Conditional Adversarial Generative Flow For Controllable Image Synthesis
2019 IEEE/CVF CONFERENCE ON COMPUTER VISION AND PATTERN RECOGNITION (CVPR 2019)(2019)
摘要
Flow-based generative models show great potential in image synthesis due to its reversible pipeline and exact loglikelihood target, yet it suffers from weak abilityfor conditional image synthesis, especially for multi-label or unaware conditions. This is because the potential distribution of image conditions is hard to measure precisely from its latent variable z. In this paper based on modeling a joint probabilisticdensity of an image and its conditions, we propose a novel flow-based generative model named conditional adversarialgenerative flow (CAGlow). Instead of disentangling attributesfrom latent space, we blaze a new trailfor learningan encoder to estimate the mapping from condition space to latent space in an adversarialmanner. Given a specific condition c, CAGlow can encode it to a sampled z, and then enable robust conditional image synthesis in complex situations like combining person identity with multiple attributes. The proposed CAGlow can be implemented in both supervised and unsupervised manners, thus can synthesize images with conditional information like categories, attributes, and even some unknown properties. Extensive experiments show that CAGlow ensures the independence of different conditions and outperforms regularGlow to a significant extent.
更多查看译文
关键词
Image and Video Synthesis,Face,Gesture,and Body Pose,Representation Learning
AI 理解论文
溯源树
样例
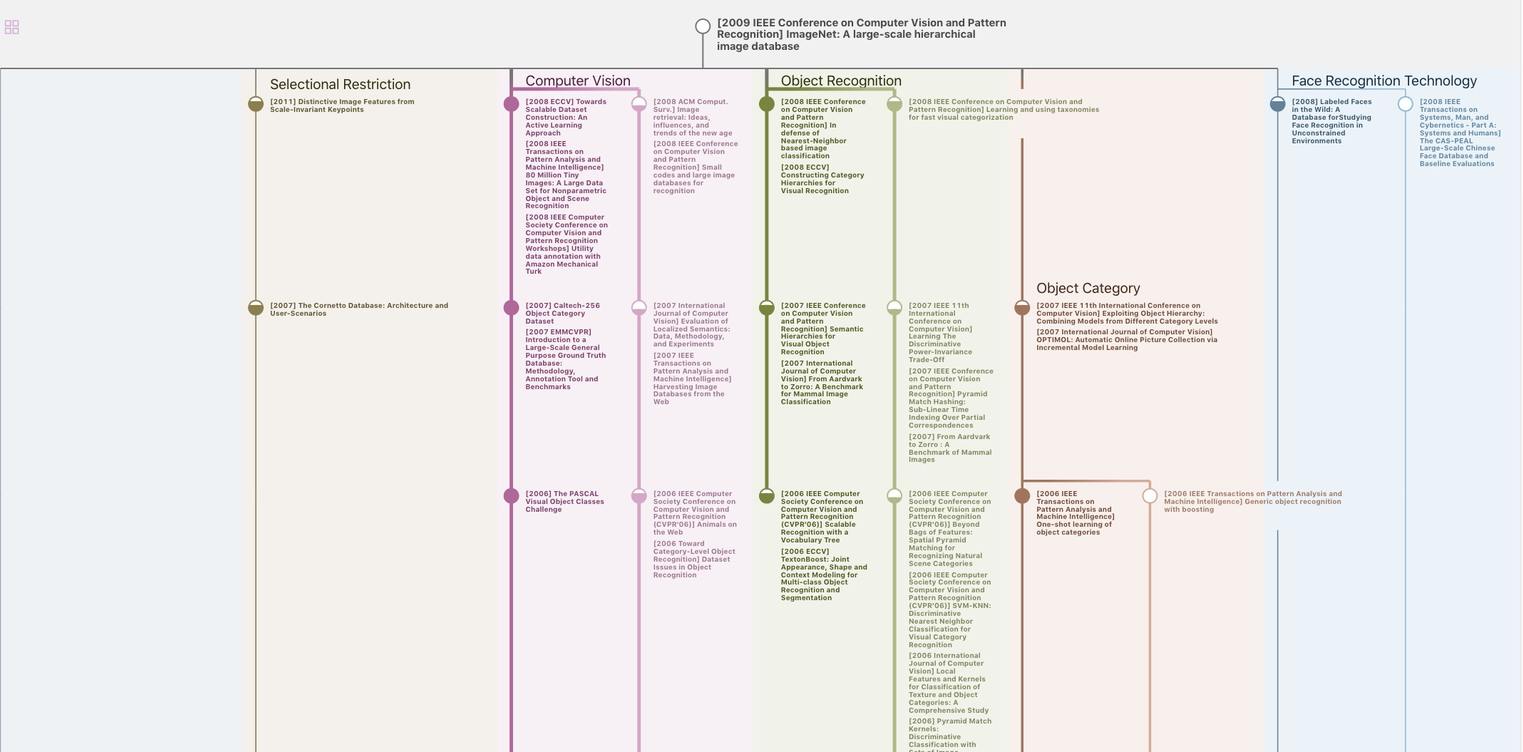
生成溯源树,研究论文发展脉络
Chat Paper
正在生成论文摘要