Tracking Events In Twitter By Combining An Lda-Based Approach And A Density-Contour Clustering Approach
INTERNATIONAL JOURNAL OF SEMANTIC COMPUTING(2019)
摘要
Nowadays, Twitter has become one of the fastest-growing microblogging services; consequently, analyzing this rich and continuously user-generated content can reveal unprecedentedly valuable knowledge. In this paper, we propose a novel two-stage system to detect and track events from tweets by integrating a Latent Dirichlet Allocation (LDA)-based approach and an efficient density-contour-based spatio-temporal clustering approach. In the proposed system, we first divide the geotagged tweet stream into temporal time windows; next, events are identified as topics in tweets using an LDA-based topic discovery step; then, each tweet is assigned an event label; next, a density-contour-based spatio-temporal clustering approach is employed to identify spatio-temporal event clusters. In our approach, topic continuity is established by calculating KL-divergences between topics and spatio-temporal continuity is established by a family of newly formulated spatial cluster distance functions. Moreover, the proposed density-contour clustering approach considers two types of densities: "absolute" density and "relative" density to identify event clusters where either there is a high density of event tweets or there is a high percentage of event tweets. We evaluate our approach using real-world data collected from Twitter, and the experimental results show that the proposed system can not only detect and track events effectively but also discover interesting patterns from geotagged tweets.
更多查看译文
关键词
Natural language processing, latent Dirichlet allocation, machine learning, density-contour-based clustering, spatio-temporal clustering
AI 理解论文
溯源树
样例
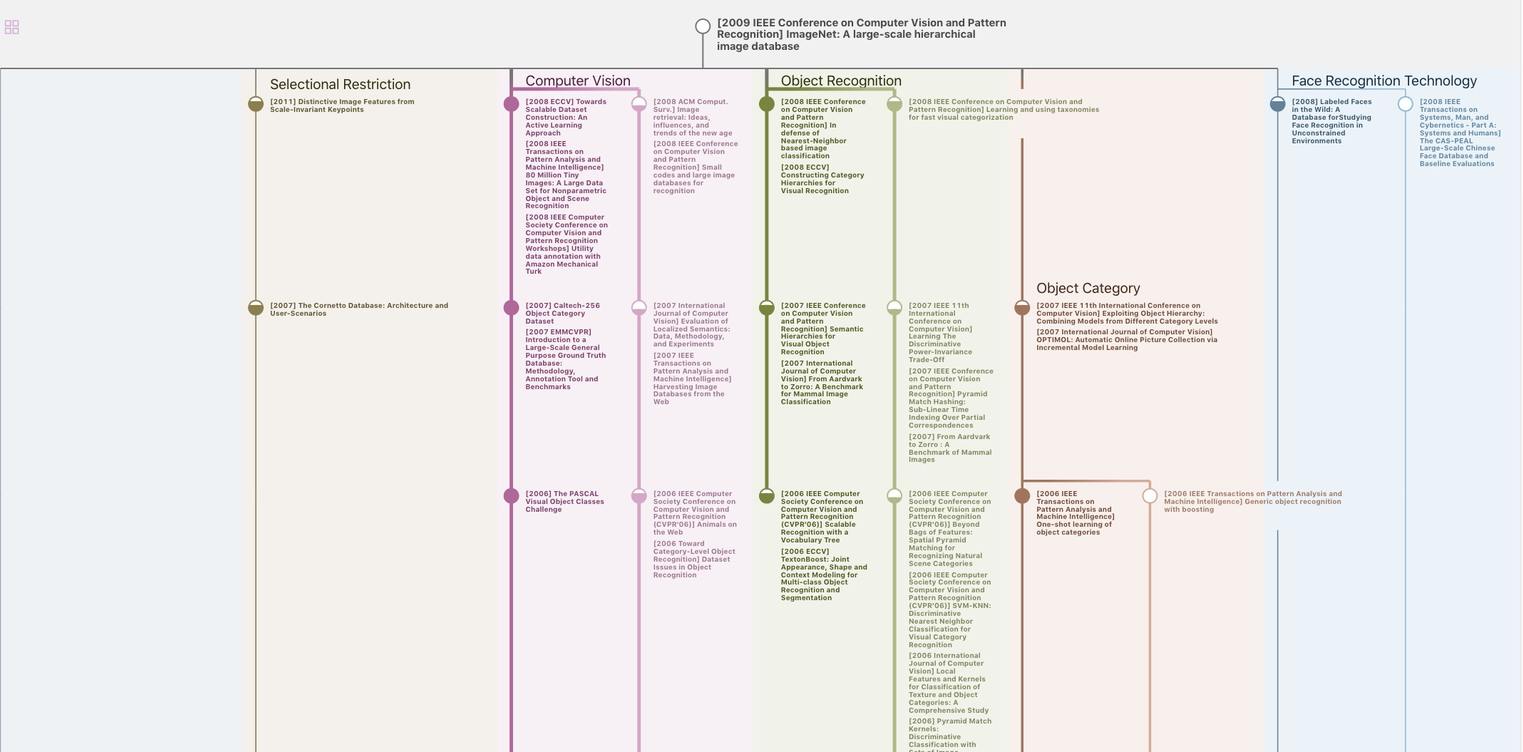
生成溯源树,研究论文发展脉络
Chat Paper
正在生成论文摘要