Multivariate outlier detection based on a robust Mahalanobis distance with shrinkage estimators
Statistical Papers(2019)
摘要
A collection of robust Mahalanobis distances for multivariate outlier detection is proposed, based on the notion of shrinkage. Robust intensity and scaling factors are optimally estimated to define the shrinkage. Some properties are investigated, such as affine equivariance and breakdown value. The performance of the proposal is illustrated through the comparison to other techniques from the literature, in a simulation study and with a real dataset. The behavior when the underlying distribution is heavy-tailed or skewed, shows the appropriateness of the method when we deviate from the common assumption of normality. The resulting high true positive rates and low false positive rates in the vast majority of cases, as well as the significantly smaller computation time show the advantages of our proposal.
更多查看译文
关键词
Multivariate \documentclass[12pt]{minimal}
\usepackage{amsmath}
\usepackage{wasysym}
\usepackage{amsfonts}
\usepackage{amssymb}
\usepackage{amsbsy}
\usepackage{mathrsfs}
\usepackage{upgreek}
\setlength{\oddsidemargin}{-69pt}
\begin{document}$$L_1$$\end{document} -median
AI 理解论文
溯源树
样例
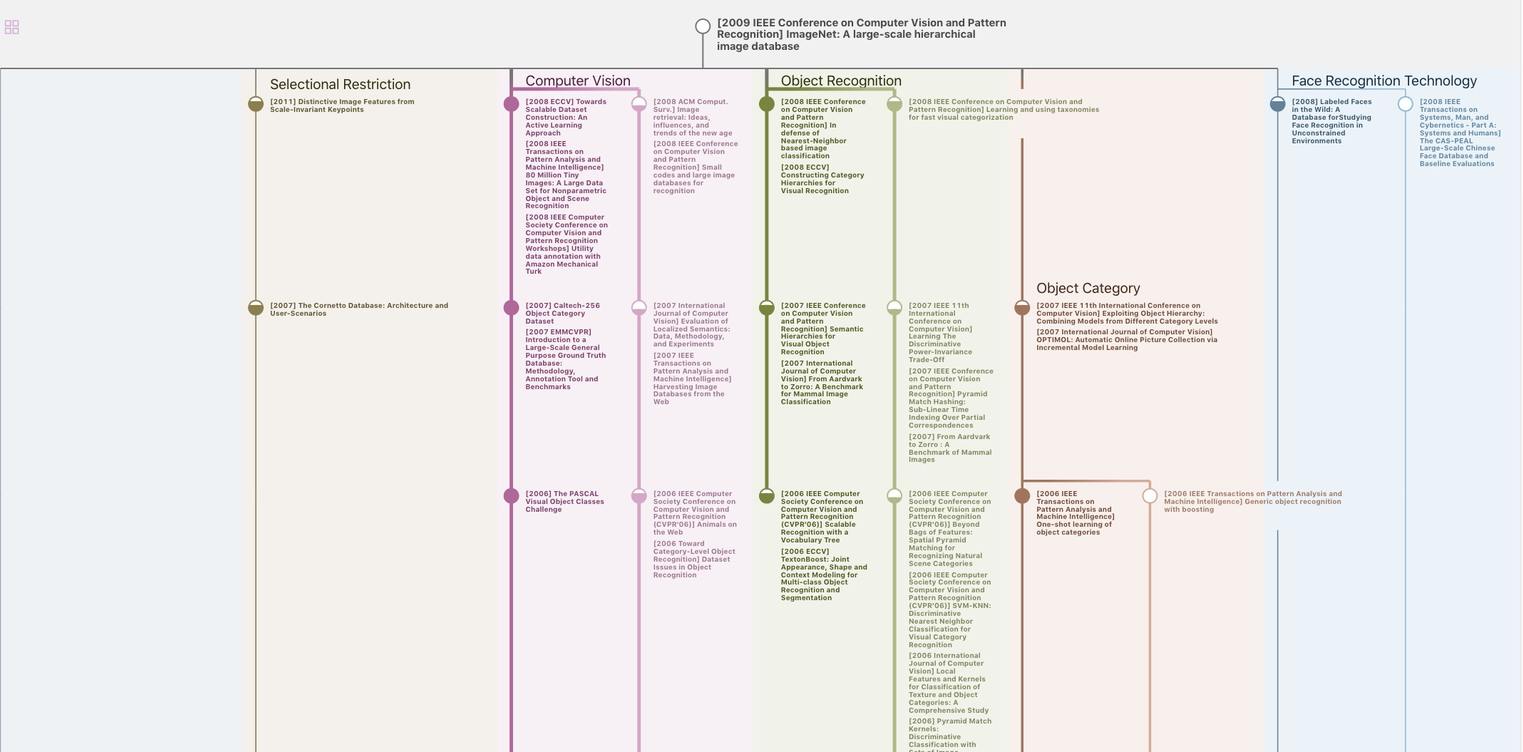
生成溯源树,研究论文发展脉络
Chat Paper
正在生成论文摘要