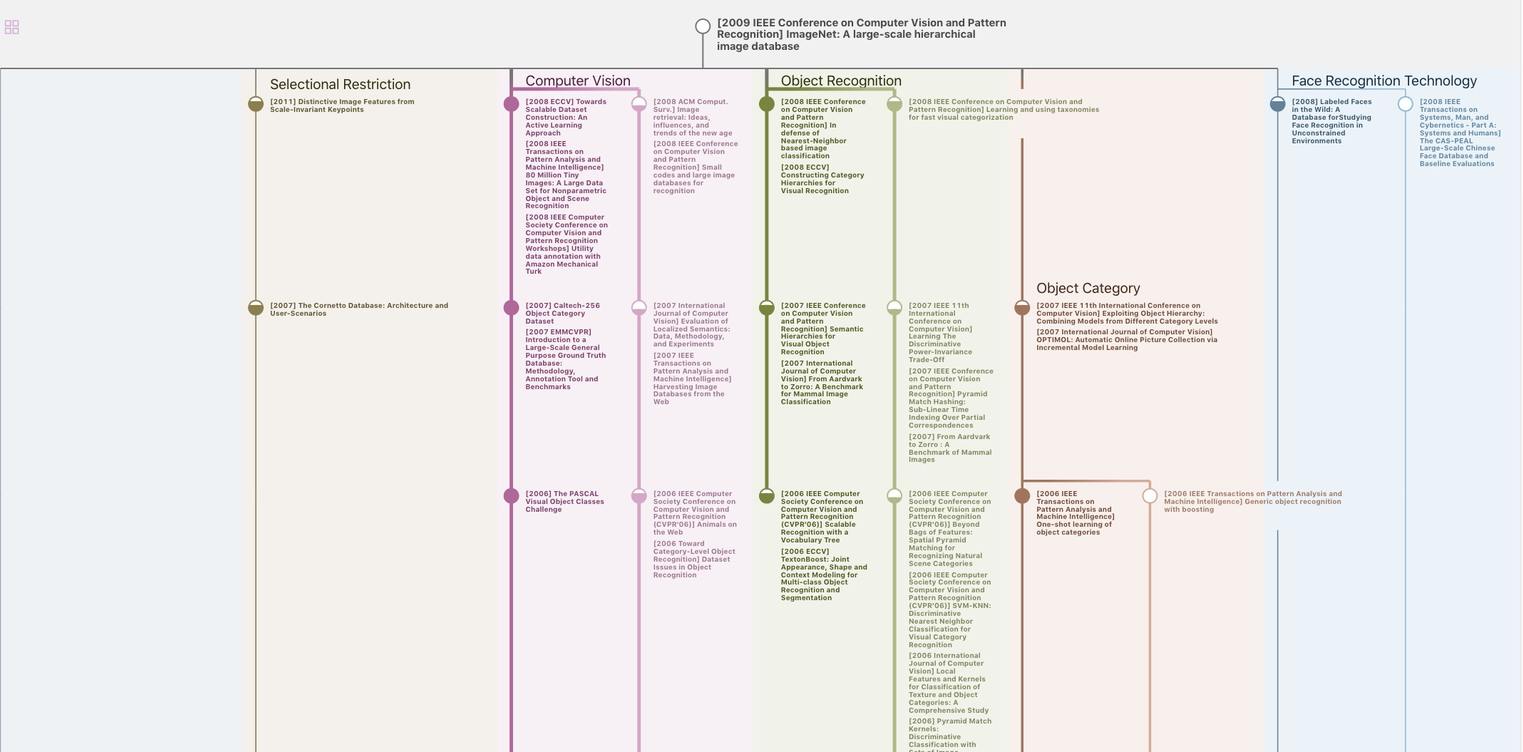
生成溯源树,研究论文发展脉络
Chat Paper
正在生成论文摘要