Memorizing Normality To Detect Anomaly: Memory-Augmented Deep Autoencoder For Unsupervised Anomaly Detection
2019 IEEE/CVF INTERNATIONAL CONFERENCE ON COMPUTER VISION (ICCV 2019)(2019)
摘要
Deep autoencoder has been extensively used for anomaly detection. Training on the normal data, the autoencoder is expected to produce higher reconstruction error for the abnormal inputs than the normal ones, which is adopted as a criterion for identifying anomalies. However, this assumption does not always hold in practice. It has been observed that sometimes the autoencoder "generalizes" so well that it can also reconstruct anomalies well, leading to the miss detection of anomalies. To mitigate this drawback for autoencoder based anomaly detector, we propose to augment the autoencoder with a memory module and develop an improved autoencoder called memory-augmented autoencoder, i.e. MemAE. Given an input, MemAE firstly obtains the encoding from the encoder and then uses it as a query to retrieve the most relevant memory items for reconstruction. At the training stage, the memory contents are updated and are encouraged to represent the prototypical elements of the normal data. At the test stage, the learned memory will be fixed, and the reconstruction is obtained from a few selected memory records of the normal data. The reconstruction will thus tend to be close to a normal sample. Thus the reconstructed errors on anomalies will be strengthened for anomaly detection. MemAE is free of assumptions on the data type and thus general to be applied to different tasks. Experiments on various datasets prove the excellent generalization and high effectiveness of the proposed MemAE.
更多查看译文
关键词
memory-augmented deep autoencoder,unsupervised anomaly detection,reconstruction error,anomaly identification,memory module,memory-augmented autoencoder,MemAE,relevant memory items,memory contents,learned memory,normality memorization,memory records,generalization,autoencoder based anomaly detector
AI 理解论文
溯源树
样例
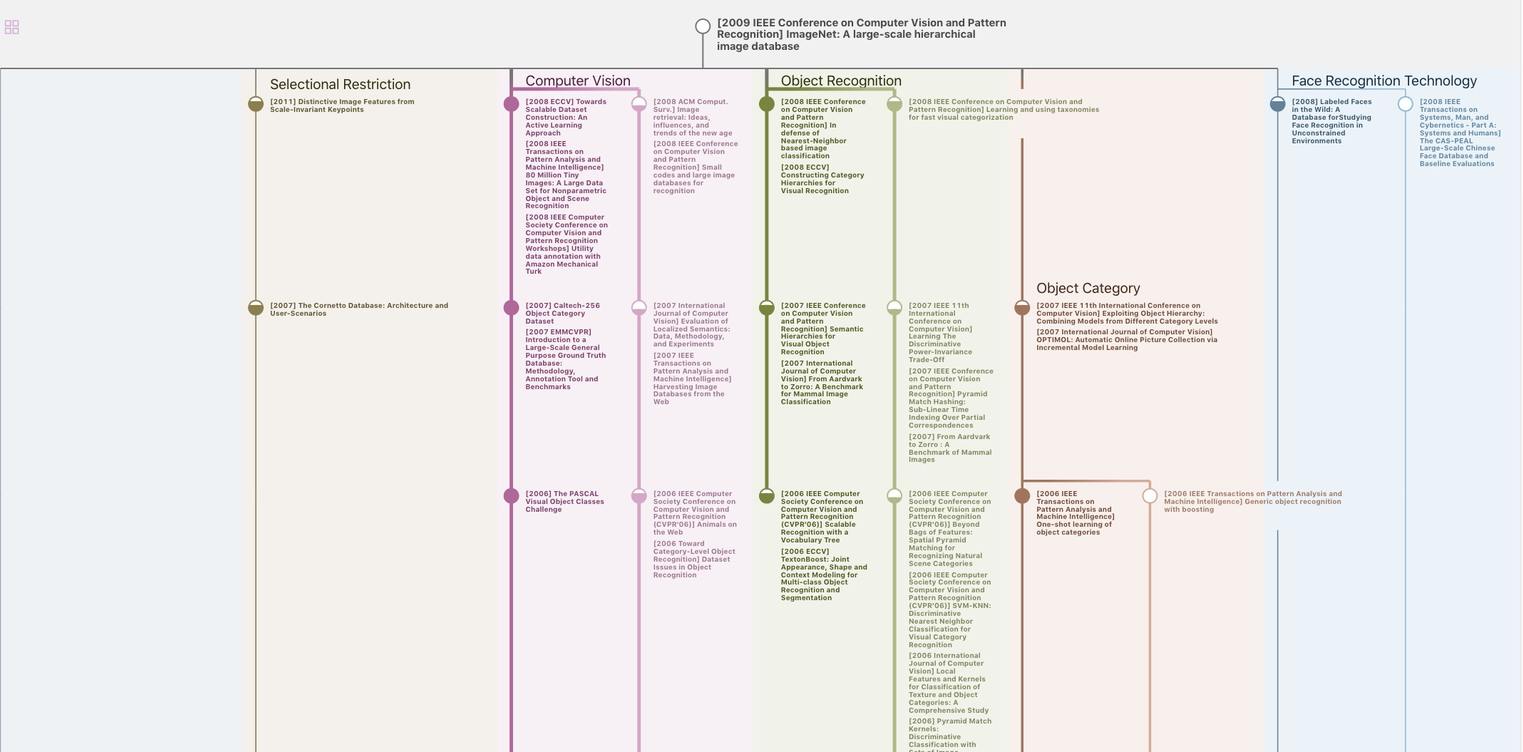
生成溯源树,研究论文发展脉络
Chat Paper
正在生成论文摘要