Improving NeuroEvolution Efficiency by Surrogate Model-Based Optimization with Phenotypic Distance Kernels.
EvoApplications(2019)
摘要
In NeuroEvolution, the topologies of artificial neural networks are optimized with evolutionary algorithms to solve tasks in data regression, data classification, or reinforcement learning. One downside of NeuroEvolution is the large amount of necessary fitness evaluations, which might render it inefficient for tasks with expensive evaluations, such as real-time learning. For these expensive optimization tasks, surrogate model-based optimization is frequently applied as it features a good evaluation efficiency. While a combination of both procedures appears as a valuable solution, the definition of adequate distance measures for the surrogate modeling process is difficult. In this study, we will extend cartesian genetic programming of artificial neural networks by the use of surrogate model-based optimization. We propose different distance measures and test our algorithm on a replicable benchmark task. The results indicate that we can significantly increase the evaluation efficiency and that a phenotypic distance, which is based on the behavior of the associated neural networks, is most promising.
更多查看译文
关键词
neuroevolution efficiency,phenotypic distance kernels,optimization,model-based
AI 理解论文
溯源树
样例
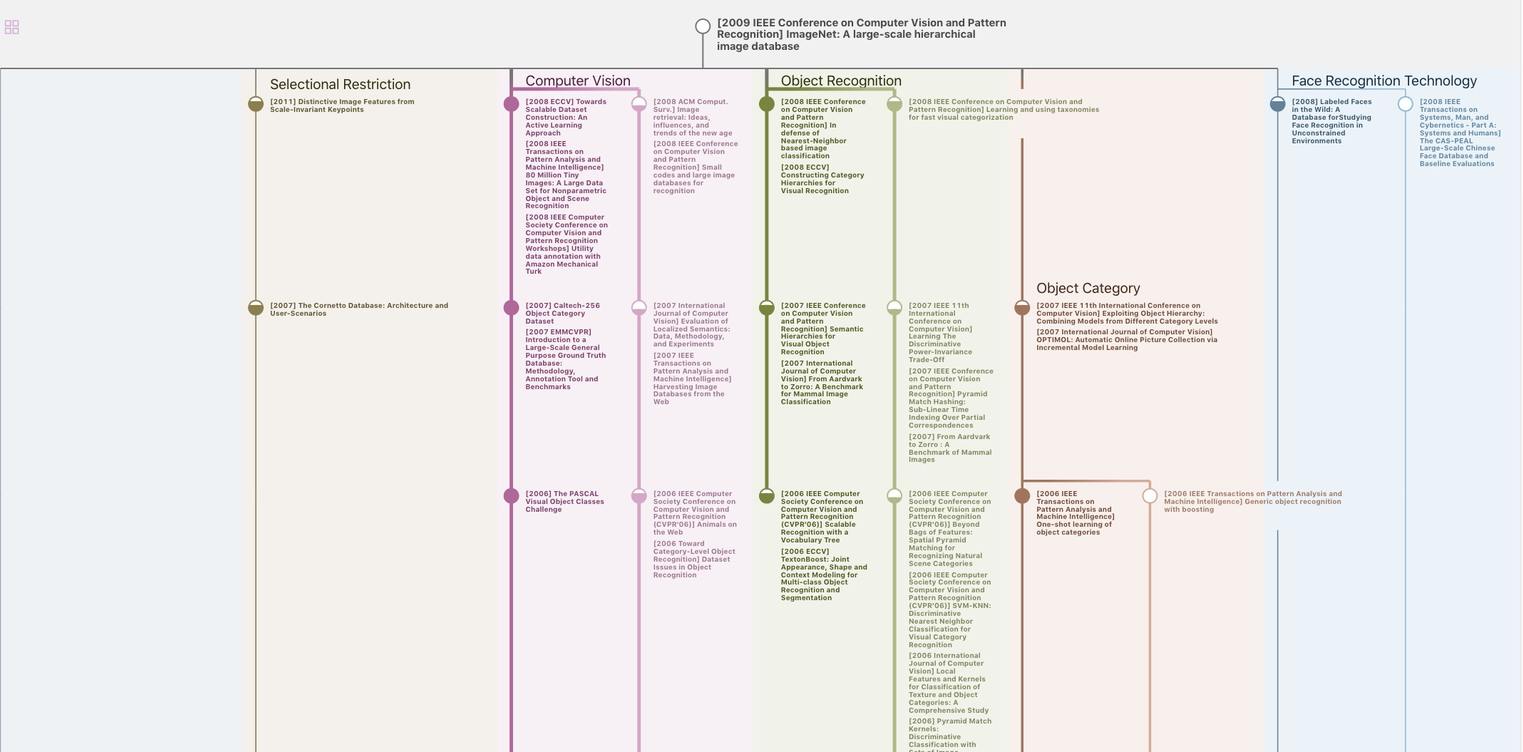
生成溯源树,研究论文发展脉络
Chat Paper
正在生成论文摘要