From Fingerprint to Footprint: Cold-start Location Recommendation by Learning User Interest from App Data
Proceedings of the ACM on Interactive, Mobile, Wearable and Ubiquitous Technologies(2019)
摘要
With increasing diversity of user interest and preference, personalized location recommendation is essential and beneficial to our daily life. To achieve this, the most critical challenge is the cold-start recommendation problem, for we cannot learn preference from cold-start users without any historical records. In this paper, we demonstrate that it is feasible to make personalized location recommendation by learning user interest and location features from app usage data. By proposing a novel generative model to transfer user interests from app usage behavior to location preference, we achieve personalized location recommendation via learning the interest's correlation between locations and apps. Based on two real-world datasets, we evaluate our method's performance with a variety of scenarios and parameters. The results demonstrate that our method outperforms the state-of-the-art solutions in solving cold-start problem, i.e., when there are 60% cold-start users, we can still achieve a 77.0% hitrate in recommending the top five locations, which is at least 9.6% higher than the baselines. Our study is the first step forward for transferring user interests learning from online fingerprints to offline footprints, which paves the way for better personalized location recommendation services.
更多查看译文
关键词
Location recommendation, cold-start problem, generative model
AI 理解论文
溯源树
样例
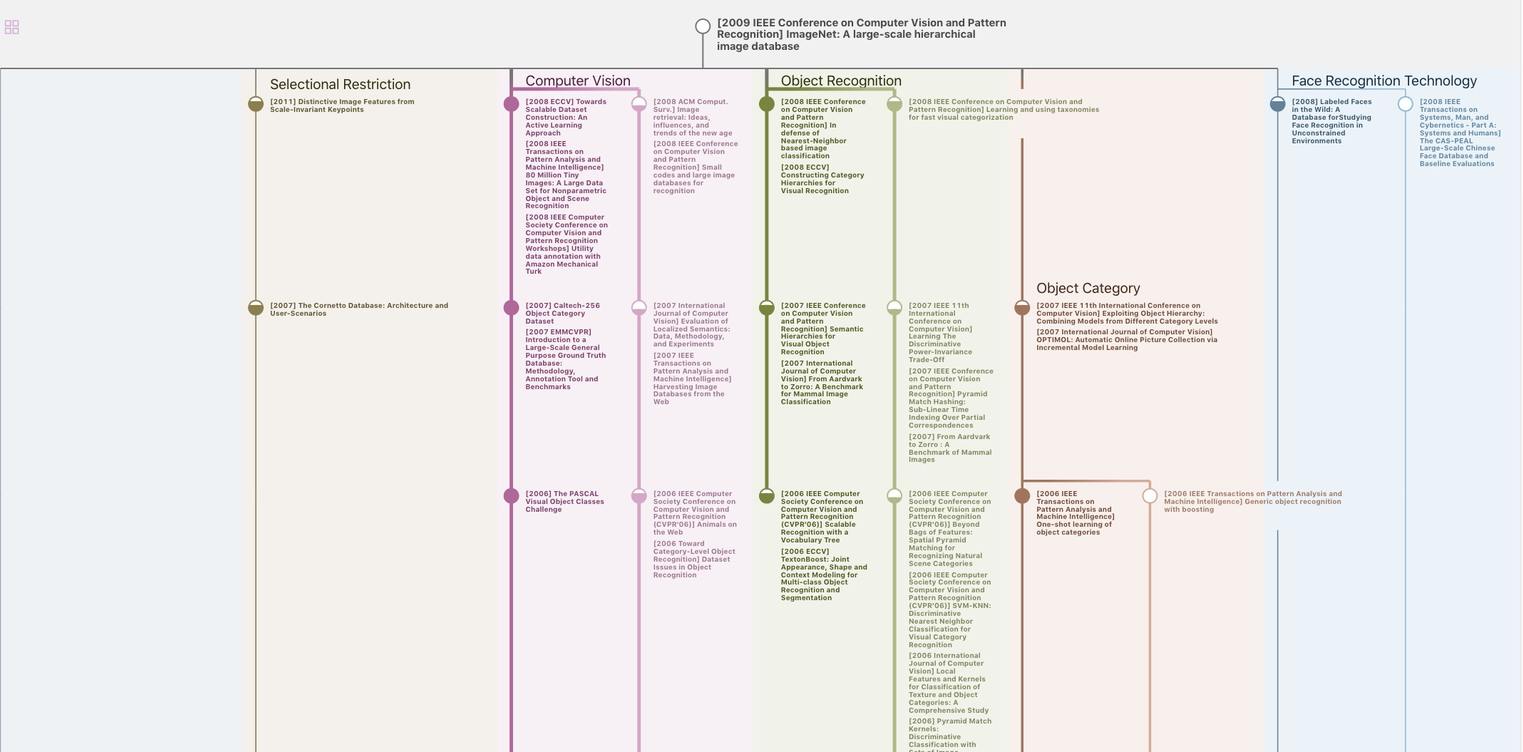
生成溯源树,研究论文发展脉络
Chat Paper
正在生成论文摘要