Unsupervised Training of Neural Mask-Based Beamforming.
INTERSPEECH(2019)
摘要
We present an unsupervised training approach for a neural network-based mask estimator in an acoustic beamforming application. The network is trained to maximize a likelihood criterion derived from a spatial mixture model of the observations. It is trained from scratch without requiring any parallel data consisting of degraded input and clean training targets. Thus, training can be carried out on real recordings of noisy speech rather than simulated ones. In contrast to previous work on unsupervised training of neural mask estimators, our approach avoids the need for a possibly pre-trained teacher model entirely. We demonstrate the effectiveness of our approach by speech recognition experiments on two different datasets: one mainly deteriorated by noise (CHiME 4) and one by reverberation (REVERB). The results show that the performance of the proposed system is on par with a supervised system using oracle target masks for training and with a system trained using a model-based teacher.
更多查看译文
关键词
deep learning, multi-channel, unsupervised learning, robust speech recognition, beamforming
AI 理解论文
溯源树
样例
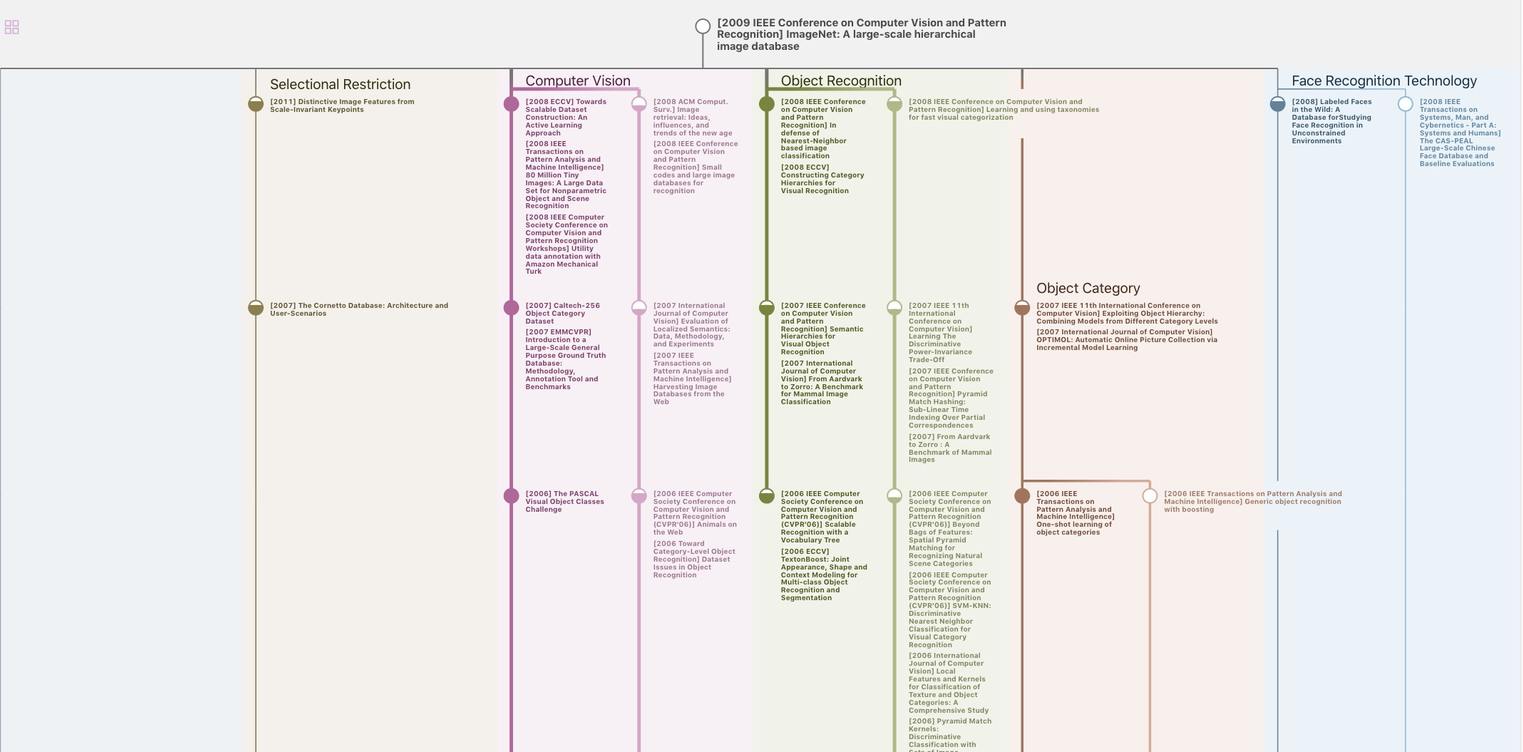
生成溯源树,研究论文发展脉络
Chat Paper
正在生成论文摘要