GP-HD: Using Genetic Programming to Generate Dynamical Systems Models for Health Care
2019 IEEE/WIC/ACM INTERNATIONAL CONFERENCE ON WEB INTELLIGENCE (WI 2019)(2019)
摘要
The huge wealth of data in the health domain can be exploited to create models that predict development of health states over time. Temporal learning algorithms are well suited to learn relationships between health states and make predictions about their future developments. However, these algorithms: (1) either focus on learning one generic model for all patients, providing general insights but often with limited predictive performance, or (2) learn individualized models from which it is hard to derive generic concepts. In this paper, we present a middle ground, namely parameterized dynamical systems models that are generated from data using a Genetic Programming (GP) framework. A fitness function suitable for the health domain is exploited. An evaluation of the approach in the mental health domain shows that performance of the model generated by the GP is on par with a dynamical systems model developed based on domain knowledge, significantly outperforms a generic Long Term Short Term Memory (LSTM) model and in some cases also outperforms an individualized LSTM model.
更多查看译文
关键词
learning (artificial intelligence),social networking (online),text analysis,pattern classification,recommender systems,graph theory,neural nets,Internet,data mining,feature extraction
AI 理解论文
溯源树
样例
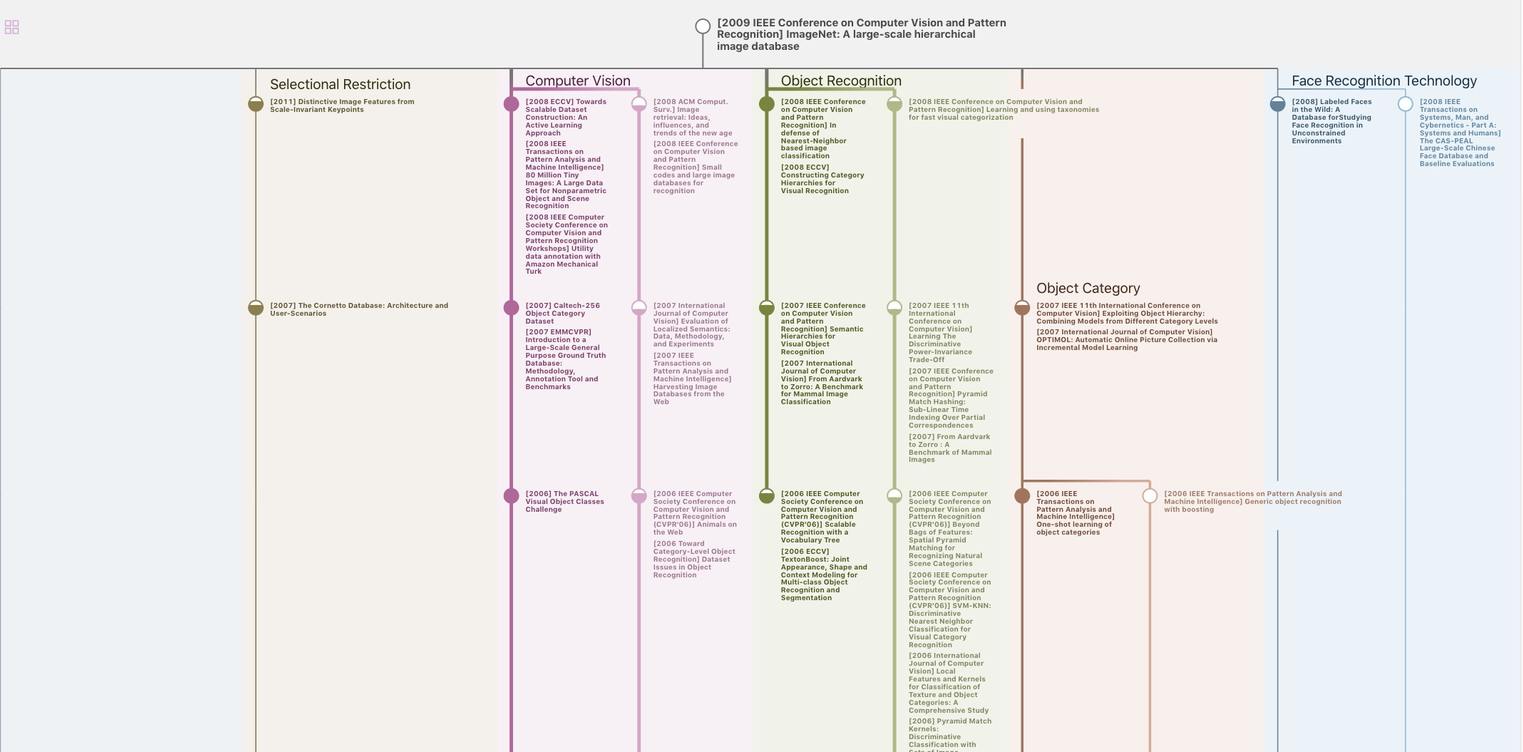
生成溯源树,研究论文发展脉络
Chat Paper
正在生成论文摘要