Snowball: Extracting Causal Chains from Climate Change Text Corpora
2018 1st International Conference on Data Intelligence and Security (ICDIS)(2018)
摘要
Unpacking causal relationships is essential for developing solutions for managing climate risks that threaten sociopolitical stability. However, the automatic discovery of complex causal chains among interlinked events and their participating actors within large corpora is not well studied. Previous studies on extracting causal relationships from text were based on laborious and incomplete hand developed lists of causal verbs, such as "causes" and "results in". Such approaches result in limited recall because standard causal verbs may not generalize well to accommodate surface variations in texts when different keywords and phrases are used to express similar causal effects. This paper presents a Snowball system to generalize triplets extracted from corpora of online news articles, and cluster them into higher-level concepts without drift. We start with a seed set of causal verbs and apply a concept generalization technique to extract causal chains and their participating actors. Our novel algorithms overcome surface variations in written expressions of causal relationships and discover the domino effects between climate events and human security. Unlike prior studies, our semi-supervised approach alleviates the need for labor intensive keyword list development and annotated datasets. Experimental evaluations by domain experts achieve an average precision of 82%, a significant improvement from prior work. Qualitative assessments of causal chains show that results are consistent with the 2014 IPCC report illuminating causal mechanisms underlying the linkages between climatic stresses and social instability.
更多查看译文
关键词
Climate Change,Causal Relations,Causal Chains,Text Mining,Information Extraction,Natural Language Processing
AI 理解论文
溯源树
样例
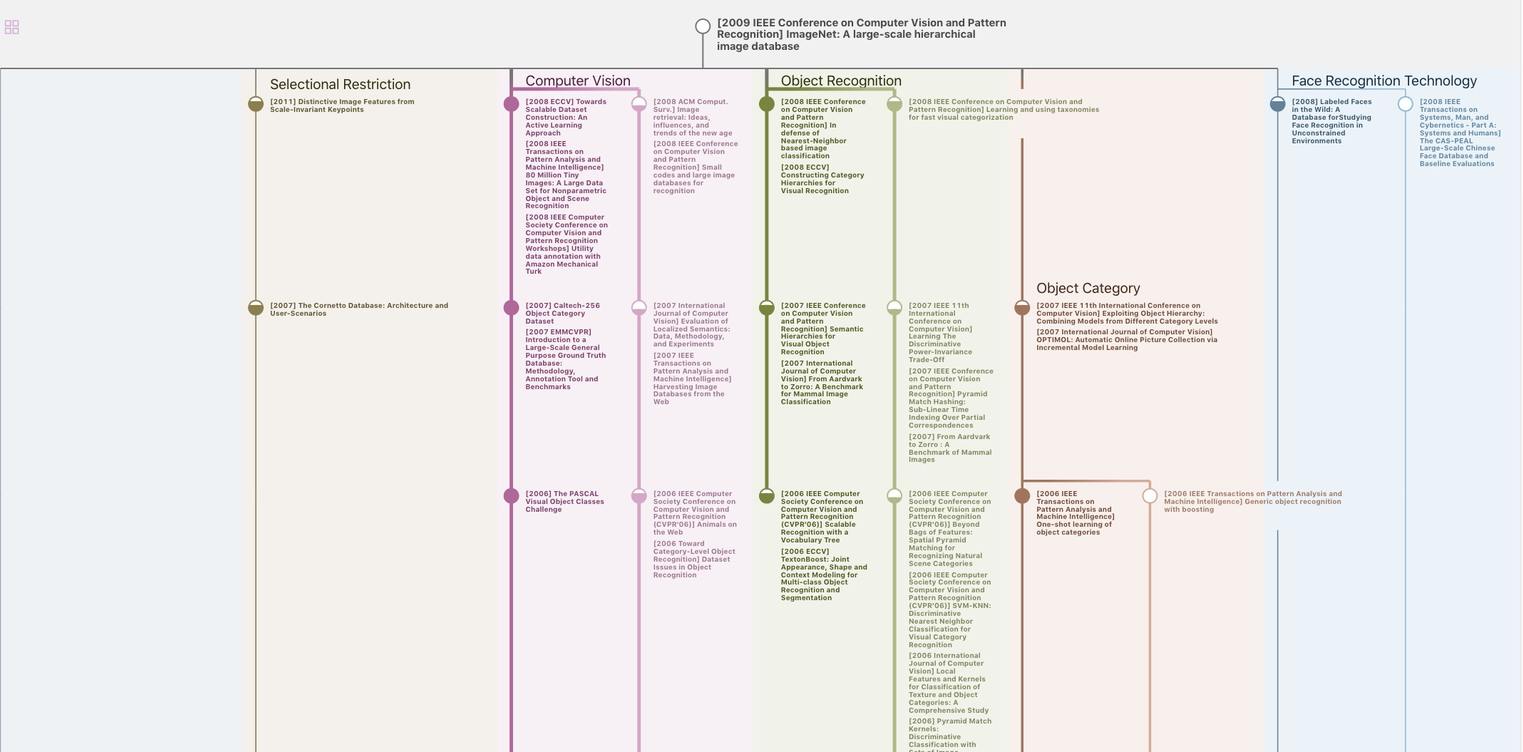
生成溯源树,研究论文发展脉络
Chat Paper
正在生成论文摘要