Compressed k NN: K-Nearest Neighbors with Data Compression.
Entropy (Basel, Switzerland)(2019)
摘要
The NN (k-nearest neighbors) classification algorithm is one of the most widely used non-parametric classification methods, however it is limited due to memory consumption related to the size of the dataset, which makes them impractical to apply to large volumes of data. Variations of this method have been proposed, such as condensed KNN which divides the training dataset into clusters to be classified, other variations reduce the input dataset in order to apply the algorithm. This paper presents a variation of the NN algorithm, of the type structure less NN, to work with categorical data. Categorical data, due to their nature, can be compressed in order to decrease the memory requirements at the time of executing the classification. The method proposes a previous phase of compression of the data to then apply the algorithm on the compressed data. This allows us to maintain the whole dataset in memory which leads to a considerable reduction of the amount of memory required. Experiments and tests carried out on known datasets show the reduction in the volume of information stored in memory and maintain the accuracy of the classification. They also show a slight decrease in processing time because the information is decompressed in real time (on-the-fly) while the algorithm is running.
更多查看译文
关键词
KNN,categorical data,classification,compression,feature pre-processing
AI 理解论文
溯源树
样例
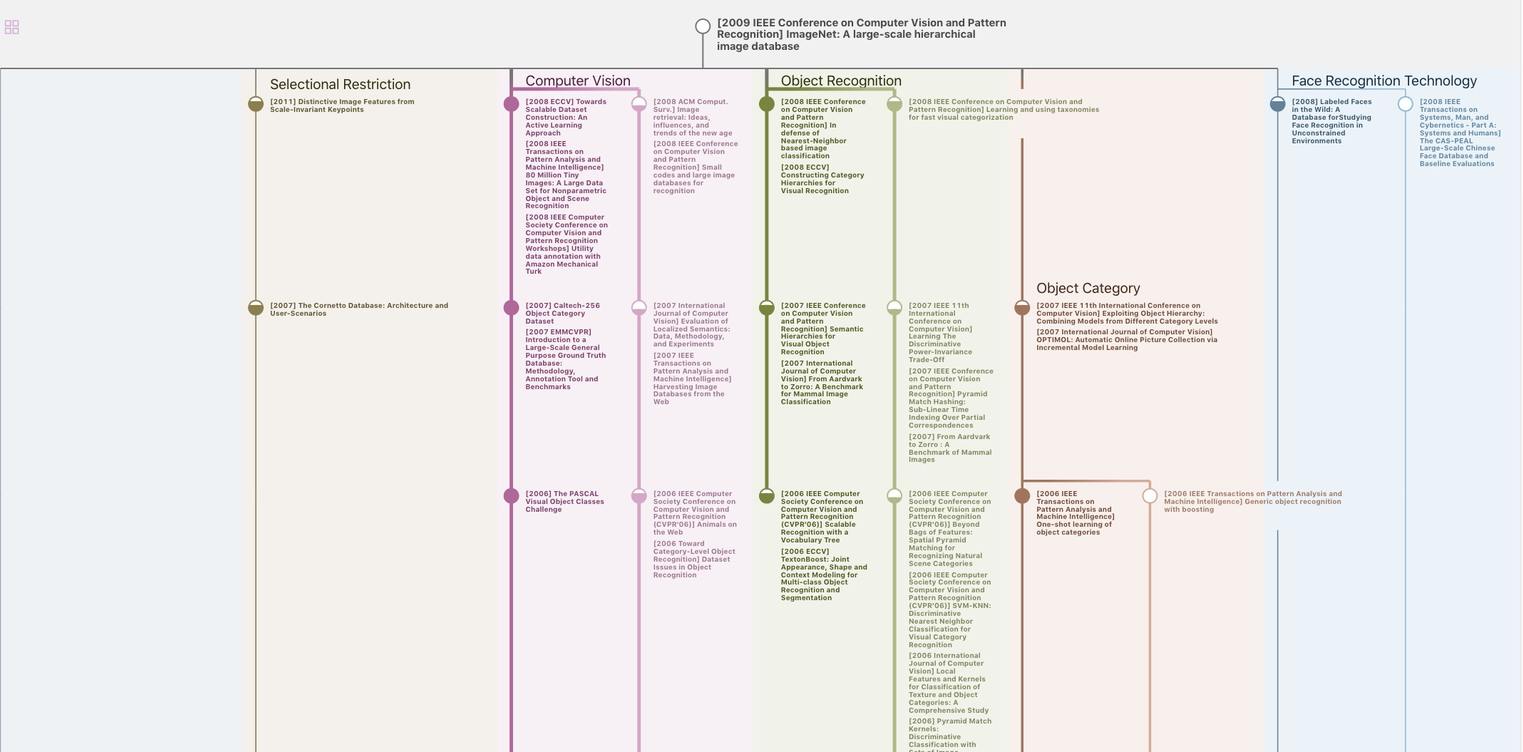
生成溯源树,研究论文发展脉络
Chat Paper
正在生成论文摘要