SGSF: a small groups based serial fusion method
Proceedings of the 18th International Conference on Information Processing in Sensor Networks(2019)
摘要
With the rapid development of Artificial Intelligence (AI) in the Internet of things (IoT), information fusion becomes one of the key technology. Serial fusion plays an important role in information fusion, which concatenates the features obtained from different sources to get a super-feature with higher discrimination. However, the performance of the serial fusion is usually affected by the poor quality of the indistinguishable real-world datasets, especially the problem of noise and imbalance. The existing noise data removal methods may destroy the data distribution, and the existing over-sampling methods may create noise samples. They may affect the result of feature reduction and further reduce the performance of the classification. To address these big practical challenges, a novel Small-Groups-based Serial Fusion method (SGSF) is proposed. SGSF consists of two main parts: a Small-Groups-based Noise Removal method (SGNR) and a small-groups-based Positive Region Oriented Oversampling method (PROO). Both of these two methods are based on the Neighborhood Rough Set (NRS) theory. SGNR identifies and removes low-density noise samples whilst keeps the high-density outlier samples, targeting to enlarge the limited NRS positive region and preserve the data distribution. PROO generates new samples towards the NRS positive region so that it can balance the dataset and increase the number of samples with more explicit features. Intensive experiments are carried out to verify the performance of SGSF based on 3 publicly available datasets and 6 self-collected medical datasets. The results demonstrate that SGSF increases the classification accuracy from existing 82.2% to 94.3%.
更多查看译文
关键词
exclave groups, neighborhood rough set small group, noise removal, positive region oriented oversampling, serial fusion
AI 理解论文
溯源树
样例
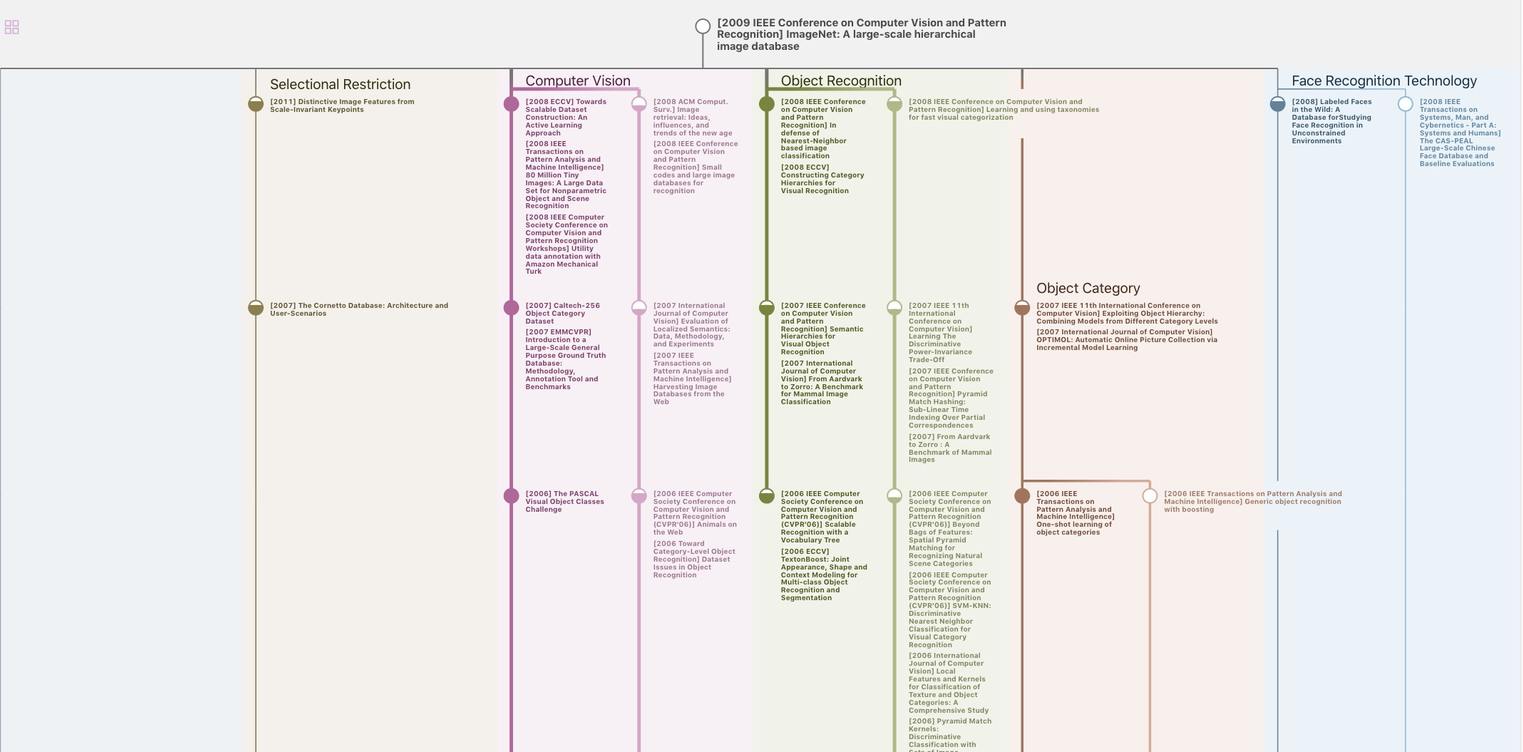
生成溯源树,研究论文发展脉络
Chat Paper
正在生成论文摘要