A Hybrid Indoor Localization System Running Ensemble Machine Learning
2018 IEEE INT CONF ON PARALLEL & DISTRIBUTED PROCESSING WITH APPLICATIONS, UBIQUITOUS COMPUTING & COMMUNICATIONS, BIG DATA & CLOUD COMPUTING, SOCIAL COMPUTING & NETWORKING, SUSTAINABLE COMPUTING & COMMUNICATIONS(2018)
摘要
The need for localization in various fields of applications and the lack of efficiency in using GPS indoor leads to the development of Indoor Localization Systems. The recent rapid growth of mobile users and Wi-Fi infrastructure of modern buildings enables different methodologies to build high performance indoor localization system with minimum investment. This paper presents a novel model for indoor localization system on Android mobile devices with built-in application running ensemble learning method and artificial neural network. The system performance is enhanced with the implementation of background filters using built-in sensors. Notably, the proposed model is designed to gradually converge to location the longer the runtime. It eventually produces the correct rate of 95 percent for small-room localization with error radius of approximately 0.5 to 1 meter and the convergence time of 10 seconds at best. The developed model can run offline and optimized for embedded systems and Android devices based on pre-built models of Wi-Fi fingerprints.
更多查看译文
关键词
Big Data, Wi-Fi, random forest, multilayer perceptron, artificial neural network, ensemble learning, model, pedometer, indoor localization system, Android, trilateration, complementary filter, sample mean, fingerprinting, database
AI 理解论文
溯源树
样例
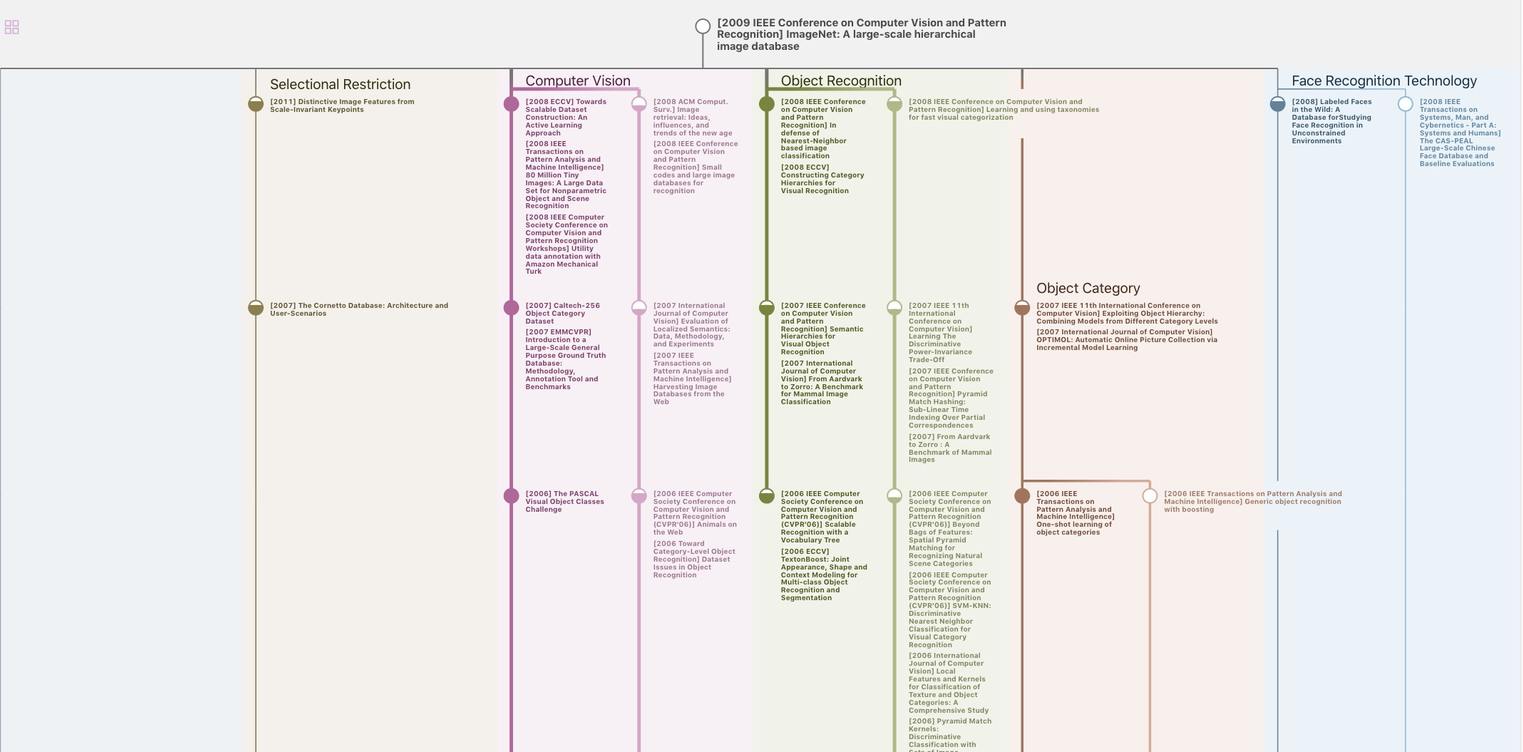
生成溯源树,研究论文发展脉络
Chat Paper
正在生成论文摘要