Double Deep Q-Network For Trajectory Generation Of A Commercial 7dof Redundant Manipulator
2019 THIRD IEEE INTERNATIONAL CONFERENCE ON ROBOTIC COMPUTING (IRC 2019)(2019)
摘要
Recent studies to solve industrial automation applications where a new and unfamiliar environment is presented have seen a shift to solutions using Reinforcement Learning policies. Building upon the recent success of Deep Q-Networks (DQNs), we present a comparison between DQNs and Double Deep Q-Networks (DDQNs) for the training of a commercial seven joint redundant manipulator in a real-time trajectory generation task. Experimental results demonstrate that the DDQN approach is more stable then DQN. Moreover, we show that these policies can be directly applied to the official visualizer provided by the robot manufacturer and to the real robot without any further training.
更多查看译文
关键词
Deep Learning,Reinforcement Learning,Industrial Manipulators
AI 理解论文
溯源树
样例
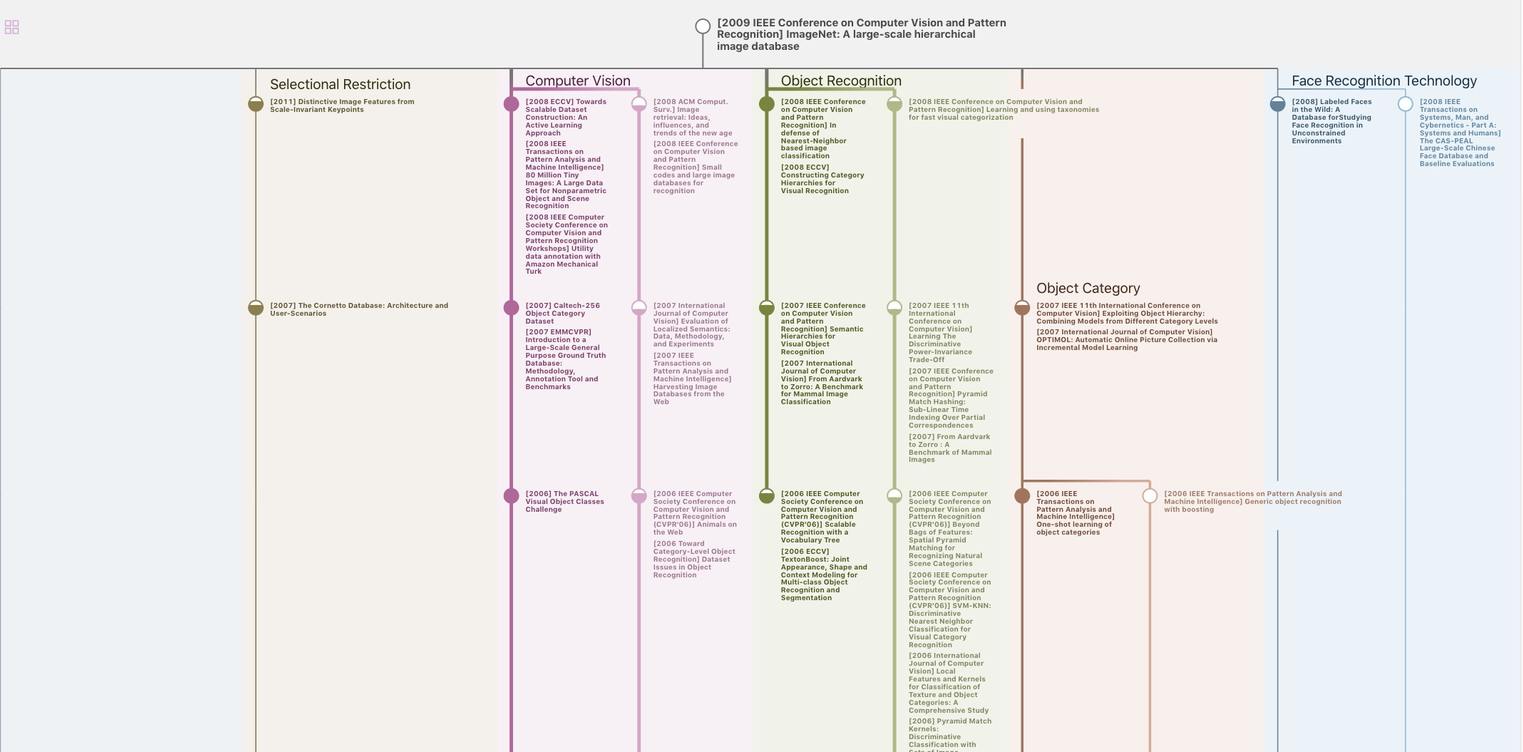
生成溯源树,研究论文发展脉络
Chat Paper
正在生成论文摘要