A Randomized Coordinate Descent Method with Volume Sampling
SIAM JOURNAL ON OPTIMIZATION(2019)
摘要
We analyze the coordinate descent method with a new coordinate selection strategy, called volume sampling. This strategy prescribes selecting subsets of variables of certain size proportionally to the determinants of principal submatrices of the matrix, that bounds the curvature of the objective function. In the particular case, when the size of the subsets equals one, volume sampling coincides with the well-known strategy of sampling coordinates proportionally to their Lipschitz constants. For the coordinate descent with volume sampling, we establish the convergence rates both for convex and strongly convex problems. Our theoretical results show that, by increasing the size of the subsets, it is possible to accelerate the method up to the factor which depends on the spectral gap between the corresponding largest eigenvalues of the curvature matrix. Several numerical experiments confirm our theoretical conclusions.
更多查看译文
关键词
convex optimization, unconstrained minimization, coordinate descent methods, randomized algorithms, volume sampling, convergence rate
AI 理解论文
溯源树
样例
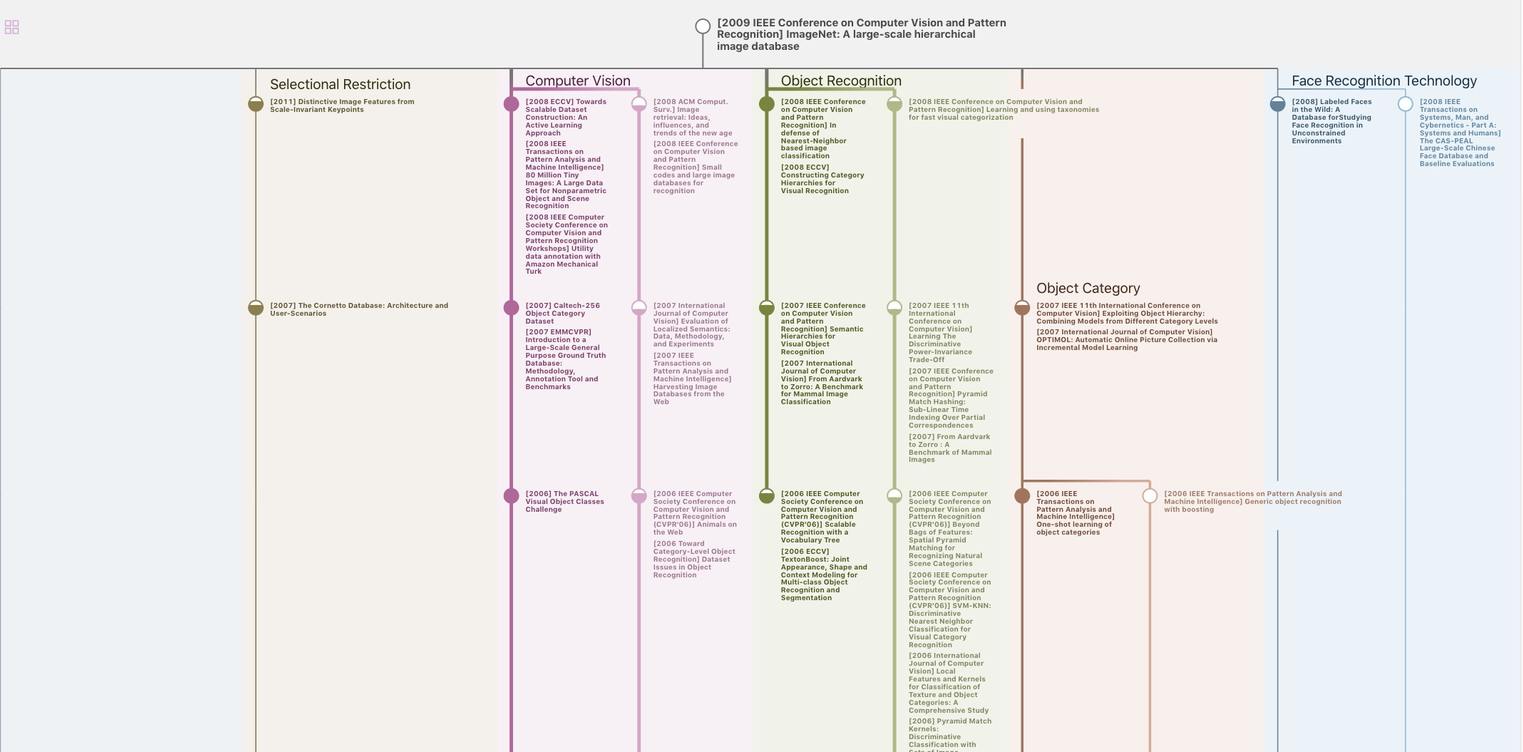
生成溯源树,研究论文发展脉络
Chat Paper
正在生成论文摘要