On The Intrinsic Dimensionality Of Image Representations
2019 IEEE/CVF CONFERENCE ON COMPUTER VISION AND PATTERN RECOGNITION (CVPR 2019)(2019)
摘要
This paper addresses the following questions pertaining to the intrinsic dimensionality of any given image representation: (i) estimate its intrinsic dimensionality, (ii) develop a deep neural network based non-linear mapping, dubbed DeepMDS, that transforms the ambient representation to the minimal intrinsic space, and (iii) validate the veracity of the mapping through image matching in the intrinsic space. Experiments on benchmark image datasets (LFW, IJB-C and ImageNet-100) reveal that the intrinsic dimensionality of deep neural network representations is significantly lower than the dimensionality of the ambient features. For instance, SphereFace's [26] 512-dim face representation and ResNet's [16] 512-dim image representation have an intrinsic dimensionality of 16 and 19 respectively. Further, the DeepMDS mapping is able to obtain a representation of significantly lower dimensionality while maintaining discriminative ability to a large extent, 59.75% TAR @ 0.1% FAR in 16-dim vs 71.26% TAR in 512-dim on IJB-C [29] and a Top-1 accuracy of 77.0% at 19-dim vs 83.4% at 512-dim on ImageNet-100.
更多查看译文
关键词
Representation Learning,Deep Learning , Face,Gesture,and Body Pose , Statistical Learning
AI 理解论文
溯源树
样例
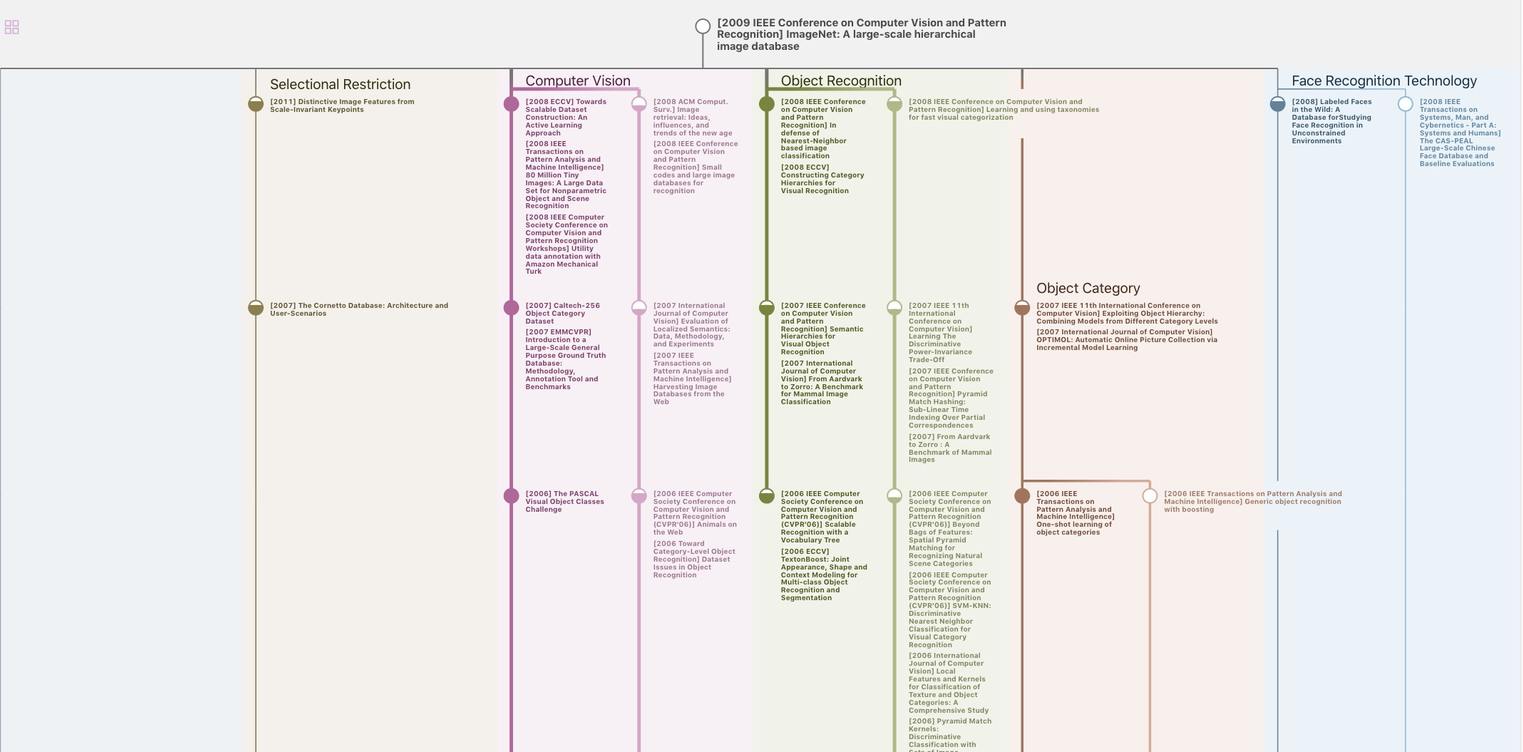
生成溯源树,研究论文发展脉络
Chat Paper
正在生成论文摘要