Improving image classifiers for small datasets by learning rate adaptations
2019 16th International Conference on Machine Vision Applications (MVA)(2019)
摘要
Our paper introduces an efficient combination of established techniques to improve classifier performance, in terms of accuracy and training time. We achieve two-fold to ten-fold speedup in nearing state of the art accuracy, over different model architectures, by dynamically tuning the learning rate. We find it especially beneficial in the case of a small dataset, where reliability of machine reasoning is lower. We validate our approach by comparing our method versus vanilla training on CIFAR-10. We also demonstrate its practical viability by implementing on an unbalanced corpus of diagnostic images.
更多查看译文
关键词
training time,nearing state,learning rate,datasets,machine reasoning,vanilla training,diagnostic images,rate adaptations,efficient combination,classifier performance,image classifiers,model architectures,two-fold to ten-fold speedup,unbalanced corpus
AI 理解论文
溯源树
样例
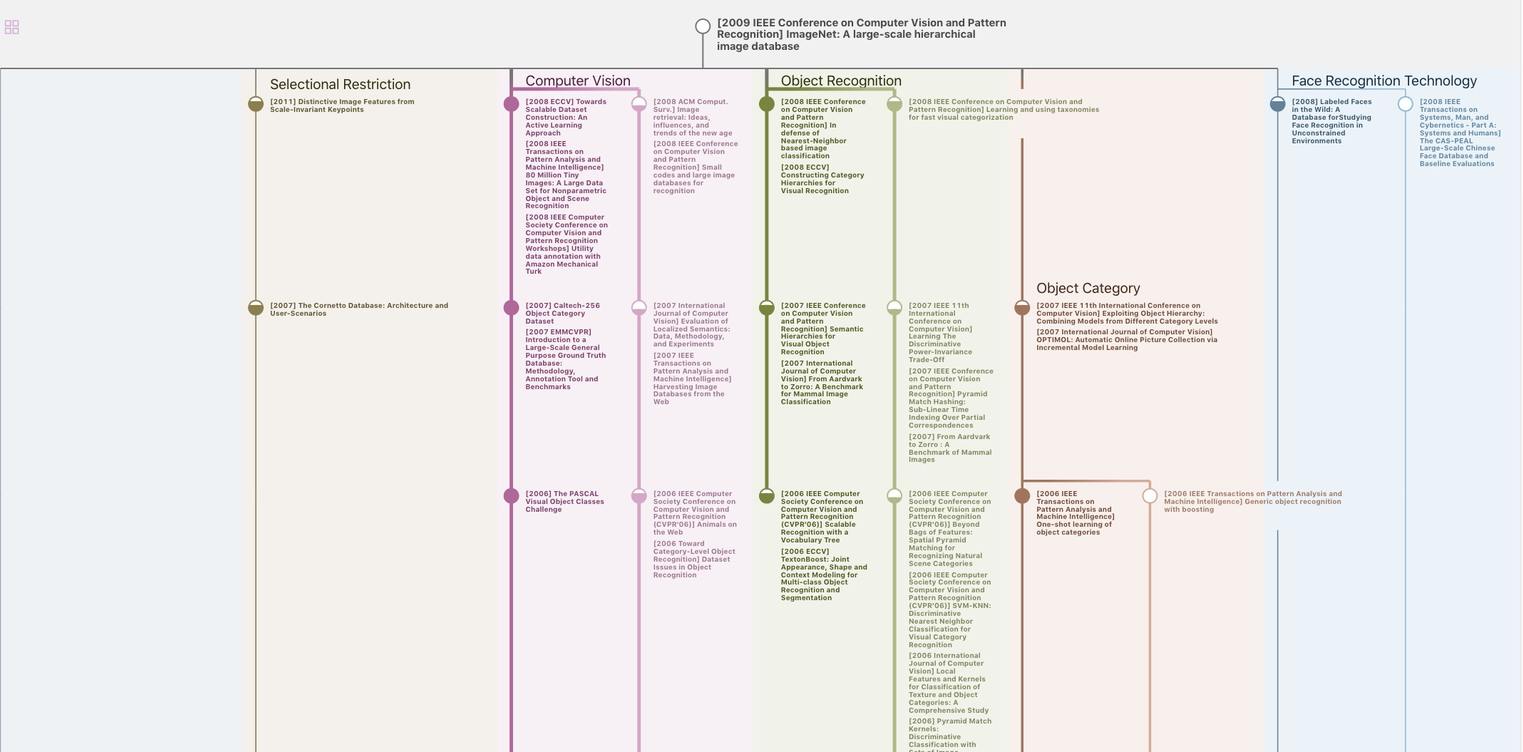
生成溯源树,研究论文发展脉络
Chat Paper
正在生成论文摘要