Speech Model Pre-Training for End-to-End Spoken Language Understanding.
INTERSPEECH(2019)
摘要
Whereas conventional spoken language understanding (SLU) systems map speech to text, and then text to intent, end-to-end SLU systems map speech directly to intent through a single trainable model. Achieving high accuracy with these end-to-end models without a large amount of training data is difficult. We propose a method to reduce the data requirements of end-to-end SLU in which the model is first pre-trained to predict words and phonemes, thus learning good features for SLU. We introduce a new SLU dataset, Fluent Speech Commands, and show that our method improves performance both when the full dataset is used for training and when only a small subset is used. We also describe preliminary experiments to gauge the model's ability to generalize to new phrases not heard during training.
更多查看译文
关键词
speech recognition, spoken language understanding, end-to-end models, transfer learning
AI 理解论文
溯源树
样例
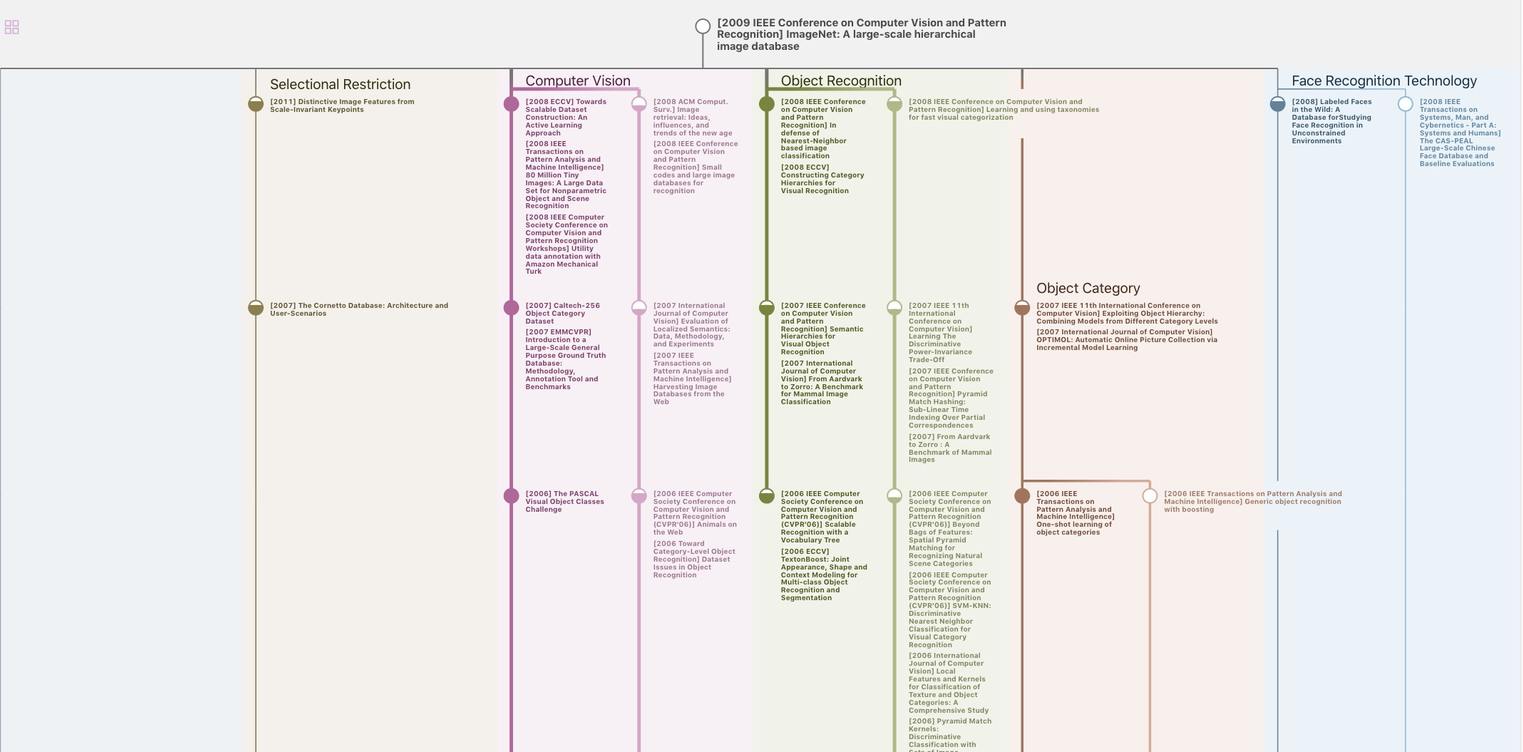
生成溯源树,研究论文发展脉络
Chat Paper
正在生成论文摘要