Monocular 3d Human Pose Estimation By Generation And Ordinal Ranking
2019 IEEE/CVF INTERNATIONAL CONFERENCE ON COMPUTER VISION (ICCV 2019)(2019)
摘要
Monocular 3D human-pose estimation from static images is a challenging problem, due to the curse of dimensionality and the ill-posed nature of lifting 2D-to-3D. In this paper, we propose a Deep Conditional Variational Autoencoder based model that synthesizes diverse anatomically plausible 3D-pose samples conditioned on the estimated 2D-pose. We show that CVAE-based 3D-pose sample set is consistent with the 2D-pose and helps tackling the inherent ambiguity in 2D-to-3D lifting. We propose two strategies for obtaining the final 3D pose- (a) depth-ordering/ordinal relations to score and weight-average the candidate 3D-poses, referred to as OrdinalScore, and (b) with supervision from an Oracle. We report close to state-of-the-art results on two benchmark datasets using OrdinalScore, and state-of-the-art results using the Oracle. We also show that our pipeline yields competitive results without paired image-to-3D annotations.
更多查看译文
关键词
monocular 3D human pose estimation,image-to-3D annotations,deep conditional variational autoencoder based model,ordinal ranking,static images,3D-pose samples,CVAE-based 3D-pose sample set,2D-to-3D lifting,depth-ordering/ordinal relations,OrdinalScore
AI 理解论文
溯源树
样例
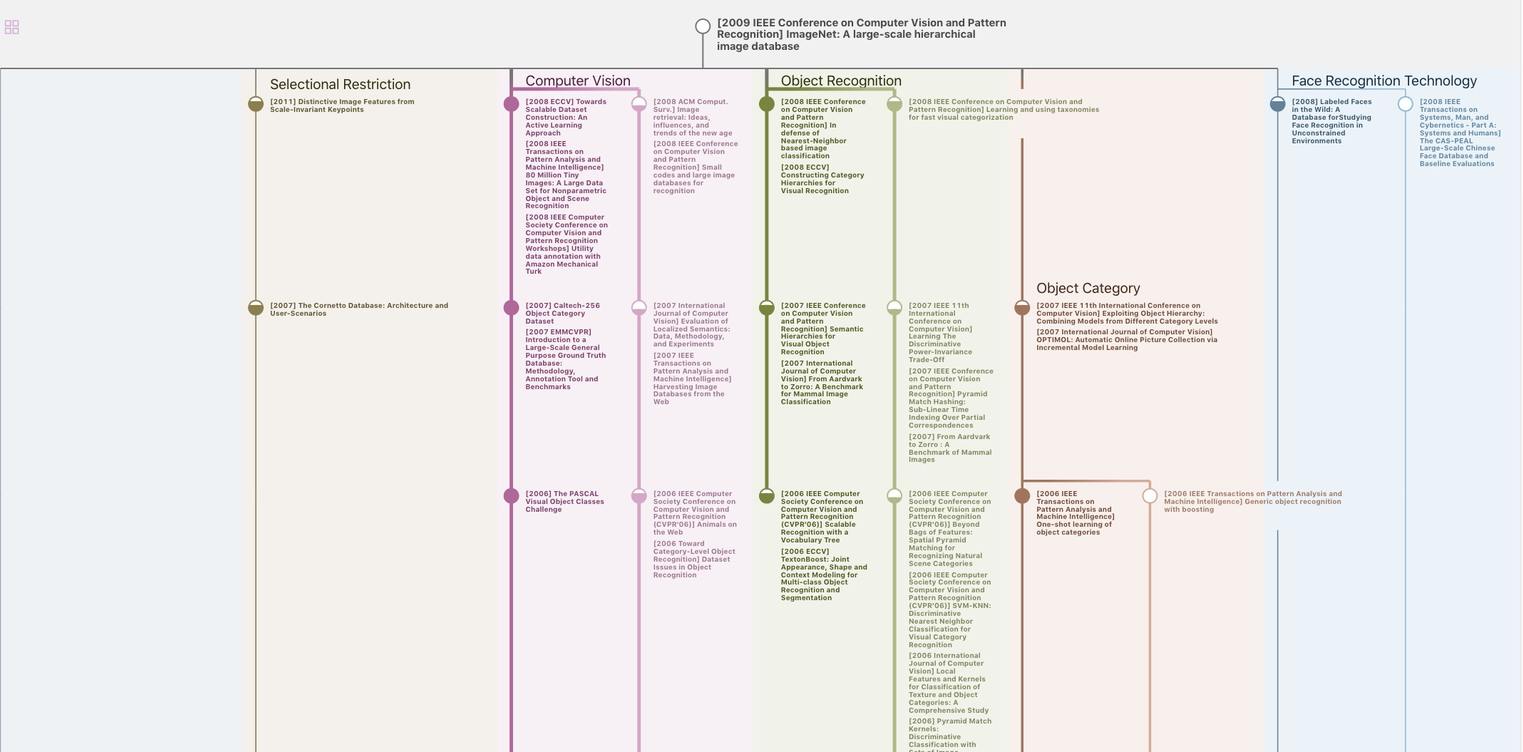
生成溯源树,研究论文发展脉络
Chat Paper
正在生成论文摘要