Deep Learning in Asset Pricing
MANAGEMENT SCIENCE(2024)
摘要
We use deep neural networks to estimate an asset pricing model for individual stock returns that takes advantage of the vast amount of conditioning information, keeps a fully flexible form, and accounts for time variation. The key innovations are to use the fundamental no-arbitrage condition as criterion function to construct the most informative test assets with an adversarial approach and to extract the states of the economy from many macroeconomic time series. Our asset pricing model outperforms out-of-sample all benchmark approaches in terms of Sharpe ratio, explained variation, and pricing errors and identifies the key factors that drive asset prices.
更多查看译文
关键词
conditional asset pricing model,no arbitrage,stock returns,nonlinear factor model,cross-section of expected returns,machine learning,deep learning,big data,hidden states,GMM
AI 理解论文
溯源树
样例
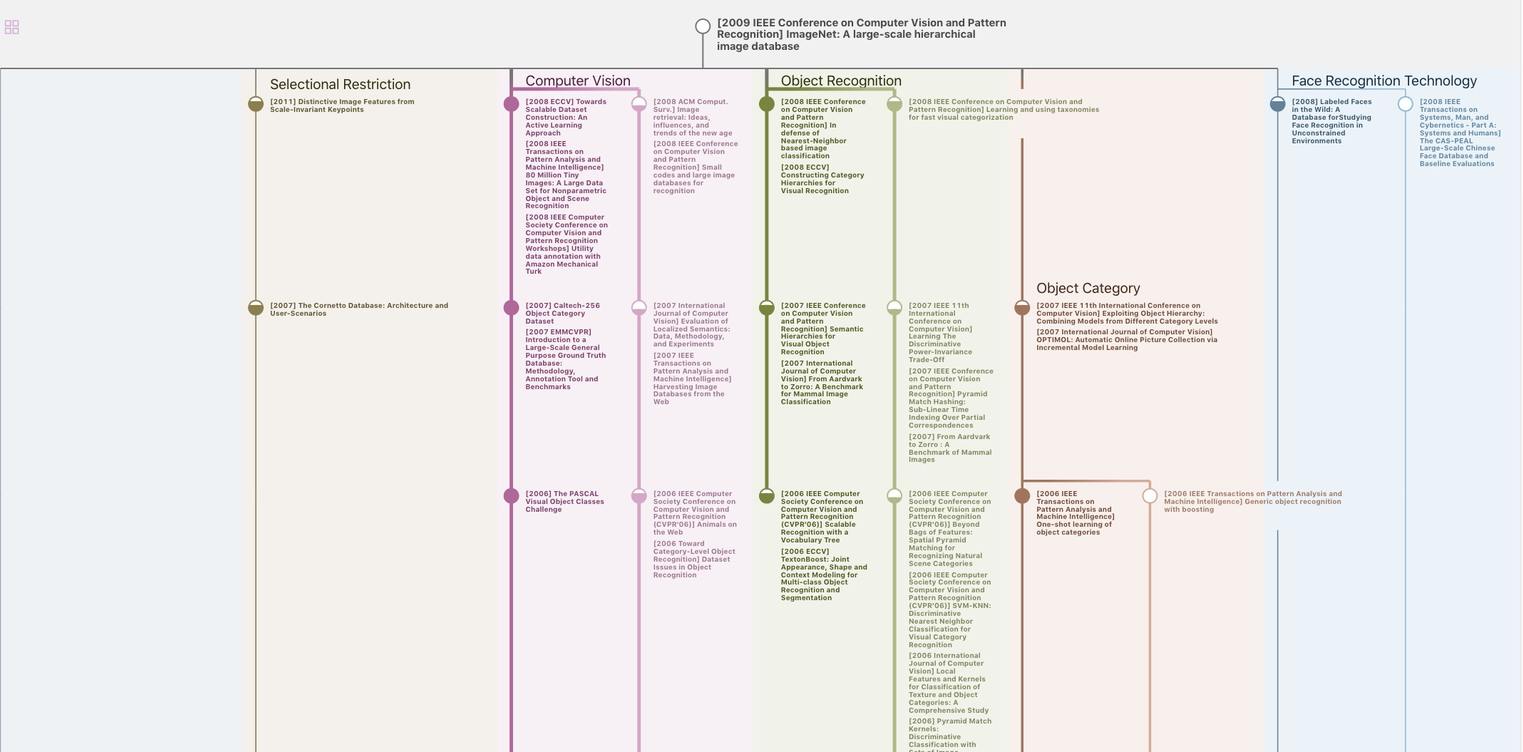
生成溯源树,研究论文发展脉络
Chat Paper
正在生成论文摘要