Electric Load Forecasting with Hourly Precision Using Long Short-Term Memory Networks
2019 International Conference on Electrical, Computer and Communication Engineering (ECCE)(2019)
摘要
Electric load forecasting is of foremost importance to plan for the future power generation and consumption of any country. To meet increasing demand and keep up with the growing economy, it is becoming increasingly challenging for the government of Bangladesh to operate and perform maintenance on its power system. Bangladesh Power System (BPS) has been operated primarily based on a trial and error method of load forecasting. In this paper, we propose an approach for the short term electric load forecasting by employing a recurrent neural network (RNN) architecture called Long Short-Term Memory (LSTM). Using this proposed approach, we forecast the electric load of one hour ahead with minimal error. Before a time series is fed into artificial neural networks (ANN), it must be devoid of seasonality and trend to get acceptable predictions. This is especially true for load demand of Bangladesh which shows sharp periodical peaks as well as a general trend of yearly increase. Therefore, we present a method of applying LSTM with data construction method to remove seasonality and trend from load time series of BPS for hourly electric load forecasting.
更多查看译文
关键词
Time series analysis,Logic gates,Forecasting,Load forecasting,Computer architecture,Neural networks,Market research
AI 理解论文
溯源树
样例
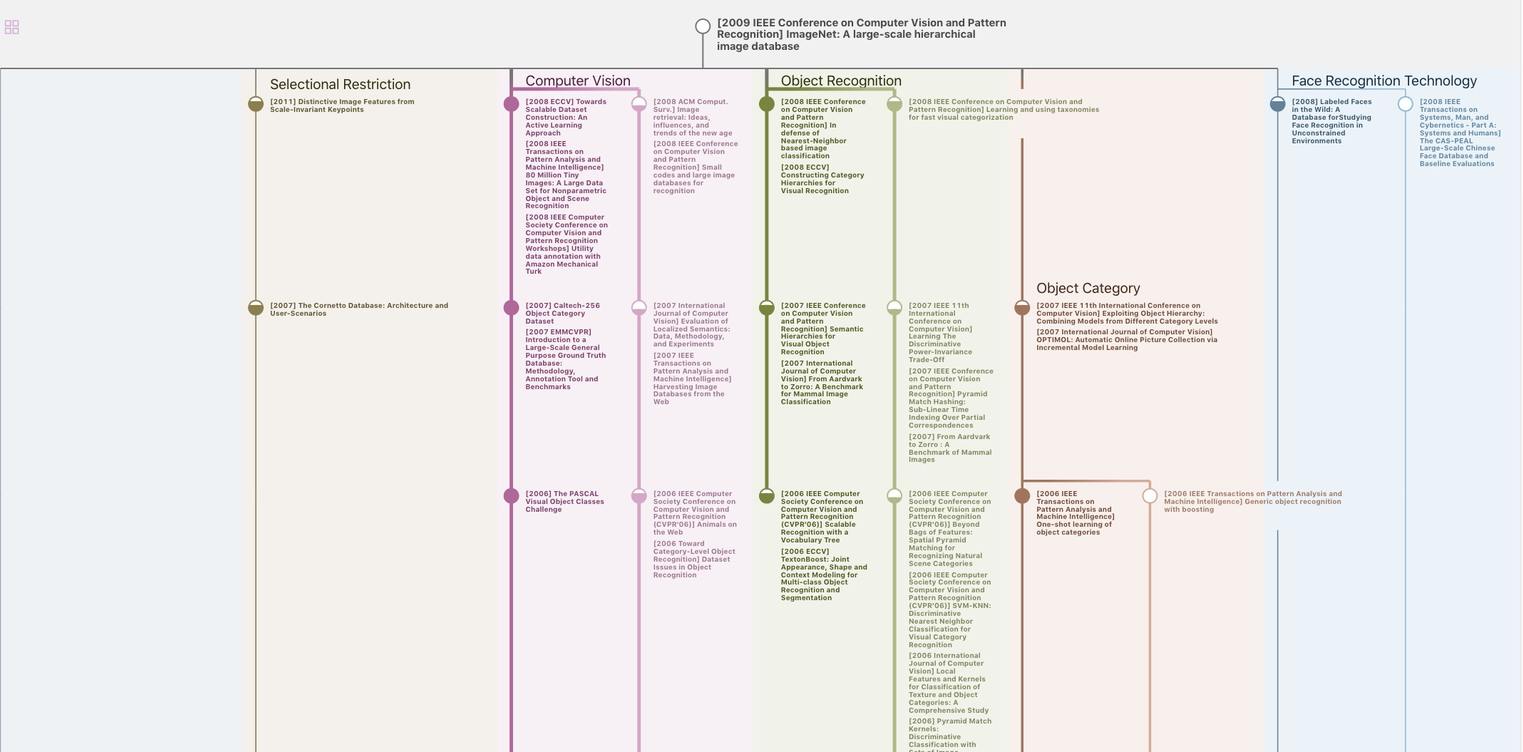
生成溯源树,研究论文发展脉络
Chat Paper
正在生成论文摘要