Relational Knowledge Distillation
2019 IEEE/CVF CONFERENCE ON COMPUTER VISION AND PATTERN RECOGNITION (CVPR 2019)(2019)
摘要
Knowledge distillation aims at transferring knowledge acquired in one model (a teacher) to another model (a student) that is typically smaller. Previous approaches can be expressed as a form of training the student to mimic output activations of individual data examples represented by the teacher. We introduce a novel approach, dubbed relational knowledge distillation (RKD), that transfers mutual relations of data examples instead. For concrete realizations of RKD, we propose distance-wise and angle-wise distillation losses that penalize structural differences in relations. Experiments conducted on different tasks show that the proposed method improves educated student models with a significant margin. In particular for metric learning, it allows students to outperform their teachers' performance, achieving the state of the arts on standard benchmark datasets.
更多查看译文
关键词
Representation Learning,Deep Learning,Recognition: Detection,Categorization,Retrieval
AI 理解论文
溯源树
样例
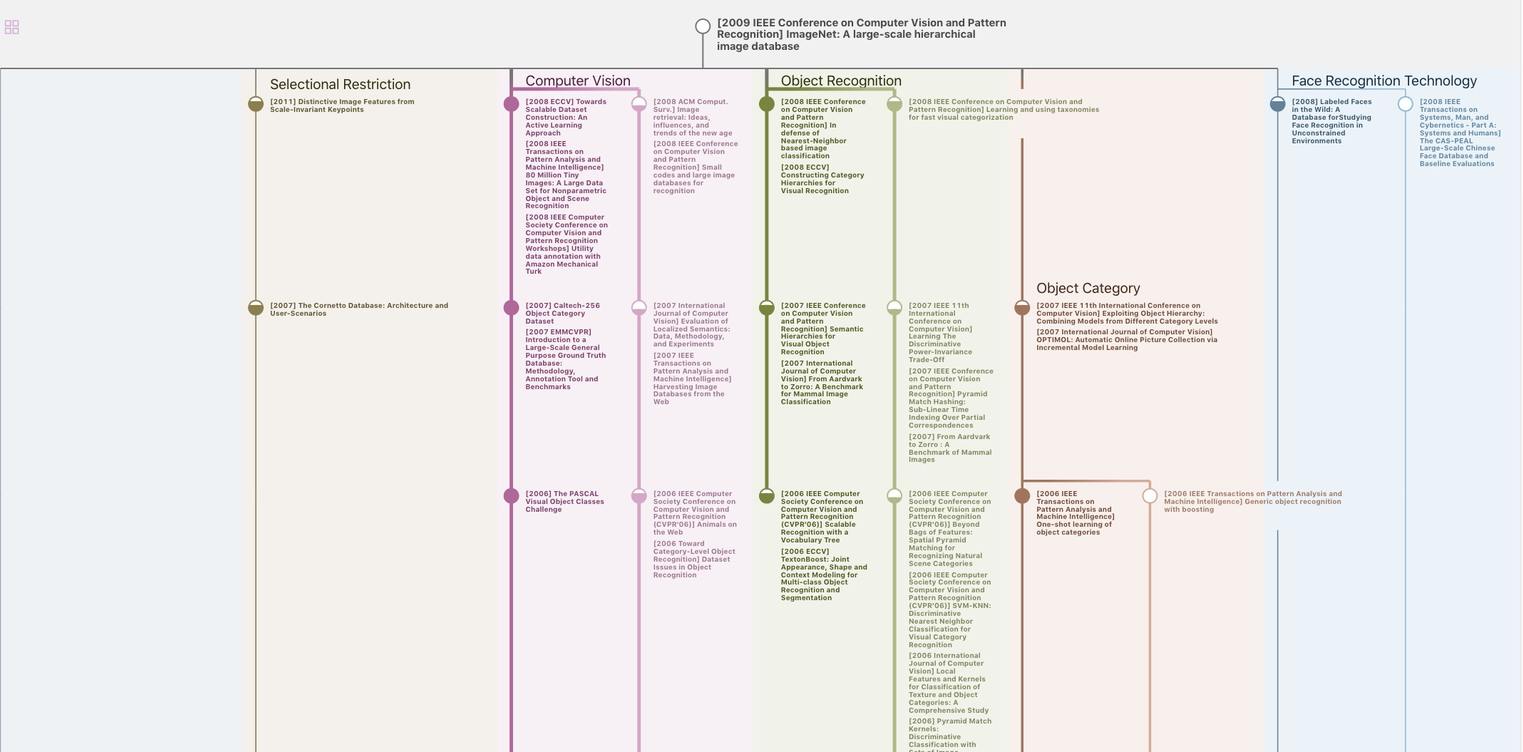
生成溯源树,研究论文发展脉络
Chat Paper
正在生成论文摘要